Open Access
ARTICLE
Multi-Scale Feature Fusion Network for Accurate Detection of Cervical Abnormal Cells
1 School of Computer and Information Science, Chongqing Normal University, Chongqing, 401331, China
2 School of Artificial Intelligence, Chongqing University of Technology, Chongqing, 401331, China
* Corresponding Author: Yang Zhang. Email:
# These authors contributed equally to this work
(This article belongs to the Special Issue: Research on Deep Learning-based Object Detection and Its Derivative Key Technologies)
Computers, Materials & Continua 2025, 83(1), 559-574. https://doi.org/10.32604/cmc.2025.061579
Received 28 December 2024; Accepted 09 January 2025; Issue published 26 March 2025
Abstract
Detecting abnormal cervical cells is crucial for early identification and timely treatment of cervical cancer. However, this task is challenging due to the morphological similarities between abnormal and normal cells and the significant variations in cell size. Pathologists often refer to surrounding cells to identify abnormalities. To emulate this slide examination behavior, this study proposes a Multi-Scale Feature Fusion Network (MSFF-Net) for detecting cervical abnormal cells. MSFF-Net employs a Cross-Scale Pooling Model (CSPM) to effectively capture diverse features and contextual information, ranging from local details to the overall structure. Additionally, a Multi-Scale Fusion Attention (MSFA) module is introduced to mitigate the impact of cell size variations by adaptively fusing local and global information at different scales. To handle the complex environment of cervical cell images, such as cell adhesion and overlapping, the Inner-CIoU loss function is utilized to more precisely measure the overlap between bounding boxes, thereby improving detection accuracy in such scenarios. Experimental results on the Comparison detector dataset demonstrate that MSFF-Net achieves a mean average precision (mAP) of 63.2%, outperforming state-of-the-art methods while maintaining a relatively small number of parameters (26.8 M). This study highlights the effectiveness of multi-scale feature fusion in enhancing the detection of cervical abnormal cells, contributing to more accurate and efficient cervical cancer screening.Keywords
Cervical cancer ranks as the fourth most common cancer in women globally, with around 604,000 new cases and 342,000 deaths in 2020 [1]. Notably, while the development of cervical cancer is relatively slow-typically taking around 10 years to progress from high-risk HPV infection through precancerous abnormalities to invasive cancer-the disease is highly treatable if detected early. This lengthy progression period offers a valuable window for effective screening and intervention, with timely screening shown to reduce incidence by at least 60% [2].Currently, the most commonly used method for cervical cancer detection is cytology-based screening, primarily conducted through liquid-based cervical cytology using Thinprep cytologic test(TCT) [3]. Physicians collect cervical cell samples from patients, prepare cervical cell slides, and perform visual inspections under a microscope, along with cytopathological analyses. Pathologists provide preliminary diagnostic opinions by evaluating cell types and morphological characteristics, such as nuclear size and the nuclear-to-cytoplasm ratio. However, manual analysis of cell slides is tedious, time-consuming, and highly subjective, which increases the likelihood of errors [4]. Moreover, since abnormal cells constitute only a small fraction of image samples, this further exacerbates the waste of medical resources.
With advancements in image processing technology and computational power, deep learning-based analysis of cervical cancer cell images has become increasingly widespread. Early detection methods for cervical abnormal cells typically involve three key steps: cell segmentation (cytoplasm and nucleus), feature extraction, and cell classification [5]. The identification of abnormal cells relies on morphological changes in the nucleus and cytoplasm, with cell segmentation considered a crucial initial step. However, cervical cell segmentation remains a challenge due to high inter-cell similarity, significant size variations, and the complexities of the imaging environment [6]. The subsequent classification relies on the extraction of handcrafted features such as cell shape, size, color, and texture, whose accuracy is inherently tied to the precision of segmentation [7]. Once segmentation errors occur, classification accuracy can be significantly reduced, ultimately impacting the detection precision of abnormal cervical cells.
To address this issue, the direct application of end-to-end object detectors for detecting abnormal cervical cells has proven to be an effective solution. Faster R-CNN [8] and RetinaNet [9] have been directly applied to large cervical cell datasets for detection [10]. However, cervical cell images differ from other natural images, such as those of animals or vehicles, due to their unique characteristics. Therefore, it is essential to fully consider the specific morphological features of cervical cell images when detecting abnormal cells. In the detection of abnormal cervical cells, there may be small differences between classes (inter-class) and large variations within the same class (intra-class). As shown in Fig. 1d illustrates two cells from different lesion categories that appear similar in appearance, while (e) shows two cells from the same lesion category that differ significantly in appearance. Additionally, cervical cell images exhibit notable variations in cell size, as seen in Fig. 1; some cells are very small (e.g., Fig. 1b), while others are relatively large (e.g., Fig. 1c). Therefore, relying solely on local inference is often insufficient. Clinically, cytopathologists typically compare the target cell with surrounding cells as a reference to determine whether it is normal or abnormal. Existing methods [11–13] lack feature interactions between cells, which can lead to suboptimal classification performance.
