Open Access
ARTICLE
A Method for Fast Feature Selection Utilizing Cross-Similarity within the Context of Fuzzy Relations
1 School of Big Data and Artificial Intelligence, Chizhou University, Chizhou, 247000, China
2 Anhui Education Big Data Intelligent Perception and Application Engineering Research Center, Anhui Provincial Joint Construction Key Laboratory of Intelligent Education Equipment and Technology, Chizhou, 247000, China
* Corresponding Author: Qinli Zhang. Email:
(This article belongs to the Special Issue: Advanced Algorithms for Feature Selection in Machine Learning)
Computers, Materials & Continua 2025, 83(1), 1195-1218. https://doi.org/10.32604/cmc.2025.060833
Received 11 November 2024; Accepted 28 January 2025; Issue published 26 March 2025
Abstract
Feature selection methods rooted in rough sets confront two notable limitations: their high computational complexity and sensitivity to noise, rendering them impractical for managing large-scale and noisy datasets. The primary issue stems from these methods’ undue reliance on all samples. To overcome these challenges, we introduce the concept of cross-similarity grounded in a robust fuzzy relation and design a rapid and robust feature selection algorithm. Firstly, we construct a robust fuzzy relation by introducing a truncation parameter. Then, based on this fuzzy relation, we propose the concept of cross-similarity, which emphasizes the sample-to-sample similarity relations that uniquely determine feature importance, rather than considering all such relations equally. After studying the manifestations and properties of cross-similarity across different fuzzy granularities, we propose a forward greedy feature selection algorithm that leverages cross-similarity as the foundation for information measurement. This algorithm significantly reduces the time complexity from O(m2n2) to O(mn2). Experimental findings reveal that the average runtime of five state-of-the-art comparison algorithms is roughly 3.7 times longer than our algorithm, while our algorithm achieves an average accuracy that surpasses those of the five comparison algorithms by approximately 3.52%. This underscores the effectiveness of our approach. This paper paves the way for applying feature selection algorithms grounded in fuzzy rough sets to large-scale gene datasets.Keywords
Cite This Article
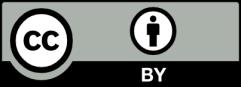
This work is licensed under a Creative Commons Attribution 4.0 International License , which permits unrestricted use, distribution, and reproduction in any medium, provided the original work is properly cited.