Open Access
ARTICLE
Mixed Attention Densely Residual Network for Single Image Super-Resolution
1 School of Information and Communication Engineering, Hainan University, Haikou, 570228, China
2 State Key Laboratory of Marine Resource Utilization in the South China Sea, Hainan University, Haikou, 570228, China
3 Research Center for Healthcare Data Science, Zhejiang Lab, Hangzhou, 311121, China
4 School of Computer Science and Cyberspace Security, Hainan University, Haikou, 570228, China
5 Graduate School of Information Science and Engineering, Ritsumeikan University, 5258577, Japan
6 College of Computer Science and Technology, Zhejiang University, Hangzhou, 311100, China
* Corresponding Author: Jingbing Li. Email:
Computer Systems Science and Engineering 2021, 39(1), 133-146. https://doi.org/10.32604/csse.2021.016633
Received 01 January 2021; Accepted 04 March 2021; Issue published 10 June 2021
Abstract
Recent applications of convolutional neural networks (CNNs) in single image super-resolution (SISR) have achieved unprecedented performance. However, existing CNN-based SISR network structure design consider mostly only channel or spatial information, and cannot make full use of both channel and spatial information to improve SISR performance further. The present work addresses this problem by proposing a mixed attention densely residual network architecture that can make full and simultaneous use of both channel and spatial information. Specifically, we propose a residual in dense network structure composed of dense connections between multiple dense residual groups to form a very deep network. This structure allows each dense residual group to apply a local residual skip connection and enables the cascading of multiple residual blocks to reuse previous features. A mixed attention module is inserted into each dense residual group, to enable the algorithm to fuse channel attention with laplacian spatial attention effectively, and thereby more adaptively focus on valuable feature learning. The qualitative and quantitative results of extensive experiments have demonstrate that the proposed method has a comparable performance with other state-of-the-art methods.Keywords
Cite This Article
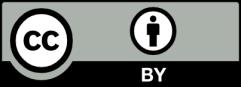