Open Access
ARTICLE
A Novel Fuzzy Rough Sets Theory Based CF Recommendation System
1 Assistant Professor, Department of IT, ULTRA College of Engineering & Technology for Women, Madurai
2 Professor,Department of Electronics and Communication Engineering, PSNA College of Engineering and Technology, Dindigul
E-mail: rkumardgl@gmail.com, jayanthi.ramu@gmail.com
Computer Systems Science and Engineering 2019, 34(3), 123-129. https://doi.org/10.32604/csse.2019.34.123
Abstract
Collaborative Filtering (CF) is one of the popular methodology in recommender systems. It suffers from the data sparsity problem, recommendation inaccuracy and big-error in predictions. In this paper, the efficient advisory tool is implemented for the younger generation to choose their right career based on their knowledge. It acquires the notions of indiscernible relation from Fuzzy Rough Sets Theory (FRST) and propose a novel algorithm named as Fuzzy Rough Set Theory Based Collaborative Filtering Algorithm (FRSTBCF). To evaluate the model, data is prepared using the cross validation method. Based on that, ratings are evaluated by calculating the MAE (mean average error), MSE (means squared error) and RMSE (root means squared error) values. Further the correctness of the model is measured by finding rates like Accuracy, Specificity, Sensitivity, Precision & False Positive Rate. The proposed FRSTBCF algorithm is compared with the traditional algorithms experiment results such as Item Based Collaborative Filtering using the cosine similarity (IBCF-COS), IBCF using the pearson correlation (IBCF-COR), IBCF using the Jaccard similarity (IBCF-JAC) and Singular Value Decomposition approximation (SVD). The proposed algorithm gives better error rate and its precision value is comparatively identical with the existing system.Keywords
Cite This Article
Citations
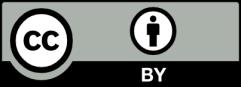