Open Access
ARTICLE
A New Generalized Weibull Model: Classical and Bayesian Estimation
1 School of Statistics, Shanxi University of Finance and Economics, Taiyuan, China
2 Department of Statistics, Yazd University, Yazd, Iran
3 Department of Statistics, Faculty of Sciences, Vali-e-Asr University of Rafsanjan, Rafsanjan, Iran
* Corresponding Author: Zubair Ahmad. Email:
Computer Systems Science and Engineering 2021, 38(1), 79-92. https://doi.org/10.32604/csse.2021.015146
Received 08 November 2020; Accepted 27 December 2020; Issue published 01 April 2021
Abstract
Statistical distributions play a prominent role in applied sciences, particularly in biomedical sciences. The medical data sets are generally skewed to the right, and skewed distributions can be used quite effectively to model such kind of data sets. In the present study, therefore, we propose a new family of distributions suitable for modeling right-skewed medical data sets. The proposed family may be called a new generalized-X family. A special sub-model of the proposed family called a new generalized-Weibull distribution is discussed in detail. The maximum likelihood estimators of the model parameters are obtained. A brief Monte Carlo simulation study is conducted to evaluate the performance of these estimators. Finally, the proposed model is applied to the remission times of the stomach cancer patient’s data. The comparison of the goodness of fit results of the proposed model is made with the other competing models such as Weibull, Kumaraswamy Weibull, and exponentiated Weibull distributions. Certain analytical measures such as Akaike information criterion, Bayesian information criterion, Anderson Darling statistic, and Kolmogorov–Smirnov test statistic are considered to show which distribution provides the best fit to data. Based on these measures, it is showed that the proposed distribution is a reasonable candidate for modeling data in medical sciences and other related fields.Keywords
Cite This Article
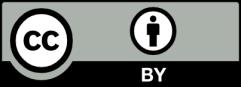
This work is licensed under a Creative Commons Attribution 4.0 International License , which permits unrestricted use, distribution, and reproduction in any medium, provided the original work is properly cited.