Open Access
ARTICLE
Ensemble Classifier Technique to Predict Gestational Diabetes Mellitus (GDM)
SRC, SASTRA Deemed to be University, Kumbakonam, Tamil Nadu, 612001, India
* Corresponding Author: A. Sumathi. Email:
Computer Systems Science and Engineering 2022, 40(1), 313-325. https://doi.org/10.32604/csse.2022.017484
Received 31 January 2021; Accepted 23 April 2021; Issue published 26 August 2021
Abstract
Gestational Diabetes Mellitus (GDM) is an illness that represents a certain degree of glucose intolerance with onset or first recognition during pregnancy. In the past few decades, numerous investigations were conducted upon early identification of GDM. Machine Learning (ML) methods are found to be efficient prediction techniques with significant advantage over statistical models. In this view, the current research paper presents an ensemble of ML-based GDM prediction and classification models. The presented model involves three steps such as preprocessing, classification, and ensemble voting process. At first, the input medical data is preprocessed in four levels namely, format conversion, class labeling, replacement of missing values, and normalization. Besides, four ML models such as Logistic Regression (LR), k-Nearest Neighbor (KNN), Support Vector Machine (SVM), and Random Forest (RF) are used for classification. In addition to the above, RF, LR, KNN and SVM classifiers are integrated to perform the final classification in which a voting classifier is also used. In order to investigate the proficiency of the proposed model, the authors conducted extensive set of simulations and the results were examined under distinct aspects. Particularly, the ensemble model has outperformed the classical ML models with a precision of 94%, recall of 94%, accuracy of 94.24%, and F-score of 94%.Keywords
Cite This Article
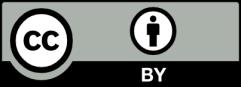
This work is licensed under a Creative Commons Attribution 4.0 International License , which permits unrestricted use, distribution, and reproduction in any medium, provided the original work is properly cited.