Open Access
REVIEW
A Literature Review on Model Conversion, Inference, and Learning Strategies in EdgeML with TinyML Deployment
1 Department of Computer Science and Artificial Intelligence, Umm Al-Qura University, Makkah Al-Mukarama, 21955, Saudi Arabia
2 Department of Computer and Network Engineering, Umm Al-Qura University, Makkah Al-Mukarama, 21955, Saudi Arabia
* Corresponding Author: Muhammad Arif. Email:
Computers, Materials & Continua 2025, 83(1), 13-64. https://doi.org/10.32604/cmc.2025.062819
Received 28 December 2024; Accepted 13 February 2025; Issue published 26 March 2025
Abstract
Edge Machine Learning (EdgeML) and Tiny Machine Learning (TinyML) are fast-growing fields that bring machine learning to resource-constrained devices, allowing real-time data processing and decision-making at the network’s edge. However, the complexity of model conversion techniques, diverse inference mechanisms, and varied learning strategies make designing and deploying these models challenging. Additionally, deploying TinyML models on resource-constrained hardware with specific software frameworks has broadened EdgeML’s applications across various sectors. These factors underscore the necessity for a comprehensive literature review, as current reviews do not systematically encompass the most recent findings on these topics. Consequently, it provides a comprehensive overview of state-of-the-art techniques in model conversion, inference mechanisms, learning strategies within EdgeML, and deploying these models on resource-constrained edge devices using TinyML. It identifies 90 research articles published between 2018 and 2025, categorizing them into two main areas: (1) model conversion, inference, and learning strategies in EdgeML and (2) deploying TinyML models on resource-constrained hardware using specific software frameworks. In the first category, the synthesis of selected research articles compares and critically reviews various model conversion techniques, inference mechanisms, and learning strategies. In the second category, the synthesis identifies and elaborates on major development boards, software frameworks, sensors, and algorithms used in various applications across six major sectors. As a result, this article provides valuable insights for researchers, practitioners, and developers. It assists them in choosing suitable model conversion techniques, inference mechanisms, learning strategies, hardware development boards, software frameworks, sensors, and algorithms tailored to their specific needs and applications across various sectors.Keywords
Cite This Article
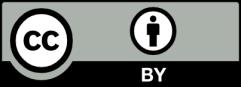
This work is licensed under a Creative Commons Attribution 4.0 International License , which permits unrestricted use, distribution, and reproduction in any medium, provided the original work is properly cited.