Open Access
ARTICLE
GACL-Net: Hybrid Deep Learning Framework for Accurate Motor Imagery Classification in Stroke Rehabilitation
1 State Key Laboratory of Multimodal Artificial Intelligence Systems, Institute of Automation, Chinese Academy of Sciences, Beijing, 100190, China
2 School of Computing, Gachon University, Seongnam, 13120, Republic of Korea
3 Computer Science Department, Faculty of Information Technology, The World Islamic Sciences and Education University, Amman, 11947, Jordan
4 Intelligent Systems Department, Faculty of Artificial Intelligence, Al-Balqa Applied University, Al-Salt, 19117, Jordan
5 IT Infrastructure Department, Qatrana Cement Company, Amman, 11821, Jordan
6 Division of Industrial and Logistics Engineering Technology, Faculty of Engineering and Technology, King Mongkut’s University of Technology North Bangkok, Rayong Campus, Rayong, 21120, Thailand
* Corresponding Author: Sangwoo Kang. Email:
Computers, Materials & Continua 2025, 83(1), 517-536. https://doi.org/10.32604/cmc.2025.060368
Received 30 October 2024; Accepted 01 February 2025; Issue published 26 March 2025
Abstract
Stroke is a leading cause of death and disability worldwide, significantly impairing motor and cognitive functions. Effective rehabilitation is often hindered by the heterogeneity of stroke lesions, variability in recovery patterns, and the complexity of electroencephalography (EEG) signals, which are often contaminated by artifacts. Accurate classification of motor imagery (MI) tasks, involving the mental simulation of movements, is crucial for assessing rehabilitation strategies but is challenged by overlapping neural signatures and patient-specific variability. To address these challenges, this study introduces a graph-attentive convolutional long short-term memory (LSTM) network (GACL-Net), a novel hybrid deep learning model designed to improve MI classification accuracy and robustness. GACL-Net incorporates multi-scale convolutional blocks for spatial feature extraction, attention fusion layers for adaptive feature prioritization, graph convolutional layers to model inter-channel dependencies, and bidirectional LSTM layers with attention to capture temporal dynamics. Evaluated on an open-source EEG dataset of 50 acute stroke patients performing left and right MI tasks, GACL-Net achieved 99.52% classification accuracy and 97.43% generalization accuracy under leave-one-subject-out cross-validation, outperforming existing state-of-the-art methods. Additionally, its real-time processing capability, with prediction times of 33–56 ms on a T4 GPU, underscores its clinical potential for real-time neurofeedback and adaptive rehabilitation. These findings highlight the model’s potential for clinical applications in assessing rehabilitation effectiveness and optimizing therapy plans through precise MI classification.Keywords
Stroke, a severe medical condition caused by the sudden disruption of blood flow to the brain, is a leading global cause of death and disability, affecting millions annually. It profoundly impacts brain function and structure, particularly in regions responsible for movement coordination and sensory processing. Consequently, stroke survivors often face motor deficits, impaired coordination, and disrupted fine motor skills, significantly hindering daily activities such as walking, grasping, and writing [1]. Beyond physical limitations, these impairments adversely affect emotional well-being, often leading to depression, anxiety, and social isolation. The prolonged rehabilitation required for stroke recovery places a considerable burden on healthcare systems and involves complex, time-intensive processes [2].
Brain-computer interface systems have emerged as promising tools for enhancing cognitive and motor rehabilitation in stroke survivors. Among the various methods used to assess rehabilitation effectiveness, electroencephalography (EEG) is the most widely adopted due to its ability to measure brain activity noninvasively [2,3]. Stroke often disrupts oscillatory patterns in the brain, particularly in motor control and cognitive processing, leading to abnormalities such as reduced coherence, disrupted functional connectivity, and slowed brain waves [4,5]. These EEG biomarkers provide valuable insights for monitoring recovery and tailoring rehabilitation programs [6]. However, challenges such as lesion heterogeneity, individual recovery variability, and the inherent complexity of EEG signals due to artifacts necessitate advanced signal processing and machine learning (ML) techniques to derive meaningful insights [7].
