Open Access
ARTICLE
An Ensemble Approach to Identify Firearm Listing on Tor Hidden-Services
1 Department of Computer Science, College of Computers and Information Technology, Taif University, P. O. Box 11099, Taif 21944, Saudi Arabia
2 Department of Information Technology, Babasaheb Bhimrao Ambedkar University, Lucknow, 226025, Uttar Pradesh, India
3 Department of Information Technology, College of Computers and Information Technology, Taif University, P. O. Box 11099, Taif 21944, Saudi Arabia
4 Department of Computer Application, Integral University, Lucknow, 226026, Uttar Pradesh, India
* Corresponding Author: Raees Ahmad Khan. Email:
Computer Systems Science and Engineering 2021, 38(2), 141-149. https://doi.org/10.32604/csse.2021.017039
Received 18 January 2021; Accepted 19 February 2021; Issue published 23 April 2021
Abstract
The ubiquitous nature of the internet has made it easier for criminals to carry out illegal activities online. The sale of illegal firearms and weaponry on dark web cryptomarkets is one such example of it. To aid the law enforcement agencies in curbing the illicit trade of firearms on cryptomarkets, this paper has proposed an automated technique employing ensemble machine learning models to detect the firearms listings on cryptomarkets. In this work, we have used part-of-speech (PoS) tagged features in conjunction with n-gram models to construct the feature set for the ensemble model. We studied the effectiveness of the proposed features in the performance of the classification model and the relative change in the dimensionality of the feature set. The experiments and evaluations are performed on the data belonging to the three popular cryptomarkets on the Tor dark web from a publicly available dataset. The prediction of the classification model can be utilized to identify the key vendors in the ecosystem of the illegal trade of firearms. This information can then be used by law enforcement agencies to bust firearm trafficking on the dark web.Keywords
Cite This Article
H. Alyami, M. Faizan, W. Alosaimi, A. Alharbi, A. Kumar Pandey et al., "An ensemble approach to identify firearm listing on tor hidden-services," Computer Systems Science and Engineering, vol. 38, no.2, pp. 141–149, 2021. https://doi.org/10.32604/csse.2021.017039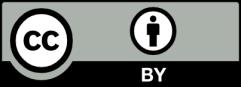