Figure 1: Description of abnormal cervical cells in the Comparison Detector dataset. (a) Size distribution histogram of abnormal cervical cells (in pixels). (b) Smaller cervical abnormal cells. (c) Larger cervical abnormal cells. The blue and red boxes in (a) indicate the positions of the cell sizes in (b) and (c) within the overall distribution. (d) Two cell clusters from different lesion categories that appear similar in appearance, making them difficult to distinguish, showing minimal differences between categories. (e) Similarly, two cervical cells categorized as LSIL, but with significant differences in appearance, indicating considerable variation within the same category
To more closely replicate the way pathologists reference surrounding cells during slide examination to identify abnormalities and enhance feature interaction between cells, while addressing the challenges associated with subtle intercellular differences and notable size variations, this study proposes a multi-scale feature fusion method (MSFF-Net) for detecting abnormal cervical cells. Specifically, we designed a feature extraction framework based on a pyramid pooling structure to fully leverage contextual relationships among cervical cells and to strengthen feature interactions between them. Additionally, we developed an attention-based multi-scale feature fusion approach that efficiently integrates multi-scale features and accentuates critical characteristics, thereby better accommodating substantial variations in cell size.
The primary contributions of this study are as follows:
1. To emulate how pathologists reference surrounding cell features to identify abnormalities and to enhance feature interactions between cells, this study introduces a multi-scale feature fusion network (MSFF-Net) designed to address substantial intra-class variability, minimal inter-class differences, and significant cell size variations.
2. Inspired by the way pathologists reference surrounding cell features, this study adopts an approach focused on capturing long-range contextual information, introducing a cross-scale pooling method (CSPM) for multi-scale feature extraction to enrich both local and global information.
3. Expanding on the idea of multi-scale feature fusion, this study enhances cross-scale feature integration and dynamically adjusts feature weights, resulting in a multi-scale fusion attention module (MSFA) to effectively handle considerable cervical cell size variations.
4. The proposed method was validated on publicly available datasets, showing better overall performance than current state-of-the-art general and specific detection methods. achieving a 2.6% increase in detection accuracy.
In recent years, with the widespread application of computers in medical imaging, the automatic detection of cervical lesion cells has become possible. Nasir et al. [14] utilized a federated machine learning technique with Bayesian regularization to predict cervical cancer. Xiang et al. [15] modified YOLOv3 to improve recognition performance for difficult categories by integrating a task-specific classifier into the original model, enabling the detection of 10 distinct abnormal cell types. Ontor, Md Zahid Hasan, et al. [16]compared the performance of YOLOv5 and its variants on cervical cell detection to identify the most suitable model for constructing a low-cost automated system for early-stage cervical cancer diagnosis. Jin et al. [17] proposed FuseDLAM to rapidly localize single squamous epithelial cells, effectively leveraging extracted features for segmentation and classification, thus reducing the reliance on expensive manual annotations. However, their approach relied solely on local information from cervical cell images, disregarding the broader contextual information.
To better leverage the full contextual scope for detecting abnormal cells. Liang et al. [18] proposed a global context-aware framework, incorporating an additional image-level classification branch to reduce false-positive predictions. Liang et al. [19] enriched the attention-based RoI features by encoding the relationships between cells and global context information. However, cell size variability is common in microscope images, and these methods continue to face challenges in addressing this scale diversity, which may result in missed diagnoses. To address the issue of size inconsistency, Cao et al. [20] implemented channel and spatial attention mechanisms within Faster R-CNN to improve cell detection performance, effectively addressing the issue of size variation by refining feature extraction to discern which features to emphasize or suppress. However, their approach did not consider interactions between cell features. In studies related to auxiliary screening for cervical cancer, several methods have been proposed to address the issue of diverse object scales. Duan et al. [21] employed heterogeneous receptive field convolutions to extract multi-scale features and address size inconsistencies in lesion regions of colpo scopic images. Khan et al. [22] used an ensemble learning approach based on multi-scale Transformers for analyzing whole slide images (WSI) to detect cells at different stages. These methods inspired us to adopt a multi-scale approach to address the scale diversity of cervical cells, which enhances the model’s adaptability to objects of varying sizes [23].
This study distinguishes itself from existing approaches by incorporating multi-scale feature fusion, which effectively integrates local details and global contextual information while enhancing interactions between cell features. Unlike traditional methods that primarily focus on single-scale features or rely solely on local information, the proposed approach demonstrates superior adaptability to cell size diversity and leverages both contextual and inter-cell relational features, resulting in improved detection accuracy and robustness.
This study presents a multi-scale feature fusion method (MSFF-Net) for cervical abnormal cell detection based on YOLOv8, designed to enhance inter-cell feature interactions to more closely replicate the examination approach of pathologists. Compared to existing methods, MSFF-Net achieves better accuracy by enhancing inter-cell feature interactions and integrating both local and global contextual information. Specifically, the CSPM module addresses challenges posed by subtle differences between abnormal and normal cells, while the MSFA module adapts to the multi-scale characteristics of detection targets. Additionally, the Inner-CIoU loss function improves bounding box regression for overlapping or ambiguous lesions, making MSFF-Net particularly suitable for cervical cancer screening in clinical practice.
