Open Access
ARTICLE
An Explainable Autoencoder-Based Feature Extraction Combined with CNN-LSTM-PSO Model for Improved Predictive Maintenance
eCornell, Division of Online Learning, Cornell University, Ithaca, NY 14850, USA
* Corresponding Author: Ishaani Priyadarshini. Email:
(This article belongs to the Special Issue: Next-Generation AI for Ethical and Explainable Decision-Making in Critical Systems)
Computers, Materials & Continua 2025, 83(1), 635-659. https://doi.org/10.32604/cmc.2025.061062
Received 16 November 2024; Accepted 10 February 2025; Issue published 26 March 2025
Abstract
Predictive maintenance plays a crucial role in preventing equipment failures and minimizing operational downtime in modern industries. However, traditional predictive maintenance methods often face challenges in adapting to diverse industrial environments and ensuring the transparency and fairness of their predictions. This paper presents a novel predictive maintenance framework that integrates deep learning and optimization techniques while addressing key ethical considerations, such as transparency, fairness, and explainability, in artificial intelligence driven decision-making. The framework employs an Autoencoder for feature reduction, a Convolutional Neural Network for pattern recognition, and a Long Short-Term Memory network for temporal analysis. To enhance transparency, the decision-making process of the framework is made interpretable, allowing stakeholders to understand and trust the model’s predictions. Additionally, Particle Swarm Optimization is used to refine hyperparameters for optimal performance and mitigate potential biases in the model. Experiments are conducted on multiple datasets from different industrial scenarios, with performance validated using accuracy, precision, recall, F1-score, and training time metrics. The results demonstrate an impressive accuracy of up to 99.92% and 99.45% across different datasets, highlighting the framework’s effectiveness in enhancing predictive maintenance strategies. Furthermore, the model’s explainability ensures that the decisions can be audited for fairness and accountability, aligning with ethical standards for critical systems. By addressing transparency and reducing potential biases, this framework contributes to the responsible and trustworthy deployment of artificial intelligence in industrial environments, particularly in safety-critical applications. The results underscore its potential for wide application across various industrial contexts, enhancing both performance and ethical decision-making.Keywords
Cite This Article
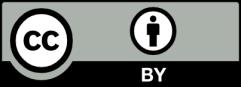
This work is licensed under a Creative Commons Attribution 4.0 International License , which permits unrestricted use, distribution, and reproduction in any medium, provided the original work is properly cited.