Open Access
ARTICLE
Highway Cost Prediction Based on LSSVM Optimized by Intial Parameters
1 School of Mechanics and Civil Engineering, China University of Mining and Technology-Beijing, Beijing, 100083, China
2 School of Information Engineering, Yangzhou University, Yangzhou, 225127, China
* Corresponding Author: Shuang Liu. Email:
Computer Systems Science and Engineering 2021, 36(1), 259-269. https://doi.org/10.32604/csse.2021.014343
Received 15 September 2020; Accepted 19 October 2020; Issue published 23 December 2020
Abstract
The cost of highway is affected by many factors. Its composition and calculation are complicated and have great ambiguity. Calculating the cost of highway according to the traditional highway engineering estimation method is a completely tedious task. Constructing a highway cost prediction model can forecast the value promptly and improve the accuracy of highway engineering cost. This work sorts out and collects 60 sets of measured data of highway engineering; establishes an expressway cost index system based on 10 factors, including main route mileage, roadbed width, roadbed earthwork, and number of bridges; and processes the data through principal component analysis (PCA) and hierarchical cluster analysis. Particle swarm optimization (PSO) is used to obtain the optimal parameter combination of the regularization parameter and the kernel function width coefficient in least squares support vector machine (LSSVM). Results show that the average relative and mean square errors of the PCA-PSO-LSSVM model are 0.79% and 10.01%, respectively. Compared with BP neural networks and unoptimized LSSVM model, the PCA-PSO-LSSVM model has smaller relative errors, better generalization ability, and higher prediction accuracy, thereby providing a new method for highway cost prediction in complex environments.Keywords
Cite This Article
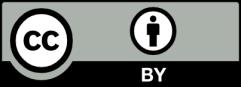
This work is licensed under a Creative Commons Attribution 4.0 International License , which permits unrestricted use, distribution, and reproduction in any medium, provided the original work is properly cited.