The MSFF-Net architecture, illustrated in Fig. 2, comprises three main components: the backbone network, neck module, and detection heads. Initially, the input image undergoes preprocessing with Mosaic high-order data augmentation strategy and adaptive image adjustment strategy. The backbone network is responsible for feature extraction, merging high-resolution but semantically shallow feature maps with low-resolution, semantically rich deep feature maps to produce high-resolution, semantically enriched feature maps that enhance detection performance. The CSPM module combines multiple grouped convolution blocks (including 1×1 and 3×3 convolutions) with three serial max pooling layers of varying receptive fields, thereby improving cell feature interaction while reducing parameter count. The neck module, which incorporates FPN and PAN structures, fuses deep and shallow features and utilizes the MSFA module for efficient multi-scale feature integration, resulting in more informative fused features. Finally, three detection heads, specifically tailored for large, medium, and small targets, are employed to achieve precise detection of cervical abnormal cells.
Figure 2: Structure diagram of MSFF-Net. The figure primarily consists of three parts: (1) a flowchart of the network’s detection process; (2) the CSPM module for capturing contextual information; and (3) the MSFA, which is placed in front of three detection heads to fuse multi-scale features for targets of different sizes
The CSPM module is designed to address the limitations of YOLOv8’s original SPPF module, which struggles with capturing long-range contextual information. Unlike traditional pooling approaches, CSPM combines grouped convolutions with multi-scale pooling to effectively capture features across diverse receptive fields. This design allows CSPM to integrate both detailed local features and global context, mimicking the diagnostic behavior of pathologists who compare suspicious abnormal cells with surrounding normal cells. The structure of CSPM is depicted in Fig. 2a.
The CSPM module first segments the feature maps along the channel dimension [24], which reduces computational load and parameter count while preserving information integrity. Specifically, it divides the input feature map into two parts along the channel dimension, applies different operations to each part, and then reassembles the feature maps. One part performs simple feature extraction, while the other applies multiple convolutions followed by pooling operations at different scales to capture features from diverse receptive fields, thus enhancing the model’s ability to detect multi-scale targets. This multi-scale pooling strategy uses a series of pooling layers of varying sizes, allowing the feature maps to progressively acquire contextual information at multiple scales through pooling at each layer. The three pooling layers, each with a distinct size, capture detailed features, medium-range features, and long-range contextual information, respectively. By concatenating features extracted from these multi-scale pooling layers, the CSPM module generates a feature map rich in scale information, incorporating both detailed local information and comprehensive global context. Additionally, by integrating multiple convolutional layers of varying sizes, the module further refines and enhances complex image features. When the feature maps from both branches are reassembled, the CSPM module produces a richer feature representation, ultimately improving object detection performance.
The process is described by the following formula:
G1,G2=Group(Xinput)(1)
Fconv1=Conv1×1(G1)(2)
Fconv2=Conv1×1(Conv3×3(Conv1×1(G2)))(3)
Pk=i=MaxPool2Dk=i(Fconv2)(i=3,5,9)(4)
Foutput=Conv1×1(Concat(Conv3×3(Conv1×1(Concat(Pk=3,Pk=5,Pk=9))),Fconv1))(5)
In the formula, G1,G2 represent the grouped input features Xinput, where G1 is used to extract local features Fconv1, and G2 undergoes multiple convolution layers to generate deep features Fconv2. Then, Fconv2 is processed through max-pooling operations with different kernel sizes (k=3,5,9) to produce multi-scale features Pk=3,Pk=5,Pk=9. Finally, the multi-scale features are fused with local features and passed through a 1×1 convolution to generate the final output Foutput. This process combines local and multi-scale information, enhancing the model’s detection capability. Applied to the cervical cell detection, the CSPM module effectively captures both local and global cell features during the feature extraction stage by integrating multiple convolutional and multi-level pooling layers, thereby improving the accuracy and reliability of abnormal cervical cell detection.
3.2 Multi-Scale Fusion Attention
Given the significant variation in the sizes, shapes, and orientations of cervical abnormal cells, an effective detection approach must handle diverse features. This study introduces the multi-scale feature fusion attention module (MSFA), which enhances detection by employing multi-branch depthwise convolutions and attention mechanisms. Strip depthwise convolutions address irregularly shaped cells, while spatial attention mechanisms improve abnormal cell localization. These designs enhance MSFF-Net’s adaptability to diverse clinical imaging conditions. As illustrated in Fig. 2b, the MSFA module is primarily composed of the following components:
First, a Depthwise Convolution (Dconv 5×5) [25] is employed to decrease the parameter count while aggregating local information. Next, multi-branch depthwise convolution kernels of different sizes [26] are employed to capture feature information in various directions (horizontal and vertical). Since the three detection heads are primarily designed for detecting targets of three different sizes, the MSFA used in front of each detection head also utilizes multi-branch depthwise convolutions of varying sizes. Then, a 1×1 convolution is applied to model the relationships between different channels. The attention weights are applied to each channel of the original feature map, generating an attention-weighted map that highlights relevant channels and suppresses irrelevant ones.
