Open Access
ARTICLE
Three-Dimensional Measurement Using Structured Light Based on Deep Learning
1 School of Mechanical Engineering, North China University of Water Conservancy and Hydroelectric Power, Zhengzhou, 450045, China
2 Department of Computer Engineering, National Institute of Technology, Kurukshetra, 136119, India
3 Department of Computer Science and Information Engineering, Asia University, 41449, Taiwan
* Corresponding Author: Tao Zhang. Email:
Computer Systems Science and Engineering 2021, 36(1), 271-280. https://doi.org/10.32604/csse.2021.014181
Received 04 September 2020; Accepted 13 November 2020; Issue published 23 December 2020
Abstract
Three-dimensional (3D) reconstruction using structured light projection has the characteristics of non-contact, high precision, easy operation, and strong real-time performance. However, for actual measurement, projection modulated images are disturbed by electronic noise or other interference, which reduces the precision of the measurement system. To solve this problem, a 3D measurement algorithm of structured light based on deep learning is proposed. The end-to-end multi-convolution neural network model is designed to separately extract the coarse- and fine-layer features of a 3D image. The point-cloud model is obtained by nonlinear regression. The weighting coefficient loss function is introduced to the multi-convolution neural network, and the point-cloud data are continuously optimized to obtain the 3D reconstruction model. To verify the effectiveness of the method, image datasets of different 3D gypsum models were collected, trained, and tested using the above method. Experimental results show that the algorithm effectively eliminates external light environmental interference, avoids the influence of object shape, and achieves higher stability and precision. The proposed method is proved to be effective for regular objects.Keywords
Cite This Article
Citations
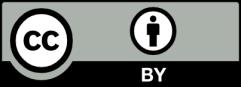
This work is licensed under a Creative Commons Attribution 4.0 International License , which permits unrestricted use, distribution, and reproduction in any medium, provided the original work is properly cited.