Open Access
ARTICLE
Semisupervised Encrypted Traffic Identification Based on Auxiliary Classification Generative Adversarial Network
1 State Grid Jiangsu Electric Power Co., Ltd. Information and Telecommunication Branch, NanJing, 210003, China
2 Institute of Information and Communication, Global Energy Interconnection Research Institute, State Grid Key Laboratory of Information and Network Security, Nanjing, 210003, China
3 Engineering Research Center of Post Big Data Technology and Application of Jiangsu Province, Research and Development Center of Post Industry Technology of the State Posts Bureau (Internet of Things Technology), Broadband Wireless Communication Technology Engineering Research Center of the Ministry of Education, Nanjing University of Posts and Telecommunications, Nanjing, 210003, China
4 Department of Electrical Engineering and Computer Science, Cleveland State University, Cleveland, 44115, USA
* Corresponding Author: Jiaming Mao. Email:
Computer Systems Science and Engineering 2021, 39(3), 373-390. https://doi.org/10.32604/csse.2021.018086
Received 24 February 2021; Accepted 21 April 2021; Issue published 12 August 2021
Abstract
The rapidly increasing popularity of mobile devices has changed the methods with which people access various network services and increased network traffic markedly. Over the past few decades, network traffic identification has been a research hotspot in the field of network management and security monitoring. However, as more network services use encryption technology, network traffic identification faces many challenges. Although classic machine learning methods can solve many problems that cannot be solved by port- and payload-based methods, manually extract features that are frequently updated is time-consuming and labor-intensive. Deep learning has good automatic feature learning capabilities and is an ideal method for network traffic identification, particularly encrypted traffic identification; Existing recognition methods based on deep learning primarily use supervised learning methods and rely on many labeled samples. However, in real scenarios, labeled samples are often difficult to obtain. This paper adjusts the structure of the auxiliary classification generation adversarial network (ACGAN) so that it can use unlabeled samples for training, and use the wasserstein distance instead of the original cross entropy as the loss function to achieve semisupervised learning. Experimental results show that the identification accuracy of ISCX and USTC data sets using the proposed method yields markedly better performance when the number of labeled samples is small compared to that of convolutional neural network (CNN) based classifier.Keywords
Cite This Article
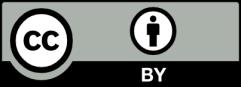
This work is licensed under a Creative Commons Attribution 4.0 International License , which permits unrestricted use, distribution, and reproduction in any medium, provided the original work is properly cited.