Additionally, inspired by the feature of spatial attention [27], thisThe module improves abnormal cell localization accuracy. MaxPool and AvgPool are applied to extract local and maximal features, respectively, creating features at different contextual scales. The features are then combined along the channel axis to create a feature map that incorporates multi-scale contextual information, which is processed by a 7×7 convolution to generate spatial attention weights. The obtained spatial attention weights are applied to the feature map, adjusting the importance of each spatial location. This enhances key features and diminishes less important ones.
The process is described by the following formula:
Mc(F)=Conv1×1(3∑i=0Scalei(DConv5×5(F)))(6)
Scalei=DConvkj×1(DConv1×kj)(i∈{1,2,3},j∈{1,2,3})(7)
F′=Mc(F)⊗F(8)
Ms=σ(Conv7×7([Avgpool(F′); Maxpool(F′)]))(9)
F′′=Ms(F′)⊗F′(10)
F represents the input features, F″ is the final output. ⊗ denotes the element-wise matrix multiplication operation. DConv represents Depthwise Convolution. Scalei,i∈{1,2,3} denotes the i-th branch of the multi-branch depthwise convolution stage in the figure. Here DConvkj×1 and Dconv1×kj represent d(kj×1) and d(1×kj), indicating the sizes of the strip depthwise convolution kernels used in this study. Since the MSFF-Net proposed in this study has three detection heads, each is used to detect targets larger than 8×8, 16×16, 32×32. Thus, each kernel size in the multi-branch depthwise convolution of the MSFA in front of the three detection heads differs, adjusted according to the target sizes of the corresponding detection heads. In each branch, this study employs two strip depthwise convolutions to approximate standard depthwise convolutions. On one hand, this approach significantly reduces computational load and parameter count, thereby enhancing the model’s training and inference speed. On the other hand, since cervical lesion cells often exhibit irregular shapes and sizes, the directional kernel design of strip depthwise convolutions is more suitable for adapting to these characteristics.
The environment of cervical cells is relatively complex, often accompanied by phenomena such as cell adhesion, overlap, and obstruction. When the detection targets overlap, CIoU considers only the overlapping area of the bounding boxes [28], which can result in an IoU value close to 1 while neglecting the relative position and shape differences of the bounding boxes. This situation can mislead the detection network, making it difficult to accurately distinguish overlapping targets. To tackle this problem, this study improves the original bounding box loss function.
Zhang et al. analyzed the process of bounding box regression and proposed the Inner-IoU [29] method that calculates the IoU loss through auxiliary bounding boxes. On this basis, this study further optimizes the CIoU loss function and forms the Inner-CIoU loss function. The method, when dealing with overlapping cervical cells, comprehensively takes into account the relative positions and size differences of the bounding boxes. It not only focuses on the overlapping areas but also captures the positional information between cells with blurred boundaries, thus helping the model handle boundary ambiguity more effectively. The size of the auxiliary bounding box in Inner-IoU can be controlled by the scale factor ratio. The ratio is a scaling factor, and when the ratio is 1, the size of the auxiliary bounding box is equal to the actual bounding box. In this study, the ratio is set to 0.7. Its calculation method is shown in Eq. (17).
bgtl=xgtc−wgt×ratio2,bgtr=xgtc+wgt×ratio2(11)
bgtt=ygtc−hgt×ratio2,bgtb=ygtc+hgt×ratio2(12)
bl=xc−w×ratio2,br=xc+w×ratio2(13)
bt=yc−h×ratio2,bb=yc+h×ratio2(14)
inter=(min(bgtr,br)−max(bgtl,bl))×(min(bgtb,bb)−max(bgtt,bt))(15)
union=(wgt×hgt)×(ratio)2+(w×h)×(ratio)2−inter(16)
IoUinner=interunion(17)
Unlike the conventional IoU loss, when the ratio is below 1, the auxiliary bounding box is smaller than the actual one, limiting the effective regression range. However, its larger gradient accelerates convergence for high IoU samples. When the ratio exceeds 1, the larger auxiliary bounding box expands the regression range, aiding low IoU regression. The Inner-CIoU loss function is expressed as:
LInner−CIoU=1−IoUinner+ρ2(b,bgt)c2+αϑ(18)
α=ϑ(1−IoU)+ϑ(19)
ϑ=4π2(arctanwgthgt−arctanwh)2(20)
where α is the weight function, ϑ is used to measure the aspect ratio. Inner-CIoU not only introduces the auxiliary bounding box but also takes into account the center point distance and the aspect ratio of the bounding box.
To verify the effectiveness of the proposed method, mean Average Precision (mAP) is utilized as the primary evaluation metric, and comparative experiments alongside ablation studies are conducted on the Comparison Detector dataset. In this study, YOLOv8 serves as the baseline network, with accuracy assessed on the cervical cell dataset based on the proposed approach. The comparative experiments demonstrate that the proposed method surpasses existing techniques in both segmentation accuracy and parameter efficiency for abnormal cell detection tasks. Furthermore, the ablation studies indicate that the CSPM and MSFA modules, as integral components of the network, consistently enhance detection performance and prove effective in the context of cervical cell images. As a reliable metric for evaluating detection quality, mAP further corroborates the effectiveness of the proposed approach.
