Open Access
TECHNICAL REPORT
NJmat 2.0: User Instructions of Data-Driven Machine Learning Interface for Materials Science
1 Department of Internet Engineering, School of Software Engineering, Nanjing University of Information Science & Technology, Nanjing, 210044, China
2 Department of Materials Physics, School of Chemistry and Materials Science, Nanjing University of Information Science & Technology, Nanjing, 210044, China
* Corresponding Author: Lei Zhang. Email:
Computers, Materials & Continua 2025, 83(1), 1-11. https://doi.org/10.32604/cmc.2025.062666
Received 24 December 2024; Accepted 17 February 2025; Issue published 26 March 2025
Abstract
NJmat is a user-friendly, data-driven machine learning interface designed for materials design and analysis. The platform integrates advanced computational techniques, including natural language processing (NLP), large language models (LLM), machine learning potentials (MLP), and graph neural networks (GNN), to facilitate materials discovery. The platform has been applied in diverse materials research areas, including perovskite surface design, catalyst discovery, battery materials screening, structural alloy design, and molecular informatics. By automating feature selection, predictive modeling, and result interpretation, NJmat accelerates the development of high-performance materials across energy storage, conversion, and structural applications. Additionally, NJmat serves as an educational tool, allowing students and researchers to apply machine learning techniques in materials science with minimal coding expertise. Through automated feature extraction, genetic algorithms, and interpretable machine learning models, NJmat simplifies the workflow for materials informatics, bridging the gap between AI and experimental materials research. The latest version (available at (accessed on 01 January 2025)) enhances its functionality by incorporating NJmatNLP, a module leveraging language models like MatBERT and those based on Word2Vec to support materials prediction tasks. By utilizing clustering and cosine similarity analysis with UMAP visualization, NJmat enables intuitive exploration of materials datasets. While NJmat primarily focuses on structure-property relationships and the discovery of novel chemistries, it can also assist in optimizing processing conditions when relevant parameters are included in the training data. By providing an accessible, integrated environment for machine learning-driven materials discovery, NJmat aligns with the objectives of the Materials Genome Initiative and promotes broader adoption of AI techniques in materials science.Keywords
Cite This Article
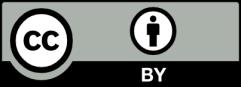
This work is licensed under a Creative Commons Attribution 4.0 International License , which permits unrestricted use, distribution, and reproduction in any medium, provided the original work is properly cited.