Open Access
ARTICLE
A Novel Stacked Network Method for Enhancing the Performance of Side-Channel Attacks
1 College of Computer Science and Technology, Hengyang Normal University, Hengyang, 421002, China
2 Hunan Provincial Key Laboratory of Intelligent Information Processing and Application, Hengyang, Normal University, Hengyang, 421002, China
* Corresponding Author: Lang Li. Email:
Computers, Materials & Continua 2025, 83(1), 1001-1022. https://doi.org/10.32604/cmc.2025.060925
Received 12 November 2024; Accepted 06 January 2025; Issue published 26 March 2025
Abstract
The adoption of deep learning-based side-channel analysis (DL-SCA) is crucial for leak detection in secure products. Many previous studies have applied this method to break targets protected with countermeasures. Despite the increasing number of studies, the problem of model overfitting. Recent research mainly focuses on exploring hyperparameters and network architectures, while offering limited insights into the effects of external factors on side-channel attacks, such as the number and type of models. This paper proposes a Side-channel Analysis method based on a Stacking ensemble, called Stacking-SCA. In our method, multiple models are deeply integrated. Through the extended application of base models and the meta-model, Stacking-SCA effectively improves the output class probabilities of the model, leading to better generalization. Furthermore, this method shows that the attack performance is sensitive to changes in the number of models. Next, five independent subsets are extracted from the original ASCAD database as multi-segment datasets, which are mutually independent. This method shows how these subsets are used as inputs for Stacking-SCA to enhance its attack convergence. The experimental results show that Stacking-SCA outperforms the current state-of-the-art results on several considered datasets, significantly reducing the number of attack traces required to achieve a guessing entropy of 1. Additionally, different hyperparameter sizes are adjusted to further validate the robustness of the method.Keywords
Cite This Article
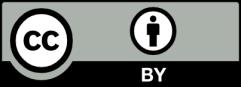
This work is licensed under a Creative Commons Attribution 4.0 International License , which permits unrestricted use, distribution, and reproduction in any medium, provided the original work is properly cited.