Open Access
ARTICLE
Defending Federated Learning System from Poisoning Attacks via Efficient Unlearning
School of Computer and Communication Engineering, Changsha University of Science and Technology, Changsha, 410114, China
* Corresponding Author: Ke Gu. Email:
Computers, Materials & Continua 2025, 83(1), 239-258. https://doi.org/10.32604/cmc.2025.061377
Received 23 November 2024; Accepted 14 February 2025; Issue published 26 March 2025
Abstract
Large-scale neural networks-based federated learning (FL) has gained public recognition for its effective capabilities in distributed training. Nonetheless, the open system architecture inherent to federated learning systems raises concerns regarding their vulnerability to potential attacks. Poisoning attacks turn into a major menace to federated learning on account of their concealed property and potent destructive force. By altering the local model during routine machine learning training, attackers can easily contaminate the global model. Traditional detection and aggregation solutions mitigate certain threats, but they are still insufficient to completely eliminate the influence generated by attackers. Therefore, federated unlearning that can remove unreliable models while maintaining the accuracy of the global model has become a solution. Unfortunately some existing federated unlearning approaches are rather difficult to be applied in large neural network models because of their high computational expenses. Hence, we propose SlideFU, an efficient anti-poisoning attack federated unlearning framework. The primary concept of SlideFU is to employ sliding window to construct the training process, where all operations are confined within the window. We design a malicious detection scheme based on principal component analysis (PCA), which calculates the trust factors between compressed models in a low-cost way to eliminate unreliable models. After confirming that the global model is under attack, the system activates the federated unlearning process, calibrates the gradients based on the updated direction of the calibration gradients. Experiments on two public datasets demonstrate that our scheme can recover a robust model with extremely high efficiency.Keywords
Cite This Article
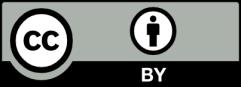
This work is licensed under a Creative Commons Attribution 4.0 International License , which permits unrestricted use, distribution, and reproduction in any medium, provided the original work is properly cited.