4.1 Implementation Details and Evaluation Metrics
In this experiment, the input images are of resolution 640×640 and undergo data augmentation to enhance performance. The training uses the SGD optimizer with a momentum of 0.937, an initial learning rate of 0.01, a batch size of 16, and a weight decay of 0.0005. The total training duration is set to 100 epochs. Detection accuracy is evaluated using the mAP metric, where the precision and recall for 11 categories are first calculated during the mAP computation process.
Precision=TPTP+FP(21)
Recall=TPTP+FN(22)
In formulas Eqs. (21) and (22), TP represents the number of correctly detected lesion cells, FP represents the number of incorrectly detected lesion cells, and FN represents the number of missed lesion cells. The average precision (AP) for each category is calculated as follows:
AP=∫10P(R)dR(23)
mAP=∑mi=1APim(24)
Finally, the mAP is obtained by summing the AP values of all categories and taking the average. In addition, “params” is used as an evaluation metric to measure the complexity and training difficulty of the model, with these factors directly impacting the model’s performance and training efficiency.
This study evaluates the proposed MSFF-Net on two datasets used for cervical cell lesion detection.
Comparison detector: This publicly available cervical cytology image dataset, sourced from [11], is intended for detecting cervical lesions. The dataset consists of 7,410 cervical microscopic images, which were extracted from whole-slide images (WSI) captured with the Pannoramic WSI II digital slide scanner. The specimens were processed using the Papsmear (Pap) staining method. The dataset is divided into a training set with 6,666 images and a test set with 744 images. All images were annotated by experienced pathologists. The cell dataset includes 11 categories: ASCUS (atypical squamous cells of undetermined significance), LSIL (low-grade squamous intraepithelial lesions), ASCH (atypical squamous cells, cannot exclude high-grade squamous intraepithelial lesion), HSIL (high-grade squamous intraepithelial lesions), SCC (squamous cell carcinoma), TRICH (trichomonas), CAND (candida), AGC (atypical glandular cells), FLORA(flora), HERPS (herpes), and ACTIN(actinomyces), as detailed in Table 1. Some example images are shown in Fig. 3. The dataset presents challenges due to the large variety of cell types, imbalanced class distribution, and complex backgrounds. To accurately detect the lesion cells, the detection model needs to have strong feature extraction and generalization capabilities.
Figure 3: Cervical cell/clumps at different stages of lesions
DCCL: The dataset, jointly released by Huawei Cloud and KingMed Diagnostics, includes 933 positive cases and 234 normal cases. Annotations for some cervical epithelial cells were performed by six experienced pathologists. The dataset consists of a total of 6301 images, with 3343 for training, 1193 for validation, and 1765 for testing. It is primarily used for semi-supervised learning [10]. The labels in the dataset include six lesion types: ASCUS, LSIL, HSIL, ASCH, SCC, and AGC, along with one false-positive label: NILM.
This study compares the proposed MSFF-Net abnormal cell detection method with currently available cervical abnormal cell detection methods, as well as some general detectors. Table 2 shows the detection results of various networks, with experimental results from the original studies.
The experimental results demonstrate that MSFF-Net network demonstrates superior detection performance on the Comparison detector dataset. From the data in the table, the following can be observed: (1) Based on YOLOv8, the proposed approach enhances detection accuracy, validating the effectiveness of the MSFF-Net. (2) Compared to the best existing models, the performance of the proposed method surpasses others while significantly reducing the number of parameters, proving the superiority of the proposed approach.
In addition to objective metrics, the detection results of two instances from the dataset are visualized in Fig. 4. The results show that the proposed MSFF-Net demonstrates better detection performance compared to YOLOv8.
Figure 4: Visualization of detection results for two instances from the Comparison detector, labeled as (a) and (b). In group (a), the baseline YOLOv8 detection missed an HSIL cell, resulting in a false negative. Additionally, background errors occurred, with two normal cells incorrectly detected as HSIL cells. The proposed MSFF-Net avoided both the background errors and the missed detection. In group (b), the baseline YOLOv8 detection resulted in two background errors, which were corrected using the proposed MSFF-Net
This study also uses heat map to visualize some of the outputs of YOLOv8 and MSFF-Net. The heat map for two instances from the dataset are shown in the Fig. 5. YOLOv8’s attention is relatively scattered, with some focus on the background. In contrast, MSFF-Net demonstrates strong attention to all abnormal cells. This is demonstrated in Fig. 5a and b, respectively. This suggests that in detecting cervical abnormal cells, MSFF-Net can better highlights the features of the abnormal cells.
Figure 5: Presents heat map visualizations for two examples. (a) and (b) show the visualization results of the first example using YOLOv8 and MSFF-Net, respectively. In (a), while YOLOv8 demonstrates good focus on abnormal cells, its accuracy is lower compared to MSFF-Net, and its attention covers a wider area. (c) and (d) show the second example’s detection results using YOLOv8 and MSFF-Net, respectively. In (c), issues such as dispersed attention lead to misdetection, while in (d), MSFF-Net resolves this problem, providing more precise localization for the two ASCH abnormal cells
To further validate the method’s effectiveness presented in this study, it is also applied to the DCCL dataset. The experimental results of the method proposed in this study compared to other model methods are shown in Table 3. The accuracy achieved using the MSFF-Net network is higher than that of other methods. However, the accuracy remains low, which is due to the incomplete labeling of the DCCL dataset limits the learnable features, making it more suited for semi-supervised learning.
