Open Access
ARTICLE
Rank-Order Correlation-Based Feature Vector Context Transformation for Learning to Rank for Information Retrieval
Dept. of Operation, Visitor Service, Collection and Information Management, National Museum of Natural Science, Taichung 40453, Taiwan
E-mail: jenyuan@mail.nmns.edu.tw
* Corresponding Author: Tel.: +886 4 2322 6940x728; fax: +886 4 2323 2621
Computer Systems Science and Engineering 2018, 33(1), 41-52. https://doi.org/10.32604/csse.2018.33.041
Abstract
As a crucial task in information retrieval, ranking defines the preferential order among the retrieved documents for a given query. Supervised learning has recently been dedicated to automatically learning ranking models by incorporating various models into one effective model. This paper proposes a novel supervised learning method, in which instances are represented as bags of contexts of features, instead of bags of features. The method applies rank-order correlations to measure the correlation relationships between features. The feature vectors of instances, i.e., the 1st-order raw feature vectors, are then mapped into the feature correlation space via projection to derive the context-level feature vectors, i.e., the 2nd-order context feature vectors. As for ranking model learning, Ranking SVM is employed with the 2nd-order context feature vectors as the input. The proposed method is evaluated using the LETOR benchmark datasets and is found to perform well with competitive results. The results suggest that the learning method benefits from the rank-order-correlation-based feature vector context transformation.Keywords
Cite This Article
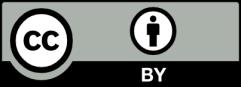
This work is licensed under a Creative Commons Attribution 4.0 International License , which permits unrestricted use, distribution, and reproduction in any medium, provided the original work is properly cited.