Open Access
ARTICLE
Intrusion Detection and Prevention Model for Blockchain Based IoMT Applications
Department of Computer Science, College of Computer in Al-Lith, Umm Al-Qura University, Makkah, Saudi Arabia
* Corresponding Author: Jameel Almalki. Email:
(This article belongs to the Special Issue: Explainable AI and Cybersecurity Techniques for IoT-Based Medical and Healthcare Applications)
Computer Systems Science and Engineering 2024, 48(1), 131-152. https://doi.org/10.32604/csse.2023.038085
Received 26 November 2022; Accepted 17 February 2023; Issue published 26 January 2024
Abstract
The recent global pandemic has resulted in growth in the medical and healthcare sectors. Applications used in these domains have become more advanced and digitally integrated. Sensor-based Internet of Things (IoT) devices are increasing in healthcare and medical units. The emerging trend with the use of IoT devices in medical healthcare is termed as Internet of Medical Things (IoMT). The instruments used in these healthcare units comprise various sensors that can record patient body observations. These recorded observations are streamed across Internet-based channels to be stored and analyzed in centralized servers. Patient diagnostics are performed based on the information retrieved from these devices. Machine learning and artificial intelligence play a significant role in diagnostic mechanisms and identifying diseases across observation sets. The data collected by these observation sets are analyzed closely with the help of Artificial Neural Networks (ANN). This distributed system of devices and servers raises privacy concerns. Blockchain is a technology that can preserve the privacy of the information collected from devices. A blockchain is a mechanism in which information goes across various network nodes. A centralized architecture where information flows from the sensor devices to a centralized server risks large-scale information leak. This paper proposes a prototype and a model for collecting information and protecting the data from intrusion. Any application that resides in the cloud and collects data from a sensor based IoT device can be prone to intrusion. The detection occurs at an early stage and in a fraction of the time. The model uses artificial neural networks and gets evaluated across various datasets. Improved accuracy and performance with data analyzed at the IoT-based devices prove the efficiency of the model proposed in this study. The stability and accuracy of the results after testing these applications make the model sustainable and acceptable across healthcare systems.Keywords
Cite This Article
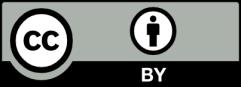
This work is licensed under a Creative Commons Attribution 4.0 International License , which permits unrestricted use, distribution, and reproduction in any medium, provided the original work is properly cited.