Effects of Network Components: To assess the impact of the improvement strategies proposed in this study, Ablation experiments were performed on the baseline model using the Comparison Detector dataset to evaluate the effects of various methods in MSFF-Net. The comparison results are presented in Table 4.
Cross-Scale Pooling Model Experiments: By adding CSPM, we observed a 1.2% increase in mAP. From the generated heat map (Fig. 6), it can be seen that before adding CSPM (Fig. 6a), the model’s attention was more scattered, with some focus on background regions or irrelevant cells, making it difficult to accurately locate lesion cells. After incorporating CSPM (Fig. 6b), the model’s attention became significantly more concentrated on abnormal cells and their related regions, demonstrating the module’s enhancement in feature extraction and modeling cell interactions.
Figure 6: (a) Shows the heat map generated before adding CSPM, while (b) shows the heat map generated after adding CSPM
CSPM divides the feature map into two parts: one for simple feature extraction and the other for multi-scale pooling to capture both local and global information, combining detailed features with contextual information. Multi-scale pooling helps capture features of cells with varying sizes, while cross-cell context integration enhances the modeling of relationships between abnormal cells and surrounding normal cells, enabling more precise differentiation between target cells and background cells. The comparison in the heat map clearly shows that the CSPM module effectively addresses insufficient feature interaction between cells and reduces background interference, significantly improving the accuracy of lesion cell detection.
Multi-Scale Fusion Attention Experiments: The original YOLOv8 baseline model tends to overlook smaller cells in the dataset, such as ASCH and HSIL. To validate the effectiveness of MSFA, a comparison was conducted to evaluate detection performance before and after integrating MSFA. As shown in Table 5, after incorporating MSFA, the detection performance for smaller cell categories like ASCH and HSIL improved, while the detection performance for larger cell clusters like CAND also showed significant improvement. The MSFA module enhances the model’s ability to handle targets of varying sizes by utilizing multi-branch depthwise convolution to extract multi-scale features, where smaller kernels capture fine details for small targets, and larger kernels extract global information for larger targets. Additionally, Avgpool and Maxpool are employed to integrate both local and global contextual information, further strengthening the model’s perception of objects of different sizes. Finally, spatial and channel attention weighting highlights the target regions while suppressing background interference.
This study addresses the significant inter-class differences, minimal intra-class variation, and considerable size variability present in cervical cytology images by proposing MSFF-Net. This approach emulates the diagnostic behavior of pathologists, who reference the characteristics of surrounding cells when identifying abnormalities, thereby enhancing feature interactions among cells. The proposed method outperforms existing state-of-the-art deep learning techniques, offering a reliable and efficient tool for cervical cancer screening. In clinical practice, MSFF-Net demonstrates significant advantages, such as improving diagnostic consistency, reducing reliance on highly experienced pathologists, and accelerating the identification of abnormal cervical cells. It is particularly beneficial in resource-constrained clinical settings, where access to skilled pathologists and advanced diagnostic tools is limited, helping to enhance screening coverage and facilitate early detection. Furthermore, MSFF-Net’s ability to integrate local and global contextual features ensures robustness across varied clinical workflows and imaging conditions. However, its clinical implementation may face challenges, such as dependency on high-quality annotated datasets for training and variability in performance across different imaging systems or patient populations. Future work should focus on improving dataset diversity and fine-tuning the model to maintain consistent performance in diverse clinical environments, thereby maximizing its clinical utility.
Acknowledgement: The authors would like to express their gratitude for the valuable feedback and suggestions provided by all the anonymous reviewers and the editorial team.
Funding Statement: This study was funded by the China Chongqing Municipal Science and Technology Bureau, grant numbers 2024TIAD-CYKJCXX0121,2024NSCQ-LZX0135;Chongqing Municipal Commission of Housing and Urban-Rural Development, grant number CKZ2024-87;the Chongqing University of Technology graduate education high-quality development project, grant number gzlsz202401; the Chongqing University of Technology-Chongqing LINGLUE Technology Co., Ltd., Electronic Information (Artificial Intelligence) graduate joint training base; the Postgraduate Education and Teaching Reform Research Project in Chongqing, grant number yjg213116; and the Chongqing University of Technology-CISDI Chongqing Information Technology Co., Ltd., Computer Technology graduate joint training base.
Author Contributions: Chuanyun Xu: Methodology, Writing original draft, Writing review & editing, Funding acquisition. Die Hu: Writing original draft, Methodology, Investigation. Yang Zhang: Methodology, Writing review & editing, Funding acquisition, Project administration. Shuaiye Huang: Formal analysis, Visualization. Yisha Sun: Validation, Formal analysis. Gang Li: Writing review & editing, Supervision, Resources, Funding acquisition. All authors reviewed the results and approved the final version of the manuscript.
