Open Access
ARTICLE
Enhancing LoRaWAN Sensor Networks: A Deep Learning Approach for Performance Optimizing and Energy Efficiency
Department of Information Systems, College of Computers and Information Sciences, King Saud University, Riyadh, P.O. Box 51178, Saudi Arabia
* Corresponding Author: Maram Alkhayyal. Email:
Computers, Materials & Continua 2025, 83(1), 1079-1100. https://doi.org/10.32604/cmc.2025.061836
Received 04 December 2024; Accepted 12 February 2025; Issue published 26 March 2025
Abstract
The rapid expansion of the Internet of Things (IoT) has led to the widespread adoption of sensor networks, with Long-Range Wide-Area Networks (LoRaWANs) emerging as a key technology due to their ability to support long-range communication while minimizing power consumption. However, optimizing network performance and energy efficiency in dynamic, large-scale IoT environments remains a significant challenge. Traditional methods, such as the Adaptive Data Rate (ADR) algorithm, often fail to adapt effectively to rapidly changing network conditions and environmental factors. This study introduces a hybrid approach that leverages Deep Learning (DL) techniques, namely Long Short-Term Memory (LSTM) networks, and Machine Learning (ML) techniques, namely Artificial Neural Networks (ANNs), to optimize key network parameters such as Signal-to-Noise Ratio (SNR) and Received Signal Strength Indicator (RSSI). LSTM-ANN model trained on the “LoRaWAN Path Loss Dataset including Environmental Variables” from Medellín, Colombia, and the model demonstrated exceptional predictive accuracy, achieving an R2 score of 0.999, Mean Squared Error (MSE) of 0.041, Root Mean Squared Error (RMSE) of 0.203, and Mean Absolute Error (MAE) of 0.167, significantly outperforming traditional regression-based approaches. These findings highlight the potential of combining advanced ML and DL techniques to address the limitations of traditional optimization strategies in LoRaWAN. By providing a scalable and adaptive solution for large-scale IoT deployments, this work lays the foundation for real-world implementation, emphasizing the need for continuous learning frameworks to further enhance energy efficiency and network resilience in dynamic environments.Keywords
Cite This Article
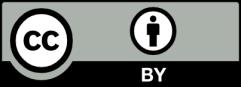
This work is licensed under a Creative Commons Attribution 4.0 International License , which permits unrestricted use, distribution, and reproduction in any medium, provided the original work is properly cited.