Open Access
ARTICLE
ASRNet: Adversarial Segmentation and Registration Networks for Multispectral Fundus Images
1 School of Information Science and Engineering, Shandong Normal University, Jinan, 250358, China
2 Key Laboratory of Intelligent Computing & Information Security in Universities of Shandong, Shandong Provincial Key Laboratory for Novel Distributed Computer Software Technology, Institute of Biomedical Sciences, Shandong Normal University, Jinan, 250358, China
3 Shandong Provincial Hospital Affiliated to Shandong University, Jinan, 250021, China
4 INSA Lyon, University of Lyon, CNRS, Inserm, Villeurbanne, 69621, Cedex, France
* Corresponding Author: Yuanjie Zheng. Email:
Computer Systems Science and Engineering 2021, 36(3), 537-549. https://doi.org/10.32604/csse.2021.014578
Received 30 September 2020; Accepted 19 November 2020; Issue published 18 January 2021
Abstract
Multispectral imaging (MSI) technique is often used to capture images of the fundus by illuminating it with different wavelengths of light. However, these images are taken at different points in time such that eyeball movements can cause misalignment between consecutive images. The multispectral image sequence reveals important information in the form of retinal and choroidal blood vessel maps, which can help ophthalmologists to analyze the morphology of these blood vessels in detail. This in turn can lead to a high diagnostic accuracy of several diseases. In this paper, we propose a novel semi-supervised end-to-end deep learning framework called “Adversarial Segmentation and Registration Nets” (ASRNet) for the simultaneous estimation of the blood vessel segmentation and the registration of multispectral images via an adversarial learning process. ASRNet consists of two subnetworks: (i) A segmentation module S that fulfills the blood vessel segmentation task, and (ii) A registration module R that estimates the spatial correspondence of an image pair. Based on the segmention-driven registration network, we train the segmentation network using a semi-supervised adversarial learning strategy. Our experimental results show that the proposed ASRNet can achieve state-of-the-art accuracy in segmentation and registration tasks performed with real MSI datasets.Keywords
Cite This Article
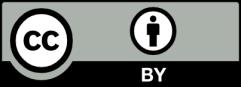
This work is licensed under a Creative Commons Attribution 4.0 International License , which permits unrestricted use, distribution, and reproduction in any medium, provided the original work is properly cited.