Availability of Data and Materials: All relevant data are within the paper. The data are available from the corresponding author on reasonable request.
Ethics Approval: Not applicable.
Conflicts of Interest: The authors declare no conflicts of interest to report regarding the present study.
References
1. Sung H, Ferlay J, Siegel RL, Laversanne M, Soerjomataram I, Jemal A, et al. Global cancer statistics 2020: GLOBOCAN estimates of incidence and mortality worldwide for 36 cancers in 185 countries. CA: A Cancer J Clinic. 2021;71(3):209–49. doi:10.3322/caac.21660. [Google Scholar] [PubMed] [CrossRef]
2. Landy R, Pesola F, Castañòn A, Sasieni P. Impact of cervical screening on cervical cancer mortality: estimation using stage-specific results from a nested case-control study. British J Cancer. 2016;115(9):1140–6. doi:10.1038/bjc.2016.290. [Google Scholar] [PubMed] [CrossRef]
3. de Bekker-Grob EW, de Kok IMCM, Bulten J, van Rosmalen J, Vedder JEM, Arbyn M, et al. Liquid-based cervical cytology using Thin Prep technology: weighing the pros and cons in a cost-effectiveness analysis. Cancer Cau Cont. 2012;23(8):1323–31. doi:10.1007/s10552-012-0011-1. [Google Scholar] [PubMed] [CrossRef]
4. Ma J, Yu J, Liu S, Chen L, Li X, Feng J, et al. PathSRGAN: multi-supervised super-resolution for cytopathological images using generative adversarial network. IEEE Transact Med Imag. 2020;39(9):2920–30. doi:10.1109/TMI.2020.2980839. [Google Scholar] [PubMed] [CrossRef]
5. Jia D, Li Z, Zhang C. A parametric optimization oriented, AFSA based random forest algorithm: application to the detection of cervical epithelial cells. IEEE Access. 2020;8:64891–905. doi:10.1109/ACCESS.2020.2984657. [Google Scholar] [CrossRef]
6. Lu Z, Carneiro G, Bradley AP, Ushizima D, Nosrati MS, Bianchi AGC, et al. Evaluation of three algorithms for the segmentation of overlapping cervical cells. IEEE J Biomed Health Inform. 2016;21(2):441–50. doi:10.1109/JBHI.2016.2519686. [Google Scholar] [PubMed] [CrossRef]
7. Zhang L, Lu L, Nogues I, Summers RM, Liu S, Yao J. DeepPap: deep convolutional networks for cervical cell classification. IEEE J Biomed Health Inform. 2017;21(6):1633–43. doi:10.1109/JBHI.2017.2705583. [Google Scholar] [PubMed] [CrossRef]
8. Ren S, He K, Girshick R, Sun J. Towards real-time object detection with region proposal networks. IEEE Transact Patt Anal Mach Intell. 2016;39(6):1137–49. doi:10.1109/TPAMI.2016.2577031. [Google Scholar] [CrossRef]
9. Lin TY, Goyal P, Girshick R, He K, Dollár P. Focal loss for dense object detection. In: 2017 IEEE International Conference on Computer Vision (ICCV); 2017 Oct 22–29; Venice, Italy: IEEE; p. 2999–3007. [Google Scholar]
10. Zhang C, Liu D, Wang L, Li Y, Chen X, Luo R, et al. DCCL: a benchmark for cervical cytology analysis. In: Machine Learning in Medical Imaging: 10th International Workshop, MLMI 2019, Held in Conjunction with MICCAI 2019; 2019 Oct 13; Shenzhen, China: Springer. p. 63–72. [Google Scholar]
11. Liang Y, Tang Z, Yan M, Chen J, Liu Q, Xiang Y. Comparison detector for cervical cell/clumps detection in the limited data scenario. Neurocomputing. 2021;437(3):195–205. doi:10.1016/j.neucom.2021.01.006. [Google Scholar] [CrossRef]
12. Lin H, Chen H, Wang X, Wang Q, Wang L, Heng PA. Dual-path network with synergistic grouping loss and evidence driven risk stratification for whole slide cervical image analysis. Med Image Anal. 2021;69(2):101955. doi:10.1016/j.media.2021.101955. [Google Scholar] [PubMed] [CrossRef]
13. Zhu X, Li X, Ong K, Zhang W, Li W, Li L, et al. Hybrid AI-assistive diagnostic model permits rapid TBS classification of cervical liquid-based thin-layer cell smears. Nat Commun. 2021;12(1):3541. doi:10.1038/s41467-021-23913-3. [Google Scholar] [PubMed] [CrossRef]
14. Nasir MU, Khalil OK, Ateeq K, Almogadwy BS, Khan MA, Adnan KM. Cervical cancer prediction empowered with federated machine learning. Comput Mater Contin. 2024;79(1):963–81. doi:10.32604/cmc.2024.047874. [Google Scholar] [CrossRef]
15. Xiang Y, Sun W, Pan C, Yan M, Yin Z, Liang Y. A novel automation-assisted cervical cancer reading method based on convolutional neural network. Biocybernet Biomed Eng. 2020;40(2):611–23. doi:10.1016/j.bbe.2020.01.016. [Google Scholar] [CrossRef]