Motor imagery (MI), a technique where patients imagine specific movements to activate corresponding motor regions in the brain, has gained traction in EEG-based rehabilitation [8–10]. MI tasks, such as imagining left- or right-hand movements, promote neuroplasticity and motor recovery by engaging the motor cortex and associated neural pathways. Studies have demonstrated that MI tasks improve cortical reorganization and motor learning, making them effective tools for stroke rehabilitation [11,12]. When combined with therapies such as physiotherapy and transcranial magnetic stimulation, MI-based rehabilitation can further enhance motor function recovery [13].
Despite its potential, MI-based rehabilitation faces several challenges. One primary challenge is the variability in patients’ ability to perform MI. Not all stroke survivors can accurately visualize or engage in the motor networks required for effective imagery [14–18]. Variability remains a function of several factors, such as stroke severity, cognitive impairments, and differences in individual imagery skills [19–22]. Moreover, measuring MI quality is challenging due to its subjectivity, making it difficult for therapists to ensure patients perform tasks correctly [23,24].
Advanced classification methods are required to address these limitations and improve the effectiveness of personalized rehabilitation strategies. For example, accurately differentiating between left and right MI can ensure the activation of correct motor networks, leading to improved recovery outcomes [25,26]. Nevertheless, accurate classification of MI tasks remains challenging because neural signatures can overlap between left and right MI, particularly in stroke patients who may have restructured brain networks. Misclassification can lead to incorrect feedback and negatively affect the therapeutic benefits of MI-based rehabilitation [24,27]. Some advanced ML methods such as convolutional neural networks (CNNs), EEGNet, and hybrid networks have shown improved classification outcomes [18–20]. However, the high variability in EEG data across stroke patients limits their generalizability. Compensatory neural mechanisms and the diversity of lesion locations further hinder the accuracy of detecting MI-specific patterns [21,23].
This study addresses the following key challenges associated with MI-based classification models:
1. The variability in EEG patterns of stroke-induced individuals is challenging. Lesion location and severity can result in diverse EEG responses, making it difficult to produce generalized classification models [17,20,23].
2. Accurately distinguishing between left and right MI is difficult due to subtle and overlapping brain patterns. Traditional models often exhibit poor classification outcomes with nuanced details [19,27].
3. Compensatory neural mechanisms in stroke patients can alter MI-generated EEG patterns, complicating accurate classification. Thus, models often perform poorly under such scenarios [21,24].
To overcome these challenges, this paper proposes a graph attentive convolutional long short-term memory (LSTM) network (GACL-Net). The model comprises a hybrid deep learning architecture specifically designed to enhance the generalization and classification performance of MI tasks for stroke patients under highly variable patterns. The model includes a multi-scale convolutional block with parallel one-dimensional (1D) convolutions that enable multi-scale feature extraction to capture diverse signal patterns. An attention fusion layer combines spatial, temporal, and frequency attention mechanisms to focus on relevant features adaptively, improving the model’s ability to handle higher data variability. A graph convolutional layer simulates inter-channel connectivity and captures complex dependencies between EEG channels. Bidirectional LSTM layers with attention capture sequential dependencies and emphasize significant temporal features, capturing subject-specific data.
Hierarchical feature aggregation is enabled through a network of global average pooling and dense layers that further refine the extracted features, with regularization techniques such as L2 regularization. This stacked architecture provides a comprehensive framework to enhance generalization robustness under high subject variability. The model has been combined with a feature extraction routine targeting spatial, temporal, and spectral characteristics of the data and tested on an open-source dataset comprising EEG patterns of stroke patients. Overall, this study makes the following contributions:
1. Introduces a novel model architecture integrating multi-scale convolutional layers, graph convolution, and attention fusion to capture spatial, temporal, and spectral patterns in stroke EEG data. This aids in enhancing the extraction of relevant features across diverse frequency bands.
2. Offers enhanced generalization and subject variability handling, thus providing robustness across inter-subject and intra-subject differences while focusing on MI tasks.
3. Combines deep learning techniques with adaptive attention mechanisms and feature extraction routines to address data heterogeneity and variability inherent in EEG signals from stroke patients.
The remainder of this paper is structured as follows. Section 2 reviews existing models for MI classification in stroke rehabilitation. Section 3 details the proposed GACL-Net architecture. Section 4 presents experimental results, and Section 5 provides a comparative analysis and discussion. Section 6 concludes the study.
