Open Access
ARTICLE
An Iteration-Based Differentially Private Social Network Data Release
1 School of Information Techonolgy, Deakin University, Burwood, Australia
E-mail: t.zhu@deakin.edu.au, ymengm@deakin.edu.au, yang.xiang@deakin.edu.au, wanlei.zhou@deakin.edu.au
2 School of Information and Security Engineering, Zhongnan University of Economics and Law, Wuhan, China
E-mail: pingxiong@znufe.edu.cn
Computer Systems Science and Engineering 2018, 33(2), 61-69. https://doi.org/10.32604/csse.2018.33.061
Abstract
Online social networks provide an unprecedented opportunity for researchers to analysis various social phenomena. These network data is normally represented as graphs, which contain many sensitive individual information. Publish these graph data will violate users’ privacy. Differential privacy is one of the most influential privacy models that provides a rigorous privacy guarantee for data release. However, existing works on graph data publishing cannot provide accurate results when releasing a large number of queries. In this paper, we propose a graph update method transferring the query release problem to an iteration process, in which a large set of queries are used as update criteria. Compared with existing works, the proposed method enhances the accuracy of query results. The extensive experiment proves that the proposed solution outperforms two state-of-the-art methods, the Laplace method and the correlated method, in terms of Mean Absolute Value. It means our methods can retain more utility of the queries while preserving the privacy.Cite This Article
Citations
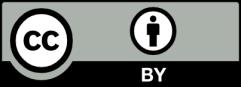
This work is licensed under a Creative Commons Attribution 4.0 International License , which permits unrestricted use, distribution, and reproduction in any medium, provided the original work is properly cited.