Open Access
ARTICLE
LogDA: Dual Attention-Based Log Anomaly Detection Addressing Data Imbalance
School of Information Science and Technology, Hainan Normal University, Haikou, 571158, China
* Corresponding Author: Haiyan Fu. Email:
(This article belongs to the Special Issue: Applications of Artificial Intelligence for Information Security)
Computers, Materials & Continua 2025, 83(1), 1291-1306. https://doi.org/10.32604/cmc.2025.060740
Received 08 November 2024; Accepted 29 January 2025; Issue published 26 March 2025
Abstract
As computer data grows exponentially, detecting anomalies within system logs has become increasingly important. Current research on log anomaly detection largely depends on log templates derived from log parsing. Word embedding is utilized to extract information from these templates. However, this method neglects a portion of the content within the logs and confronts the challenge of data imbalance among various log template types after parsing. Currently, specialized research on data imbalance across log template categories remains scarce. A dual-attention-based log anomaly detection model (LogDA), which leveraged data imbalance, was proposed to address these issues in the work. The LogDA model initially utilized a pre-trained model to extract semantic embedding from log templates. Besides, the similarity between embedding was calculated to discern the relationships among the various templates. Then, a Transformer model with a dual-attention mechanism was constructed to capture positional information and global dependencies. Compared to multiple baseline experiments across three public datasets, the proposed approach could improve precision, recall, and F1 scores.Keywords
Cite This Article
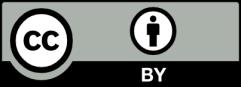
This work is licensed under a Creative Commons Attribution 4.0 International License , which permits unrestricted use, distribution, and reproduction in any medium, provided the original work is properly cited.