MI classification for rehabilitation has spawned numerous models, from traditional ML approaches to sophisticated deep hybrid networks. Traditional models relying on manual feature extraction have proven inadequate for complex MI tasks, particularly in stroke patients. Similarly, conventional deep learning architectures like CNNs [28] and LSTM [26] show limited classification rates, failing to capture subtle patterns even with increased network depth.
Cyclic generative adversarial networks (GAN) have addressed data availability constraints [20,25], yet their classification capabilities remain restricted for complex patterns. While transformer-based modeling captures long-range dependencies and temporal patterns in EEG signals, these networks struggle with data variability and demand substantial computational resources. Graph neural networks with temporal convolutions, designed to model dynamic brain region interactions, face scalability issues and accuracy degradation across variable EEG patterns. Hybrid networks combining multiple layers often compound individual layer limitations, particularly under high variability conditions.
Several studies have investigated MI tasks specifically for stroke patients. Voinas et al. [17] implemented a random forest (RF) algorithm incorporating wavelet packet decomposition (WPD), higher-order statistics (HOS), and common spatial pattern features (CSP), and filter-bank common spatial pattern (FBCSP) achieving 71% accuracy with six stroke patients but showing limited cross-subject generalization. Djamal et al. [18] developed a CNN-based classification using frequency band features, reaching 90% accuracy across 25 stroke patients and 25 healthy subjects. EEGNet [19], achieved 69.75% accuracy with ten hemiparetic stroke patients performing left and right MI tasks. Xu et al. [20] combined cycle GAN for data generation with CNN evaluation, attaining 78.3% accuracy. Miladinović et al. [21] compared spatial filtering techniques including source power co-modulation (SPoC), spectrally weighted CSP (Spec-CSP), and FBCSP, with FBCSP achieving 85.1% accuracy across five stroke patients, though cross-subject variability remained challenging. Benzy et al. [23] explored phase locking value (PLV) and event related desynchronization/synchronization (ERD/ERS), reaching 74.4% accuracy but struggling with diverse stroke condition generalization. EEGNet with transfer learning (TL) was proposed [24] to improve generalization, the performance was still limited by the high variability in stroke patient data.
A critical analysis as shown in Table 1 reveals persistent limitations despite these advancements. TL approaches using pre-trained EEGNet models show generalization potential but face computational constraints and struggle with stroke patient data variability. CycleGAN-based data generation techniques address limited data availability but remain sensitive to distribution shifts and struggle to capture EEG data nuances in stroke rehabilitation. These limitations underscore the need for more sophisticated approaches addressing the complex, variable nature of stroke-affected EEG signals. The proposed GACL-Net tackles these challenges through an innovative architecture combining multi-scale feature extraction, attention mechanisms, and graph convolutional layers to enhance generalization and classification performance.
The system design encompasses feature generation and model training stages, preceded by data preprocessing. Raw EEG data undergoes filtering, normalization, windowing, augmentation, and splitting. The model incorporates non-conventional layers strategically stacked for optimal boundary decisions. The workflow of GACL-Net is shown in Algorithm 1.
The study utilized a publicly available dataset [29] comprising EEG responses from 50 acute stroke patients performing 40 MI tasks each. The dataset included 33 EEG and electro-oculography (EOG) channels focusing on the motor cortex, with participants executing left and right hand MI guided by visual prompts. Each 8-s trial consisted of instruction, MI, and rest phases. Participants (31–77 years old, 7.8:2.2 male-to-female ratio) underwent 20-min sessions, including preparation. The dataset incorporated clinical metrics such as National Institute of Health stroke scale, modified Barthel index, and modified Rankin scale scores, facilitating MI classification by capturing subtle differences between left- and right-hand movement patterns in acute stroke patients. Table 2 summarizes key parameters of the dataset.
Data preprocessing encompassed filtering, windowing, normalization, augmentation, and dataset splitting into training, testing, and validation data. The process began with baseline correction using mean (μ) removal for drift reduction, followed by bandpass filtering (0.5–40 Hz) to eliminate noise. Data underwent windowing with 2 s windows and 1 s overlap, then augmentation through Gaussian noise insertion (standard deviation (σ) between 0–0.05) and 1D axis rotation. Z-score normalization was applied using Eq. (1):
xscaled=XEEG−μσ(1)
The feature extraction framework enhances model performance under high-variability conditions in stroke patients by capturing diverse signal characteristics across spatial, temporal, and spectral domains. This framework targets the complex patterns inherent in EEG data, focusing on multi-scale characteristics to ensure robustness across heterogeneous patient data.
