Open Access
ARTICLE
Cyclic Autoencoder for Multimodal Data Alignment Using Custom Datasets
1 College of Information Engineering, Shanghai Maritime University, Shanghai, 200135, China
2 Division of Management and Education, University of Pittsburgh, Bradford, 16701, USA
* Corresponding Author: Jin Liu. Email:
Computer Systems Science and Engineering 2021, 39(1), 37-54. https://doi.org/10.32604/csse.2021.017230
Received 21 January 2021; Accepted 24 February 2021; Issue published 10 June 2021
Abstract
The subtitle recognition under multimodal data fusion in this paper aims to recognize text lines from image and audio data. Most existing multimodal fusion methods tend to be associated with pre-fusion as well as post-fusion, which is not reasonable and difficult to interpret. We believe that fusing images and audio before the decision layer, i.e., intermediate fusion, to take advantage of the complementary multimodal data, will benefit text line recognition. To this end, we propose: (i) a novel cyclic autoencoder based on convolutional neural network. The feature dimensions of the two modal data are aligned under the premise of stabilizing the compressed image features, thus the high-dimensional features of different modal data are fused at the shallow level of the model. (ii) A residual attention mechanism that helps us improve the performance of the recognition. Regions of interest in the image are enhanced and regions of disinterest are weakened, thus we can extract the features of the text regions without further increasing the depth of the model (iii) a fully convolutional network for video subtitle recognition. We choose DenseNet-121 as the backbone network for feature extraction, which effectively enabling the recognition of video subtitles in complex backgrounds. The experiments are performed on our custom datasets, and the automatic and manual evaluation results show that our method reaches the state-of-the-art.Keywords
Cite This Article
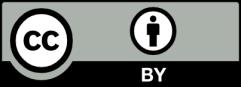