Open Access
ARTICLE
A Generative Image Steganography Based on Disentangled Attribute Feature Transformation and Invertible Mapping Rule
1 School of Computer Science, Nanjing University of Information Science and Technology, Nanjing, 210044, China
2 Engineering Research Center of Digital Forensics, Nanjing University of Information Science and Technology, Ministry of Education, Nanjing, 210044, China
* Corresponding Author: Xiang Zhang. Email:
(This article belongs to the Special Issue: Multimedia Security in Deep Learning)
Computers, Materials & Continua 2025, 83(1), 1149-1171. https://doi.org/10.32604/cmc.2025.060876
Received 12 November 2024; Accepted 24 January 2025; Issue published 26 March 2025
Abstract
Generative image steganography is a technique that directly generates stego images from secret information. Unlike traditional methods, it theoretically resists steganalysis because there is no cover image. Currently, the existing generative image steganography methods generally have good steganography performance, but there is still potential room for enhancing both the quality of stego images and the accuracy of secret information extraction. Therefore, this paper proposes a generative image steganography algorithm based on attribute feature transformation and invertible mapping rule. Firstly, the reference image is disentangled by a content and an attribute encoder to obtain content features and attribute features, respectively. Then, a mean mapping rule is introduced to map the binary secret information into a noise vector, conforming to the distribution of attribute features. This noise vector is input into the generator to produce the attribute transformed stego image with the content feature of the reference image. Additionally, we design an adversarial loss, a reconstruction loss, and an image diversity loss to train the proposed model. Experimental results demonstrate that the stego images generated by the proposed method are of high quality, with an average extraction accuracy of 99.4% for the hidden information. Furthermore, since the stego image has a uniform distribution similar to the attribute-transformed image without secret information, it effectively resists both subjective and objective steganalysis.Keywords
Cite This Article
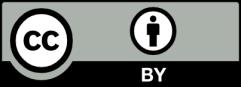
This work is licensed under a Creative Commons Attribution 4.0 International License , which permits unrestricted use, distribution, and reproduction in any medium, provided the original work is properly cited.