Open Access
ARTICLE
Deep Learning for Object Detection: A Survey
1 Science and Technology Industry Department, Nanjing University of Information Science & Technology, 210044, China
2 School of Computer & Software, Jiangsu Engineering Center of Network Monitoring, Nanjing University of Information Science & Technology, 210044, China
3 Science and Technology Industry Department, Nanjing University of Information Science & Technology, 210044, China
4 Department Computer Science, King Saud University, Riyadh, KSA
* Corresponding Author: Tingjuan Zhang. Email:
Computer Systems Science and Engineering 2021, 38(2), 165-182. https://doi.org/10.32604/csse.2021.017016
Received 18 January 2021; Accepted 19 February 2021; Issue published 23 April 2021
Abstract
Object detection is one of the most important and challenging branches of computer vision, which has been widely applied in people s life, such as monitoring security, autonomous driving and so on, with the purpose of locating instances of semantic objects of a certain class. With the rapid development of deep learning algorithms for detection tasks, the performance of object detectors has been greatly improved. In order to understand the main development status of target detection, a comprehensive literature review of target detection and an overall discussion of the works closely related to it are presented in this paper. This paper various object detection methods, including one-stage and two-stage detectors, are systematically summarized, and the datasets and evaluation criteria used in object detection are introduced. In addition, the development of object detection technology is reviewed. Finally, based on the understanding of the current development of target detection, we discuss the main research directions in the future.Keywords
Cite This Article
Citations
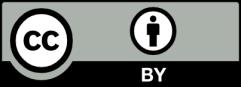