16. Zahid Hasan Ontor M, Mamun Ali M, Ahmed K, Bui FM, Ahmed Al-Zahrani F, Hasan Mahmud SM, et al. Early-stage cervical cancerous cell detection from cervix images using YOLOv5. Comput Mater Contin. 2023;74(2):3727–41. doi:10.32604/cmc.2023.032794. [Google Scholar] [CrossRef]
17. Jin Y, Ma J, Lian Y, Wang F, Wu T, Hu H, et al. Cervical cytology screening using the fused deep learning architecture with attention mechanisms. Appl Soft Comput. 2024;166:112202. doi:10.1016/j.asoc.2024.112202. [Google Scholar] [CrossRef]
18. Liang Y, Pan C, Sun W, Liu Q, Du Y. Global context-aware cervical cell detection with soft scale anchor matching. Comput Methods Programs Biomed. 2021;204:106061. doi:10.1016/j.cmpb.2021.106061. [Google Scholar] [PubMed] [CrossRef]
19. Liang Y, Feng S, Liu Q, Kuang H, Liu J, Liao L, et al. Exploring contextual relationships for cervical abnormal cell detection. IEEE J Biomed Health Inform. 2023;27(8):4086–97. doi:10.1109/JBHI.2023.3276919. [Google Scholar] [PubMed] [CrossRef]
20. Cao L, Yang J, Rong Z, Li L, Xia B, You C, et al. A novel attention-guided convolutional network for the detection of abnormal cervical cells in cervical cancer screening. Med Image Anal. 2021;73:102197. doi:10.1016/j.media.2021.102197. [Google Scholar] [PubMed] [CrossRef]
21. Duan Z, Xu C, Li Z, Feng B, Nie C. FMA-Net: fusion of multi-scale attention for grading cervical precancerous lesions. Mathematics. 2024;12(7):958. doi:10.3390/math12070958. [Google Scholar] [CrossRef]
22. Khan A, Han S, Ilyas N, Lee YM, Lee B. CervixFormer: a Multi-scale swin transformer-Based cervical pap-Smear WSI classification framework. Comput Methods Programs Biomed. 2023;240:107718. doi:10.1016/j.cmpb.2023.107718. [Google Scholar] [PubMed] [CrossRef]
23. Liu C, Zhang S, Hu M, Song Q. Object detection in remote sensing images based on adaptive multi-scale feature fusion method. Remote Sens. 2024;16(5):907. doi:10.3390/rs16050907. [Google Scholar] [CrossRef]
24. Wang CY, Mark Liao HY, Wu YH, Chen PY, Hsieh JW, Yeh IH. CSPNet: a new backbone that can enhance learning capability of CNN. In: Proceedings of the IEEE/CVF Conference on Computer Vision and Pattern Recognition Workshops; 2020; Seattle, WA, USA. p. 390–1. [Google Scholar]
25. Guo Y, Li Y, Wang L, Rosing T. Depthwise convolution is all you need for learning multiple visual domains. Proc AAAI Conf Artif Intell. 2019;33(1):8368–75. doi:10.1609/aaai.v33i01.33018368. [Google Scholar] [CrossRef]
26. Guo M, Lu C, Hou Q, Liu Z, Cheng M, Segnext Hu S. Rethinking convolutional attention design for semantic segmentation. Adv Neural Inform Process Syst. 2022;35:1140–56. [Google Scholar]
27. Woo S, Park J, Lee JY, Kweon IS. Cbam: convolutional block attention module. In: Proceedings of the European Conference on Computer Vision (ECCV); 2018; Springer. p. 3–19. [Google Scholar]
28. Zheng Z, Wang P, Liu W, Li J, Ye R, Ren D. Distance-IoU loss: faster and better learning for bounding box regression. Proc AAAI Conf Artif Intell. 2020;34(7):12993–3000. doi:10.1609/aaai.v34i07.6999. [Google Scholar] [CrossRef]
29. Zhang H, Xu C, Zhang S. Inner-IoU: more effective intersection over union loss with auxiliary bounding box. arXiv:231102877. 2023. [Google Scholar]
30. Xu C, Li M, Li G, Zhang Y, Sun C, Bai N. Cervical cell/clumps detection in cytology images using transfer learning. Diagnostics. 2022;12(10):2477. doi:10.3390/diagnostics12102477. [Google Scholar] [PubMed] [CrossRef]
31. Peng Z, Hu R, Wang F, Fan H, Eng YW, Li Z, et al. Deep adaptively feature extracting network for cervical squamous lesion cell detection. In: International Conference on Machine Learning for Cyber Security; 2022; Springer. p. 238–53. [Google Scholar]
32. Wang A, Chen H, Liu L, Chen K, Lin Z, Han J, et al. YOLOv10: real-time end-to-end object detection. arXiv:240514458. 2024. [Google Scholar]
Cite This Article
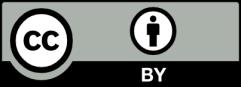
This work is licensed under a Creative Commons Attribution 4.0 International License , which permits unrestricted use, distribution, and reproduction in any medium, provided the original work is properly cited.