Open Access
ARTICLE
Leveraging Graph Cut’s Energy Function for Context Aware Facial Recognition in Indoor Environments
1 Pan-Atlantic University, Lagos, Nigeria
2 Department of Electrical Engineering, Tshwane University of Technology, Pretoria, South Africa
3 Faculty of Engineering, the Built Environment and Technology Nelson Mandela University, South Africa
* Corresponding Author: Shengzhi Du. Email:
Computer Systems Science and Engineering 2021, 38(2), 229-238. https://doi.org/10.32604/csse.2021.015372
Received 17 November 2020; Accepted 14 February 2021; Issue published 23 April 2021
Abstract
Context-aware facial recognition regards the recognition of faces in association with their respective environments. This concept is useful for the domestic robot which interacts with humans when performing specific functions in indoor environments. Deep learning models have been relevant in solving facial and place recognition challenges; however, they require the procurement of training images for optimal performance. Pre-trained models have also been offered to reduce training time significantly. Regardless, for classification tasks, custom data must be acquired to ensure that learning models are developed from other pre-trained models. This paper proposes a place recognition model that is inspired by the graph cut energy function, which is specifically designed for image segmentation. Common objects in the considered environment are identified and thereafter they are passed over to a graph cut inspired model for indoor environment classification. Additionally, faces in the considered environment are extracted and recognised. Finally, the developed model can recognise a face together with its environment. The strength of the proposed model lies in its ability to classify indoor environments without the usual training process(es). This approach differs from what is obtained in traditional deep learning models. The classification capability of the developed model was compared to state-of-the-art models and exhibited promising outcomes.Keywords
Cite This Article
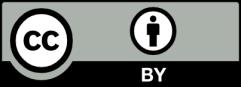
This work is licensed under a Creative Commons Attribution 4.0 International License , which permits unrestricted use, distribution, and reproduction in any medium, provided the original work is properly cited.