Open Access
ARTICLE
A Neural Network-Driven Method for State of Charge Estimation Using Dynamic AC Impedance in Lithium-Ion Batteries
1 Graduate Institute of A.I. Cross-Disciplinary Tech, National Taiwan University of Science and Technology, Taipei, 106335, Taiwan
2 Department of Electrical Engineering, National Changhua University of Education, Changhua, 50074, Taiwan
3 Department of Electrical Engineering, National Yunlin University of Science and Technology, Yunlin, 640301, Taiwan
4 Department of Electrical Engineering, National Taiwan University of Science and Technology, Taipei, 106335, Taiwan
* Corresponding Author: Guan-Jhu Chen. Email:
Computers, Materials & Continua 2025, 83(1), 823-844. https://doi.org/10.32604/cmc.2025.061498
Received 26 November 2024; Accepted 16 January 2025; Issue published 26 March 2025
Abstract
As lithium-ion batteries become increasingly prevalent in electric scooters, vehicles, mobile devices, and energy storage systems, accurate estimation of remaining battery capacity is crucial for optimizing system performance and reliability. Unlike traditional methods that rely on static alternating internal resistance (SAIR) measurements in an open-circuit state, this study presents a real-time state of charge (SOC) estimation method combining dynamic alternating internal resistance (DAIR) with artificial neural networks (ANN). The system simultaneously measures electrochemical impedance |Z| at various frequencies, discharge C-rate, and battery surface temperature during the discharge process, using these parameters for ANN training. The ANN, leveraging its superior nonlinear system modeling capabilities, effectively captures the complex nonlinear relationships between AC impedance and SOC through iterative training. Compared to other machine learning approaches, the proposed ANN features a simpler architecture and lower computational overhead, making it more suitable for integration into battery management system (BMS) microcontrollers. In tests conducted with Samsung batteries using lithium cobalt oxide cathode material, the method achieved an overall average error of merely 0.42% in self-validation, with mean absolute errors (MAE) for individual SOCs not exceeding 1%. Secondary validation demonstrated an overall average error of 1.24%, with MAE for individual SOCs below 2.5%. This integrated DAIR-ANN approach not only provides enhanced estimation accuracy but also simplifies computational requirements, offering a more effective solution for battery management in practical applications.Keywords
Cite This Article
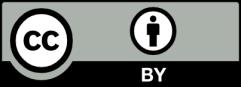
This work is licensed under a Creative Commons Attribution 4.0 International License , which permits unrestricted use, distribution, and reproduction in any medium, provided the original work is properly cited.