The extracted features include alpha- and beta-band power (Powerband), calculated using Welch’s method as shown in Eq. (2) to analyze motor functions typically disrupted in stroke patients. The amplitude envelope, derived through Hilbert-Huang transform calculated by using Eq. (3), captures instantaneous amplitude characteristics sensitive to stroke signal anomalies. Coherence (Cxy) calculated by Eq. (4) measures phase and amplitude coupling between EEG signals, highlighting functional connectivity changes common in stroke patients.
Powerband=∫fhighflowPSD(f)df(2)
where chn denotes the channel and wi denotes the window. Monitoring was performed at 8–13 Hz and 13–30 Hz for alpha and beta bands, respectively.
AmplitudeEnvelope=|ℋ(EEGwichn)|(3)
where EEGwichn is the input and ℋ is the Hilbert transform. Using this feature, local amplitude variations were modeled to represent neuronal excitability and brain response variability in patients with stroke.
Cxy(f)=|Sxy(f)|2Sxx(f)Syy(f)(4)
where Sxy(f) is the cross-spectral density and Sxx(f), Syy(f) are the auto-spectral densities. Any connectivity disruptions across channels can be captured through coherence, signifying the monitoring and recovery of stroke patients.
ERD/ERS calculated by Eq. (5) assess frequency band changes during motor tasks, crucial for evaluating motor impairment. The fractal dimension (FD) calculated by Eq. (5) quantifies signal complexity, which can fluctuate due to neural changes in stroke patients. The Lyapunov exponent (λ) calculated by Eq. (7) captures signal predictability and chaos, providing insights into disrupted neural dynamics post-stroke.
ERD/ERS=PowerTask−PowerBaselinePowerBaseline×100%(5)
FD=log(σ(EEGwichn)μ(EEGwichn))(6)
λ=limt→∞1tn∑i=1log|Δxi|(7)
where Δxi represents differences in EEG signal values.
This comprehensive feature set enables the model to capture signal variability and neural dynamics effectively. The integration of these features enhances model generalization through detailed functional connectivity analysis in stroke patients. Each feature contributes unique insights into the complex patterns of neural activity, making the model particularly suited for analyzing stroke-affected EEG signals. The featured representation serves as input to the GACL-Net model, enabling robust classification of MI tasks.
The proposed GACL-Net was designed to classify left and right MI tasks as MIleft and MIright, respectively. The overall architecture is illustrated in Fig. 1. The input of the model (EEGwifeatured), which is a featured representation of the raw EEG data for each channel over window. The architecture comprises several key components, including multi-scale convolutional blocks (Eq. (8)), attention fusion layers (Eq. (9)), graph convolution layers (Eq. (10)), bidirectional LSTM layers with attention (Eq. (11)), and dense layers with softmax activation function (Eq. (12)). Each of these blocks and layers was stacked to extract and integrate relevant spatiotemporal and spectral features. The model passes the input through a multi-scale convolutional block, which aids in capturing features at different scales using three convolutional layers using kernel sizes (3, 5, 7) for capturing diverse spatial patterns. The convolutional operation is defined as:
Conv(EEGwifeatured)=K∑k=−Kwk⋅EEGwi−kfeatured(8)
where wk are the learnable weights of the convolutional filters and K represents the kernel size. The concatenation of these layers, followed by batch normalization and dropout, further aids in stabilizing learned features. The outcomes from the convolutional layers are passed through the attention fusion layer, which helps combine the spatial, temporal, and frequency patterns of EEG signals by adapting spatial and temporal attention mechanisms. This layer focuses on relevant features by allocating dynamic weights to them, considering their importance. The attention fusion layer captured the following patterns:
Attention(Q,K,V)=softmax(QKT√dk)V(9)
where Q, K, and V are the query, key, and value matrices, respectively, derived from the input. The next block for extracting information is the graph convolutional layer, which focuses on interchannel connectivity. This helps to simulate the brain’s functional network by capturing the dependencies between different EEG channels. Thus, the model transforms and captures information through inter-channel interactions. The outcomes are expressed as follows:
GraphConv(EEGwifeatured)=∑nAij⋅Conv(EEGwifeatured)(10)
where Aij denotes the adjacency matrix representing the connectivity between channels. Following the capture of these dependencies, the model incorporated a Bi-LSTM layer that captured the temporal dependencies in the EEG data by processing the input in the forward and backward directions. The layer takes information from the past and future and is embedded to improve the overall feature-learning process. The procedure was as follows:
ht=LSTM(→EEGwifeatured,←EEGwifeatured)(11)
where →EEGwifeatured and ←EEGwifeatured represent the forward and backward passes of the LSTM network, respectively. The outcomes from these layers are passed through global average pooling, which condenses the feature representation and is followed by fully connected layers and regularization. This ensures that the model captures high-level abstractions and avoids overfitting. The final layer is a softmax layer that outputs the probabilities for each class (MIleft and MIright) as follows:
Softmax(zi)=ezi∑jezj(12)
where zi is the input to the softmax function for class i.
