Open Access
ARTICLE
AI-Based Tire Pressure Detection Using an Enhanced Deep Learning Architecture
Graduate Institute of Vehicle Engineering, National Changhua University of Education, Changhua, 50007, Taiwan
* Corresponding Author: Shih-Lin Lin. Email:
Computers, Materials & Continua 2025, 83(1), 537-557. https://doi.org/10.32604/cmc.2025.061379
Received 23 November 2024; Accepted 11 February 2025; Issue published 26 March 2025
Abstract
Tires are integral to vehicular systems, directly influencing both safety and overall performance. Traditional tire pressure inspection methods—such as manual or gauge-based approaches—are often time-consuming, prone to inconsistency, and lack the flexibility needed to meet diverse operational demands. In this research, we introduce an AI-driven tire pressure detection system that leverages an enhanced GoogLeNet architecture incorporating a novel Softplus-LReLU activation function. By combining the smooth, non-saturating characteristics of Softplus with a linear adjustment term, this activation function improves computational efficiency and helps stabilize network gradients, thereby mitigating issues such as gradient vanishing and neuron death. Our enhanced GoogLeNet algorithm was validated on a dedicated tire pressure image database comprising three categories-low pressure, normal pressure, and undetected. Experimental results revealed a classification accuracy of 98.518% within 11 min and 56 s of total processing time, substantially surpassing the original GoogLeNet’s 95.1852% and ResNet18’s 92.7778%. This performance gain is attributed to superior feature extraction within the Inception modules and the robust integration of our novel activation function, leading to improved detection reliability and faster inference. Beyond accuracy and speed, the proposed system offers significant benefits for real-time monitoring and vehicle safety by providing timely and precise tire pressure assessments. The automation facilitated by our AI-based method addresses the limitations of manual inspection, delivering consistent, high-quality results that can be easily scaled or customized for various vehicular platforms. Overall, this work establishes a solid foundation for advanced tire pressure monitoring systems and opens avenues for further exploration in AI-assisted vehicle maintenance, contributing to safer and more efficient automotive operations.Keywords
Cite This Article
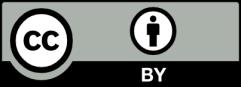
This work is licensed under a Creative Commons Attribution 4.0 International License , which permits unrestricted use, distribution, and reproduction in any medium, provided the original work is properly cited.