Open Access
ARTICLE
MVLA-Net: A Multi-View Lesion Attention Network for Advanced Diagnosis and Grading of Diabetic Retinopathy
1 Artificial Intelligence and Data Analytics (AIDA) Lab, College of Computer & Information Sciences, Prince Sultan University, Riyadh, 11586, Saudi Arabia
2 Faculty of Information Sciences, University of Education, Vehari Campus, Vehari, 61100, Pakistan
3 Department of Mathematical Sciences, College of Science, Princess Nourah bint Abdulrahman University, Riyadh, 84428, Saudi Arabia
4 Shifa College of Medicine, Shifa Tameer-E-Millat University, Islamabad, 44000, Pakistan
5 Center of Excellence in Cyber Security (CYBEX), Prince Sultan University, Riyadh, 11586, Saudi Arabia
* Corresponding Authors: Faten S. Alamri. Email: ,
(This article belongs to the Special Issue: Expanding Horizons in Ophthalmic Diagnostics: A Multidisciplinary AI Approach)
Computers, Materials & Continua 2025, 83(1), 1173-1193. https://doi.org/10.32604/cmc.2025.061150
Received 18 November 2024; Accepted 18 February 2025; Issue published 26 March 2025
Abstract
Innovation in learning algorithms has made retinal vessel segmentation and automatic grading techniques crucial for clinical diagnosis and prevention of diabetic retinopathy. The traditional methods struggle with accuracy and reliability due to multi-scale variations in retinal blood vessels and the complex pathological relationship in fundus images associated with diabetic retinopathy. While the single-modal diabetic retinopathy grading network addresses class imbalance challenges and lesion representation in fundus image data, dual-modal diabetic retinopathy grading methods offer superior performance. However, the scarcity of dual-modal data and the lack of effective feature fusion methods limit their potential due to multi-scale variations. This paper addresses these issues by focusing on multi-scale retinal vessel segmentation, dual feature fusion, data augmentation, and attention-based grading. The proposed model aims to improve comprehensive segmentation for retinal images with varying vessel thicknesses. It employs a dual-branch parallel architecture that integrates a transformer encoder with a convolutional neural network encoder to extract local and global information for synergistic saliency learning. Besides that, the model uses residual structures and attention modules to extract critical lesions, enhancing the accuracy and reliability of diabetic retinopathy grading. To evaluate the efficacy of the proposed approach, this study compared it with other pre-trained publicly open models, ResNet152V2, ConvNext, Efficient Net, DenseNet, and Swin Transform, with the same developmental parameters. All models achieved approximately 85% accuracy with the same image preparation method. However, the proposed approach outperforms and optimizes existing models by achieving an accuracy of 99.17%, 99.04%, and 99.24%, on Kaggle APTOS19, IDRiD, and EyePACS datasets, respectively. These results support the model’s utility in helping ophthalmologists diagnose diabetic retinopathy more rapidly and accurately.Keywords
Cite This Article
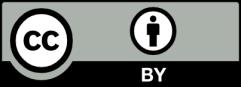
This work is licensed under a Creative Commons Attribution 4.0 International License , which permits unrestricted use, distribution, and reproduction in any medium, provided the original work is properly cited.