Open Access
ARTICLE
Human-Animal Affective Robot Touch Classification Using Deep Neural Network
1 AL-Iraqia University, College of Education, Computer Department, Baghdad, Iraq
2 Community College of Abqaiq, King Faisal University, Al-Ahsa, Saudi Arabia
3 Deanship of E-learning and Distance Education, King Faisal University, Al-Ahsa, Saudi Arabia
4 UMM Al-Qura University, College of Computing, Makkah, Saudi Arabia
5 Department of Computer Sciences and Information Technology, Albaha University, Al Baha, Saudi Arabia
6 College of Computer Science and Information Technology, King Faisal University, Al-Ahsa, Saudi Arabia
* Corresponding Author: Theyazn H. H. Aldhyani. Email:
Computer Systems Science and Engineering 2021, 38(1), 25-37. https://doi.org/10.32604/csse.2021.014992
Received 31 October 2020; Accepted 25 January 2021; Issue published 01 April 2021
Abstract
Touch gesture recognition is an important aspect in human–robot interaction, as it makes such interaction effective and realistic. The novelty of this study is the development of a system that recognizes human–animal affective robot touch (HAART) using a deep learning algorithm. The proposed system was used for touch gesture recognition based on a dataset provided by the Recognition of the Touch Gestures Challenge 2015. The dataset was tested with numerous subjects performing different HAART gestures; each touch was performed on a robotic animal covered by a pressure sensor skin. A convolutional neural network algorithm is proposed to implement the touch recognition system from row inputs of the sensor devices. The leave-one-subject-out cross-validation method was used to validate and evaluate the proposed system. A comparative analysis between the results of the proposed system and the state-of-the-art performance is presented. Findings show that the proposed system could recognize the gestures in almost real time (after acquiring the minimum number of frames). According to the results of the leave-one-subject-out cross-validation method, the proposed algorithm could achieve a classification accuracy of 83.2%. It was also superior compared with existing systems in terms of classification ratio, touch recognition time, and data preprocessing on the same dataset. Therefore, the proposed system can be used in a wide range of real applications, such as image recognition, natural language recognition, and video clip classification.Keywords
Cite This Article
Citations
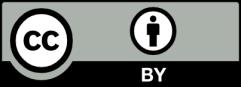
This work is licensed under a Creative Commons Attribution 4.0 International License , which permits unrestricted use, distribution, and reproduction in any medium, provided the original work is properly cited.