Open Access
ARTICLE
FHGraph: A Novel Framework for Fake News Detection Using Graph Contrastive Learning and LLM
1 School of Cyber Science and Engineering, Southeast University, Nanjing, 210096, China
2 Key Laboratory of Computer Network and Information Integration (Southeast University), Ministry of Education, Nanjing, 210096, China
* Corresponding Author: Sanfeng Zhang. Email:
Computers, Materials & Continua 2025, 83(1), 309-333. https://doi.org/10.32604/cmc.2025.060455
Received 01 November 2024; Accepted 08 January 2025; Issue published 26 March 2025
Abstract
Social media has significantly accelerated the rapid dissemination of information, but it also boosts propagation of fake news, posing serious challenges to public awareness and social stability. In real-world contexts, the volume of trustable information far exceeds that of rumors, resulting in a class imbalance that leads models to prioritize the majority class during training. This focus diminishes the model’s ability to recognize minority class samples. Furthermore, models may experience overfitting when encountering these minority samples, further compromising their generalization capabilities. Unlike node-level classification tasks, fake news detection in social networks operates on graph-level samples, where traditional interpolation and oversampling methods struggle to effectively generate high-quality graph-level samples. This challenge complicates the identification of new instances of false information. To address this issue, this paper introduces the FHGraph (Fake News Hunting Graph) framework, which employs a generative data augmentation approach and a latent diffusion model to create graph structures that align with news communication patterns. Using the few-sample learning capabilities of large language models (LLMs), the framework generates diverse texts for minority class nodes. FHGraph comprises a hierarchical multiview graph contrastive learning module, in which two horizontal views and three vertical levels are utilized for self-supervised learning, resulting in more optimized representations. Experimental results show that FHGraph significantly outperforms state-of-the-art (SOTA) graph-level class imbalance methods and SOTA graph-level contrastive learning methods. Specifically, FHGraph has achieved a 2% increase in F1 Micro and a 2.5% increase in F1 Macro in the PHEME dataset, as well as a 3.5% improvement in F1 Micro and a 4.3% improvement in F1 Macro on RumorEval dataset.Keywords
Cite This Article
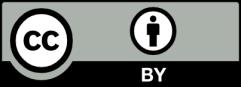
This work is licensed under a Creative Commons Attribution 4.0 International License , which permits unrestricted use, distribution, and reproduction in any medium, provided the original work is properly cited.