Figure 1: Schematic architecture of the proposed GACL-Net for MI classification in stroke patients. The model incorporates multi-scale convolutional blocks for diverse feature extraction, attention fusion layers for adaptive feature focus, graph convolutional layers to capture inter-channel dependencies, and bi-directional LSTM layers with attention for temporal dynamics modeling. This hierarchical structure enables effective learning of complex spatial-temporal patterns in EEG signals
The rationale for integrating these components into GACL-Net can be analyzed from a biologically informed perspective. The model design has been developed to capture the spatio-temporal and spectral dynamics that are inherent in the EEG signals. The multiscale convolutional blocks have been employed with varying kernel sizes of 3, 5 and 7 to capture the neural activities at different spatial scales. These can help explore the varying spatial resolutions of brain signal patterns. The attention fusion layers help emphasize spatial, temporal, and frequency features, and serve as adaptive mechanisms to focus on relevant neural activities (associated with the targeted classes). The graph convolutional network module helps in improving connectivity modeling by capturing inter-channel dependencies through the use of an adjacency matrix. The module offers strength to the model by capturing both spatial and functional network information. The bi-LSTM layer then captures the sequential dependencies and ensures that the variability and overlapping patterns in the EEG data are fully understood. Overall, this combination of convolutional, attention, graph convolution, and LSTM layers allows the model to effectively learn complex patterns from EEG data. Thus, it is highly suitable for distinguishing between MIleft and MIright. The hierarchical structure ensures that both spatial and temporal dynamics are captured.
The architecture of GACL-Net is developed as modular blocks. Each of these blocks has been designed to process the EEG signals hierarchically. The input data are initially in the form of samples, channels, windows, and features. The last three dimensions are combined to return a shape of 1848 feature samples passed on the model. An overall flow of input shapes between the model blocks is presented in Table 3.
The attention fusion layer, strategically positioned after the multi-scale convolution, processes enriched spatial features through sophisticated spatial and temporal attention mechanisms. While maintaining the input shape, this layer adaptively refines feature representations, ensuring subsequent layers operate on the most salient aspects of the data. This integration significantly enhances the model’s robustness to EEG variability, ultimately improving classification performance across diverse patient populations.
The GACL-Net model was trained using the Adam optimizer with a learning rate of 1×10−4. The dataset was split into training and testing sets with an 80:20 ratio, with 20% of the training data reserved for validation. Training was conducted over 20 epochs using a batch size of 32. The model employed categorical cross-entropy as the loss function, with accuracy as the primary performance metric. Model evaluation incorporated multiple metrics including accuracy, precision, recall, and F1-score on the test data.
This section presents the results generated for the proposed model, indicating the presence of nuanced boundaries highlighted using feature visualization and the corresponding power of the GACL-Net model to correctly classify the classes in the presence of such decision boundaries. Additionally, the use of cross-validation further helps analyze the generalization capabilities of the model. These outcomes have been presented in the ensuing paragraphs.
4.1 Statistical Analysis under Stroke Conditions
To analyze the variability across subjects with stroke records, statistical analysis was performed for the left and right MI conditions. Variability was determined in terms of μ and σ under visualization and high-end statistics, including analysis of variance (ANOVA).
