Open Access
ARTICLE
XGBoost-Liver: An Intelligent Integrated Features Approach for Classifying Liver Diseases Using Ensemble XGBoost Training Model
1 Business and Management Sciences Department, Purdue University, West Lafayette, IN 47907, USA
2 New Emerging Technologies and 5G Network and Beyond Research Chair, Department of Computer Engineering, College of Computer and Information Sciences, King Saud University, Riyadh, 11564, Saudi Arabia
3 Department of Computer Science, Abdul Wali Khan University Mardan, Mardan, 23200, KPK, Pakistan
* Corresponding Author: Salman Khan. Email:
Computers, Materials & Continua 2025, 83(1), 1435-1450. https://doi.org/10.32604/cmc.2025.061700
Received 01 December 2024; Accepted 05 February 2025; Issue published 26 March 2025
Abstract
The liver is a crucial gland and the second-largest organ in the human body and also essential in digestion, metabolism, detoxification, and immunity. Liver diseases result from factors such as viral infections, obesity, alcohol consumption, injuries, or genetic predispositions. Pose significant health risks and demand timely diagnosis and treatment to enhance survival rates. Traditionally, diagnosing liver diseases relied heavily on clinical expertise, often leading to subjective, challenging, and time-intensive processes. However, early detection is essential for effective intervention, and advancements in machine learning (ML) have demonstrated remarkable success in predicting various conditions, including Chronic Obstructive Pulmonary Disease (COPD), hypertension, and diabetes. This study proposed a novel XGBoost-liver predictor by integrating distinct feature methodologies, including Ranking and Statistical Projection-based strategies to detect early signs of liver disease. The Fisher score method is applied to perform global interpretation analysis, helping to select optimal features by assessing their contributions to the overall model. The performance of the proposed model has been extensively evaluated through k-fold cross-validation tests. Firstly, the performance of the proposed model is evaluated using individual and hybrid features. Secondly, the XGBoost-Liver model performance is compared to that of commonly used classifier algorithms. Thirdly, its performance is compared with the existing state-of-the-art computational models. The experimental results show that the proposed model performed better than the existing predictors, reaching an average accuracy rate of 92.07%. This paper demonstrates the potential of machine learning to improve liver disease prediction, enhance diagnostic accuracy, and enable timely medical interventions for better patient outcomes.Keywords
Cite This Article
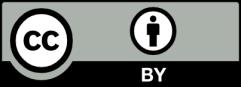
This work is licensed under a Creative Commons Attribution 4.0 International License , which permits unrestricted use, distribution, and reproduction in any medium, provided the original work is properly cited.