Open Access
ARTICLE
CE-CDNet: A Transformer-Based Channel Optimization Approach for Change Detection in Remote Sensing
1 School of Computer Science and Technology, Zhengzhou University of Light Industry, Zhengzhou, 450002, China
2 Department of Computing, Xi’an Jiaotong-Liverpool University, Suzhou, 215123, China
* Corresponding Author: Wei Wang. Email:
Computers, Materials & Continua 2025, 83(1), 803-822. https://doi.org/10.32604/cmc.2025.060966
Received 13 November 2024; Accepted 06 January 2025; Issue published 26 March 2025
Abstract
In recent years, convolutional neural networks (CNN) and Transformer architectures have made significant progress in the field of remote sensing (RS) change detection (CD). Most of the existing methods directly stack multiple layers of Transformer blocks, which achieves considerable improvement in capturing variations, but at a rather high computational cost. We propose a channel-Efficient Change Detection Network (CE-CDNet) to address the problems of high computational cost and imbalanced detection accuracy in remote sensing building change detection. The adaptive multi-scale feature fusion module (CAMSF) and lightweight Transformer decoder (LTD) are introduced to improve the change detection effect. The CAMSF module can adaptively fuse multi-scale features to improve the model’s ability to detect building changes in complex scenes. In addition, the LTD module reduces computational costs and maintains high detection accuracy through an optimized self-attention mechanism and dimensionality reduction operation. Experimental test results on three commonly used remote sensing building change detection data sets show that CE-CDNet can reduce a certain amount of computational overhead while maintaining detection accuracy comparable to existing mainstream models, showing good performance advantages.Keywords
Cite This Article
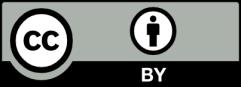
This work is licensed under a Creative Commons Attribution 4.0 International License , which permits unrestricted use, distribution, and reproduction in any medium, provided the original work is properly cited.