Open Access
ARTICLE
FedCPS: A Dual Optimization Model for Federated Learning Based on Clustering and Personalization Strategy
1 School of Cyber Security and Computer, Hebei University, Baoding, 071000, China
2 School of Management, Hebei University, Baoding, 071000, China
3 Information Technology Center, Hebei University, Baoding, 071000, China
* Corresponding Author: Yifan Liu. Email:
Computers, Materials & Continua 2025, 83(1), 357-380. https://doi.org/10.32604/cmc.2025.060709
Received 07 November 2024; Accepted 21 January 2025; Issue published 26 March 2025
Abstract
Federated learning is a machine learning framework designed to protect privacy by keeping training data on clients’ devices without sharing private data. It trains a global model through collaboration between clients and the server. However, the presence of data heterogeneity can lead to inefficient model training and even reduce the final model’s accuracy and generalization capability. Meanwhile, data scarcity can result in suboptimal cluster distributions for few-shot clients in centralized clustering tasks, and standalone personalization tasks may cause severe overfitting issues. To address these limitations, we introduce a federated learning dual optimization model based on clustering and personalization strategy (FedCPS). FedCPS adopts a decentralized approach, where clients identify their cluster membership locally without relying on a centralized clustering algorithm. Building on this, FedCPS introduces personalized training tasks locally, adding a regularization term to control deviations between local and cluster models. This improves the generalization ability of the final model while mitigating overfitting. The use of weight-sharing techniques also reduces the computational cost of central machines. Experimental results on MNIST, FMNIST, CIFAR10, and CIFAR100 datasets demonstrate that our method achieves better personalization effects compared to other personalized federated learning methods, with an average test accuracy improvement of 0.81%–2.96%. Meanwhile, we adjusted the proportion of few-shot clients to evaluate the impact on accuracy across different methods. The experiments show that FedCPS reduces accuracy by only 0.2%–3.7%, compared to 2.1%–10% for existing methods. Our method demonstrates its advantages across diverse data environments.Keywords
Cite This Article
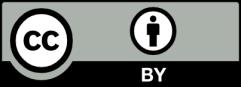
This work is licensed under a Creative Commons Attribution 4.0 International License , which permits unrestricted use, distribution, and reproduction in any medium, provided the original work is properly cited.