Open Access
ARTICLE
Generalized Normalized Euclidean Distance Based Fuzzy Soft Set Similarity for Data Classification
1 Faculty of Computer and Information Technology, Universiti Tun Hussein Onn Malaysia, Batu Pahat, Malaysia
2 Department of Information Technology, Politeknik Negeri Padang, Padang, Indonesia
3 Department of Information System, Universitas Ahmad Dahlan, Yogyakarta, Indonesia
4 Department of Statistics, Universitas Negeri Makassar, Makassar, Indonesia
* Corresponding Author: Rahmat Hidayat. Email:
Computer Systems Science and Engineering 2021, 38(1), 119-130. https://doi.org/10.32604/csse.2021.015628
Received 30 November 2020; Accepted 17 February 2021; Issue published 01 April 2021
Abstract
Classification is one of the data mining processes used to predict predetermined target classes with data learning accurately. This study discusses data classification using a fuzzy soft set method to predict target classes accurately. This study aims to form a data classification algorithm using the fuzzy soft set method. In this study, the fuzzy soft set was calculated based on the normalized Hamming distance. Each parameter in this method is mapped to a power set from a subset of the fuzzy set using a fuzzy approximation function. In the classification step, a generalized normalized Euclidean distance is used to determine the similarity between two sets of fuzzy soft sets. The experiments used the University of California (UCI) Machine Learning dataset to assess the accuracy of the proposed data classification method. The dataset samples were divided into training (75% of samples) and test (25% of samples) sets. Experiments were performed in MATLAB R2010a software. The experiments showed that: (1) The fastest sequence is matching function, distance measure, similarity, normalized Euclidean distance, (2) the proposed approach can improve accuracy and recall by up to 10.3436% and 6.9723%, respectively, compared with baseline techniques. Hence, the fuzzy soft set method is appropriate for classifying data.
Keywords
Cite This Article
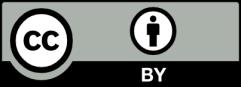
This work is licensed under a Creative Commons Attribution 4.0 International License , which permits unrestricted use, distribution, and reproduction in any medium, provided the original work is properly cited.