Open Access
ARTICLE
Lightweight Classroom Student Action Recognition Method Based on Spatiotemporal Multimodal Feature Fusion
1 Key Laboratory of Education Informatization for Nationalities, Ministry of Education, Yunnan Normal University, Kunming, 650500, China
2 Yunnan Key Laboratory of Smart Education, Yunnan Normal University, Kunming, 650500, China
* Corresponding Author: Jianhou Gan. Email:
(This article belongs to the Special Issue: Big Data and Artificial Intelligence in Control and Information System)
Computers, Materials & Continua 2025, 83(1), 1101-1116. https://doi.org/10.32604/cmc.2025.061376
Received 23 November 2024; Accepted 13 January 2025; Issue published 26 March 2025
Abstract
The task of student action recognition in the classroom is to precisely capture and analyze the actions of students in classroom videos, providing a foundation for realizing intelligent and accurate teaching. However, the complex nature of the classroom environment has added challenges and difficulties in the process of student action recognition. In this research article, with regard to the circumstances where students are prone to be occluded and classroom computing resources are restricted in real classroom scenarios, a lightweight multi-modal fusion action recognition approach is put forward. This proposed method is capable of enhancing the accuracy of student action recognition while concurrently diminishing the number of parameters of the model and the Computation Amount, thereby achieving a more efficient and accurate recognition performance. In the feature extraction stage, this method fuses the keypoint heatmap with the RGB (Red-Green-Blue color model) image. In order to fully utilize the unique information of different modalities for feature complementarity, a Feature Fusion Module (FFE) is introduced. The FFE encodes and fuses the unique features of the two modalities during the feature extraction process. This fusion strategy not only achieves fusion and complementarity between modalities, but also improves the overall model performance. Furthermore, to reduce the computational load and parameter scale of the model, we use keypoint information to crop RGB images. At the same time, the first three networks of the lightweight feature extraction network X3D are used to extract dual-branch features. These methods significantly reduce the computational load and parameter scale. The number of parameters of the model is 1.40 million, and the computation amount is 5.04 billion floating-point operations per second (GFLOPs), achieving an efficient lightweight design. In the Student Classroom Action Dataset (SCAD), the accuracy of the model is 88.36%. In NTU RGB+D 60 (Nanyang Technological University Red-Green-Blue-Depth dataset with 60 categories), the accuracies on X-Sub (The people in the training set are different from those in the test set) and X-View (The perspectives of the training set and the test set are different) are 95.76% and 98.82%, respectively. On the NTU RGB+D 120 dataset (Nanyang Technological University Red-Green-Blue-Depth dataset with 120 categories), the accuracies on X-Sub and X-Set (the perspectives of the training set and the test set are different) are 91.97% and 93.45%, respectively. The model has achieved a balance in terms of accuracy, computation amount, and the number of parameters.Keywords
Cite This Article
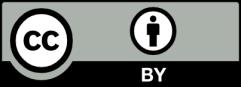
This work is licensed under a Creative Commons Attribution 4.0 International License , which permits unrestricted use, distribution, and reproduction in any medium, provided the original work is properly cited.