Open Access
ARTICLE
Leveraging Deep Learning for Precise Chronic Bronchitis Identification in X-Ray Modalities
1 School of Computing, Faculty of Technology, University of Portsmouth, Winston Churchill Ave Southsea, Portsmouth, PO1 2UP, UK
2 Portsmouth Artificial Intelligence and Data Science Center, University of Portsmouth, Winston Churchill Ave, Southsea, Portsmouth, PO1 2UP, UK
3 Department of Computer Science, College of Computer and Information Sciences, Jouf University, Sakaka, Aljouf, 72341, Saudi Arabia
4 School of Biological and Behavioural Sciences, Queen Mary University of London, London, E1 4NS, UK
5 Department of Computer Sciences, Bahria University, Lahore, Punjab, 54700, Pakistan
* Corresponding Author: Fahad Ahmad. Email:
Computers, Materials & Continua 2025, 83(1), 381-405. https://doi.org/10.32604/cmc.2025.062452
Received 18 December 2024; Accepted 10 February 2025; Issue published 26 March 2025
Abstract
Image processing plays a vital role in various fields such as autonomous systems, healthcare, and cataloging, especially when integrated with deep learning (DL). It is crucial in medical diagnostics, including the early detection of diseases like chronic obstructive pulmonary disease (COPD), which claimed 3.2 million lives in 2015. COPD, a life-threatening condition often caused by prolonged exposure to lung irritants and smoking, progresses through stages. Early diagnosis through image processing can significantly improve survival rates. COPD encompasses chronic bronchitis (CB) and emphysema; CB particularly increases in smokers and generally affects individuals between 50 and 70 years old. It damages the lungs’ air sacs, reducing oxygen transport and causing symptoms like coughing and shortness of breath. Treatments such as beta-agonists and inhaled steroids are used to manage symptoms and prolong lung function. Moreover, COVID-19 poses an additional risk to individuals with CB due to its impact on the respiratory system. The proposed system utilizes convolutional neural networks (CNN) to diagnose CB. In this system, CNN extracts essential and significant features from X-ray modalities, which are then fed into the neural network. The network undergoes training to recognize patterns and make accurate predictions based on the learned features. By leveraging DL techniques, the system aims to enhance the precision and reliability of CB detection. Our research specifically focuses on a subset of 189 lung disease images, carefully selected for model evaluation. To further refine the training process, various data augmentation and noise removal techniques are implemented. These techniques significantly enhance the quality of the training data, improving the model’s robustness and generalizability. As a result, the diagnostic accuracy has improved from 98.6% to 99.2%. This advancement not only validates the efficacy of our proposed model but also represents a significant improvement over existing literature. It highlights the potential of CNN-based approaches in transforming medical diagnostics through refined image analysis, learning capabilities, and automated feature extraction.Keywords
Cite This Article
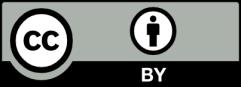
This work is licensed under a Creative Commons Attribution 4.0 International License , which permits unrestricted use, distribution, and reproduction in any medium, provided the original work is properly cited.