Open Access
ARTICLE
MMCSD: Multi-Modal Knowledge Graph Completion Based on Super-Resolution and Detailed Description Generation
National Digital Switching System Engineering & Technological R&D Center, Information Engineering University, Zhengzhou, 450001, China
* Corresponding Authors: Huansha Wang. Email: ; Ruiyang Huang. Email:
Computers, Materials & Continua 2025, 83(1), 761-783. https://doi.org/10.32604/cmc.2025.060395
Received 31 October 2024; Accepted 20 January 2025; Issue published 26 March 2025
Abstract
Multi-modal knowledge graph completion (MMKGC) aims to complete missing entities or relations in multi-modal knowledge graphs, thereby discovering more previously unknown triples. Due to the continuous growth of data and knowledge and the limitations of data sources, the visual knowledge within the knowledge graphs is generally of low quality, and some entities suffer from the issue of missing visual modality. Nevertheless, previous studies of MMKGC have primarily focused on how to facilitate modality interaction and fusion while neglecting the problems of low modality quality and modality missing. In this case, mainstream MMKGC models only use pre-trained visual encoders to extract features and transfer the semantic information to the joint embeddings through modal fusion, which inevitably suffers from problems such as error propagation and increased uncertainty. To address these problems, we propose a Multi-modal knowledge graph Completion model based on Super-resolution and Detailed Description Generation (MMCSD). Specifically, we leverage a pre-trained residual network to enhance the resolution and improve the quality of the visual modality. Moreover, we design multi-level visual semantic extraction and entity description generation, thereby further extracting entity semantics from structural triples and visual images. Meanwhile, we train a variational multi-modal auto-encoder and utilize a pre-trained multi-modal language model to complement the missing visual features. We conducted experiments on FB15K-237 and DB13K, and the results showed that MMCSD can effectively perform MMKGC and achieve state-of-the-art performance.Keywords
Cite This Article
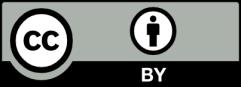
This work is licensed under a Creative Commons Attribution 4.0 International License , which permits unrestricted use, distribution, and reproduction in any medium, provided the original work is properly cited.