Analysis of the μ and σ across subjects (including all channels) indicated high variability. The mean variations across subjects are shown in Fig. 2. The variations ranged from as low as 3331.66 to as high as 33,276.57. This wide range indicates that different subjects have markedly different average EEG signal magnitudes. For instance, Subject 19 had a low μ EEG signal of 3331.66, whereas Subject 22 had a significantly high μ of 33,276.57. These differences indicate variations in baseline EEG activities across individuals; therefore, a more generalized model is required to effectively capture such differences when performing cross-subject variations. The overall pattern is shown in Fig. 3.
Figure 2: Statistical analysis of EEG signal variability across stroke patients. (a) μ of EEG signal values for each subject, demonstrating high inter-subject variability ranging from 3331.66 to 33,276.57. (b) σ of EEG signals for each subject, illustrating diverse levels of signal fluctuation across patients, with values ranging from 172.63 to 1320.13
Figure 3: Statistical analysis and model performance. (a) Distribution of mean EEG signal values across all subjects, illustrating the heterogeneity in baseline EEG activity among stroke patients. (b) Confusion matrix showing the classification performance of the GACL-Net model on the test dataset
A similar σ analysis was performed to measure the variability and dispersion of the signal values, as shown in Fig. 2. The σ analysis showed a considerable range among the participants, with values ranging from 172.63 to 1320.13. Such variations reflect different levels of signal fluctuation and noise across subjects. For instance, Subject 24 had a high σ of 1320.13, which indicates substantial variability in the EEG signals. Subject 20 had a significantly lower σ of 172.63, which suggests a relatively stable EEG signal behavior. Significant differences in σ across subjects revealed that the extent of signal variability was not uniform. This highlights the diversity of the EEG signal characteristics among individuals.
ANOVA analysis revealed key insights into EEG signal variability under stroke conditions for left and right MI tasks. The μ of EEG signals showed an F-value of 0.704 with a p-value of 0.593, while σ analysis yielded an F-value of 0.435 with a p-value of 0.783. These high p-values indicate no significant differences in either μ or σ between left and right MI tasks. While substantial inter-subject variability was observed, the consistency between classes within subjects suggests considerable pattern overlap. This pattern suggests neural restructuring post-stroke, where left and right brain activities become more convergent. These findings highlight the challenge of distinguishing between MI classes due to nuanced patterns, making high accuracy classification particularly demanding.
4.2 Capturing Nuanced Patterns
Topographic map analysis was conducted to examine the similarities between MI classes. The analysis revealed subtle pattern differences that are typically challenging to distinguish between classes. Despite these minimal differences, the model’s sophisticated architecture successfully classified these instances with over 99% accuracy.
The topographic maps in Fig. 4 illustrate the left and right MI class patterns, generated from the mean of the 10 most nuanced trials across subjects. Using normalized activation values (0 to 1) for individual channels, the analysis revealed minimal class differences of approximately 0.004. Although these magnitude differences are small, the spatial activation patterns showed class-specific variations, demonstrating the model’s capability to detect and classify subtle EEG pattern differences.
Figure 4: Topographic maps for mean activation patterns (10 closes class samples) for MI tasks. (a) Class 1 activation map highlights the average spatial distribution of activations across EEG channels for left MI. (b) Class 2 activation map highlights the average spatial distribution of activations across EEG channels for right MI. (c) Difference map, highlighting subtle differences in activation intensities between the two classes
This analysis validates the model’s effectiveness in capturing fine-grained neural patterns, a crucial capability for accurate MI classification in stroke rehabilitation. The successful differentiation of such subtle patterns underscores the model’s sensitivity to small but significant variations in neural activity.
4.3 Training and Validation Performance
The training and validation performances of the proposed model were evaluated using several metrics. Initially, the training outcomes were determined by investigating the loss and accuracy performance over increasing epochs using the training and validation subsets. The model was found to converge suitably when and gradually with increasing epochs without depicting any instances of overfitting or underfitting. The training accuracy started at 0.495 and gradually exceeded 99% across both the training and validation datasets. Similarly, compared to an initial loss of 0.7103, the model converged to a loss value of 0.185. These outcomes are depicted in Fig. 5 for accuracy and loss performance.
