Open Access
ARTICLE
Application of Deep-Learning Potential in Simulating the Structural and Physical Characteristics of Platinum
1 Faculty of Materials Science and Engineering, Kunming University of Science and Technology, Kunming, 650093, China
2 Yunnan Key Laboratory of Integrated Computational Materials Engineering for Advanced Light Metals, Kunming, 650093, China
* Corresponding Authors: Ju Rong. Email: ; Yudong Sui. Email:
(This article belongs to the Special Issue: Advances in Computational Materials Science: Focusing on Atomic-Scale Simulations and AI-Driven Innovations)
Computers, Materials & Continua 2025, 83(1), 685-700. https://doi.org/10.32604/cmc.2025.060713
Received 07 November 2024; Accepted 03 January 2025; Issue published 26 March 2025
Abstract
The deep potential (DP) is an innovative approach based on deep learning that uses ab initio calculation data derived from density functional theory (DFT), to create high-accuracy potential functions for various materials. Platinum (Pt) is a rare metal with significant potential in energy and catalytic applications, However, there are challenges in accurately capturing its physical properties due to high experimental costs and the limitations of traditional empirical methods. This study employs deep learning methods to construct high-precision potential models for single-element systems of Pt and validates their predictive performance in complex environments. The newly developed DP is highly consistent with DFT results in predicting the stable phases, lattice constants, surface energies, and phonons dispersion relations of Pt, demonstrating outstanding quantum-level accuracy. Additionally, the complex phase transitions and domain formations of Pt are extensively and quantitatively analyzed. Molecular dynamic simulations utilizing the DP approach show that Pt’s face-centered cubic (FCC) structure undergoes a phase transition from solid to liquid at its melting point of 1986 K, this is in close agreement with the experimental value of 2041.5 K. Increased temperature enhances the diffusion of Pt atoms, with a self-diffusion coefficient of 1.17 × 10−11 m2/s at the melting point, comparable to that of other FCC metals. This result can be utilized for the precise analysis of the fundamental properties of the rare metal Pt at the microscopic scale, and it facilitates the development of binary or multi-component deep potential models that include Pt.Keywords
Supplementary Material
Supplementary Material FileCite This Article
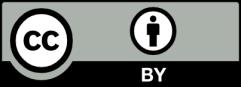
This work is licensed under a Creative Commons Attribution 4.0 International License , which permits unrestricted use, distribution, and reproduction in any medium, provided the original work is properly cited.