Open Access
ARTICLE
RP-NBSR: A Novel Network Attack Detection Model Based on Machine Learning
1 School of Computer Science & Technology, Henan Polytechnic University, Jiaozuo Henan, 454000, China
2 College of Computer Science & Technology, Jilin University, Changchun Jilin, 130012, China
3 Department of Computer Science, University College London, London, United Kingdom
* Corresponding Author: Hui Wang. Email:
Computer Systems Science and Engineering 2021, 37(1), 121-133. https://doi.org/10.32604/csse.2021.014988
Received 31 October 2020; Accepted 16 December 2020; Issue published 05 February 2021
Abstract
The rapid progress of the Internet has exposed networks to an increased number of threats. Intrusion detection technology can effectively protect network security against malicious attacks. In this paper, we propose a ReliefF-P-Naive Bayes and softmax regression (RP-NBSR) model based on machine learning for network attack detection to improve the false detection rate and F1 score of unknown intrusion behavior. In the proposed model, the Pearson correlation coefficient is introduced to compensate for deficiencies in correlation analysis between features by the ReliefF feature selection algorithm, and a ReliefF-Pearson correlation coefficient (ReliefF-P) algorithm is proposed. Then, the Relief-P algorithm is used to preprocess the UNSW-NB15 dataset to remove irrelevant features and obtain a new feature subset. Finally, naïve Bayes and softmax regression (NBSR) classifier is constructed by cascading the naïve Bayes classifier and softmax regression classifier, and an attack detection model based on RP-NBSR is established. The experimental results on the UNSW-NB15 dataset show that the attack detection model based on RP-NBSR has a lower false detection rate and higher F1 score than other detection models.Keywords
Cite This Article
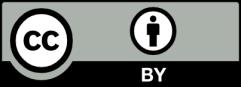
This work is licensed under a Creative Commons Attribution 4.0 International License , which permits unrestricted use, distribution, and reproduction in any medium, provided the original work is properly cited.