Figure 5: Training and validation performance of the GACL-Net over 30 epochs. (a) Training and validation accuracy curves, demonstrating consistent improvement and convergence of the model. The final accuracy exceeds 99% for both training and validation sets, indicating robust learning without overfitting. (b) Training and validation loss curves, show a steady decrease from an initial value of 0.7103 to a final value of 0.185, further confirming the model’s effective learning and generalization capabilities
Model evaluation was conducted on a held-out 20% test subset, completely separate from the training data. The test set performance closely matched the training and validation results, with the model achieving 99.52% accuracy, demonstrating robust generalization without overfitting. The confusion matrix in Fig. 3 and the performance metrics in Table 4 confirm these findings at a 95% confidence interval.
To validate the model’s generalization capabilities, leave-one-subject-out cross-validation (LOSO-CV) and performance evaluation on an additional dataset (Dataset 2) [30] were conducted. The LOSO-CV methodology assessed the model’s performance by completely excluding one subject’s data during training, providing a rigorous test of generalization to unseen subjects. This process was repeated 50 times, with each iteration holding out a different subject’s data as the test set. The model maintained robust performance, showing only a 2.1% accuracy decline as shown in Table 4.
Further validation utilized Dataset 2, containing EEG recordings during left and right MI tasks involving paretic and unaffected hands of 15 stroke patients, with each participant performing 40 trials. The recordings featured 63-channel EEG data sampled at 512 Hz, with each trial containing 3500 data points (6.84 s). The data underwent bandpass filtering (0.5–40 Hz) for baseline removal. When evaluated using standard protocols, the model demonstrated comparable performance, exhibiting only a 1.3% accuracy reduction compared to the primary dataset results, as detailed in Table 4.
4.4 Model Complexity and Feature Selection
A feature selection routine has been implemented in this study to analyze the impact of model performance and complexity levels [31]. A genetic algorithm (GA) has been employed to optimize the subset of features that contribute most significantly to the model’s performance [32]. During experimentation, the number of generations (ngen) in the GA was varied, resulting in different combinations of selected features. These groups were then evaluated using the existing GACL model. The primary objective of the GA is to identify the set of features that maximizes performance while potentially reducing computational complexity. The complexity has been characterized as logarithmic big-O, as described by using Eq. (13):
O=log10(F)⋅log10(33⋅480+2⋅(332⋅32+33⋅1024⋅F)+33⋅6144)(13)
where F represents the number of selected features, and the terms correspond to the contributions from the multi-scale convolutional block, attention fusion layer, and graph convolutional layer, respectively.
The outcomes of the feature selection and complexity analysis are summarized in Table 5. This table illustrates the complexity and performance of the model based on varying ngen and selected features. It was found that maximum model performance was achieved with all eight features selected; as features were reduced, model performance correspondingly decreased. Nevertheless, model complexity is proportional to the number of features, highlighting a trade-off between performance and computational efficiency.
Comprehensive ablation experiments systematically evaluated the contribution of key components in the GACL-Net architecture. The first ablation model (A1) excluded the multi-scale convolutional block, responsible for capturing spatial patterns at various scales. This omission reduced classification accuracy by 8% to 0.9255 on the test dataset. The second ablation model (A2) removed the attention fusion layer, which extracts spatial, temporal, and frequency-specific features, decreased accuracy by 9.3% to 0.9023, demonstrating the importance of adaptive feature weighting. The third ablation study (A3) removed the graph convolutional layer, designed for modeling inter-channel dependencies and functional connectivity. This elimination of channel interactions reduced model performance by 5% to 0.9454. Excluding the bidirectional LSTM with attention is the fourth experiment (A4), which captures sequential dependencies and temporal dynamics, decreased accuracy by 7.2% to 0.9239, highlighting the significance of temporal information in MI classification.
Further studies investigated component synergies and their combined effects. The fifth ablation (A5) removed both the multi-scale convolutional block and attention fusion layer, resulting in a substantial 14.5% accuracy reduction and revealing strong synergistic relationships. The sixth study (A6) eliminated the graph convolutional layer and bi-directional LSTM with attention, reducing accuracy by 12.5% and demonstrating the importance of combined temporal dynamics and channel relationships. The final ablation (A7) removed the attention fusion layer and graph convolutional layer, causing an 11.5% accuracy drop and highlighting the interdependence of adaptive attention and inter-channel connectivity. A summary of the ablation studies performance is shown in Table 6.
The proposed GACL-Net model demonstrates outstanding performance in MI classification for stroke rehabilitation, achieving 99.52% accuracy on the test dataset and 97.43% under LOSO-CV. These results surpass existing methods, addressing key challenges in EEG-based MI classification, including patient variability, overlapping neural signatures, and compensatory mechanisms. Statistical analysis revealed substantial EEG signal variability among stroke patients, with μ ranging from 3331.66 to 33,276.57 and σ from 172.63 to 1320.13. Despite this heterogeneity, GACL-Net maintains robust generalization, underscoring its ability to detect subtle neural patterns that conventional models often miss. The model’s efficient processing speed (33–56 ms on a T4 GPU) further enhances its practical utility for real-time clinical applications, where immediate feedback is critical.
Comparative analysis in Table 7 demonstrates GACL-Net’s superior performance against existing approaches in MI classification for stroke patients. Traditional ML models like RF, which rely on hand-crafted features (such as WPD or CSP) [17], struggle with inter-subject variability and overlapping neural signatures due to EEG data complexity. Lightweight architectures such as EEGNet [19] and CNN [18] show limited ability to capture nuanced temporal and spatial patterns. Even with TL [24], EEGNet’s performance remains constrained by the unique complexities of stroke patient EEG data, particularly in handling non-stationary signals. Similarly, spatial filtering methods [21] and PLV approaches using ERD/ERS [23] achieve moderate performance but fall short due to disrupted brain connectivity patterns in stroke patients.
GACL-Net’s exceptional classification accuracy stems from its sophisticated architectural features. Multi-scale convolutional blocks capture diverse spatial patterns at different scales, enabling detection of subtle EEG signal differences. Attention fusion layers adaptively focus on relevant features, addressing high variability in stroke-induced patterns. Graph convolutional layers model complex brain functional connectivity through inter-channel dependencies, particularly crucial for stroke patients with disrupted neural patterns. The bi-directional LSTM with attention mechanisms captures long-term temporal dependencies bidirectionally, essential for understanding dynamic EEG signals during MI tasks. This comprehensive architecture enables superior MI classification in stroke patients.
5.2 Statistical Validation of Performance Metrics
Statistical validation of GACL-Net’s performance improvements utilized paired t-tests and bootstrap confidence intervals for comparison against baseline models. Paired t-tests revealed significant differences in accuracy across all samples, with low p-values (p < 0.001) confirming statistical significance. Bootstrap confidence interval analysis further validated these findings. GACL-Net demonstrated superior performance across all comparisons as shown in Table 8, with accuracy improvements ranging from 9.53% vs. CNN to 32.99% vs. EEGNet with TL. The consistently positive, non-zero-overlapping confidence intervals provide robust statistical evidence of GACL-Net’s enhanced classification capabilities.
GACL-Net’s exceptional accuracy offers significant implications for clinical rehabilitation practices. The model’s precise MI task classification enables tailored rehabilitation protocols, enhancing therapeutic effectiveness through personalized treatment approaches. Its potential integration into real-time neurofeedback systems provides immediate, accurate patient feedback during MI tasks, potentially accelerating motor function recovery. The model’s sensitivity to subtle EEG pattern changes enables precise monitoring of rehabilitation progress, allowing clinicians to make data-driven adjustments to treatment plans. This capability, combined with the system’s real-time processing (33–56 ms on T4 GPU), makes it particularly valuable for clinical applications where immediate feedback is crucial. Furthermore, GACL-Net’s robust performance establishes new research opportunities in neural plasticity and motor recovery mechanisms, advancing our understanding of stroke rehabilitation processes and potentially leading to innovative therapeutic approaches.
5.4 Limitations and Future Directions
Despite GACL-Net’s strong performance, several limitations warrant attention in future research. While the current datasets are substantial, validation across larger and more diverse patient populations remains necessary to confirm generalizability. The study lacks longitudinal assessment of rehabilitation outcomes, necessitating extended studies to evaluate long-term recovery tracking efficacy. Additionally, the model’s controlled-setting performance may not directly translate to clinical environments, requiring further testing and adaptation for practical implementation. Though achieving high accuracy, the model needs enhanced interpretability to help clinicians understand the EEG features driving classifications.
The study excluded several advanced architectures from consideration. Transformer-based models with self-attention mechanisms, capable of capturing long-range dependencies and temporal dynamics in EEG data, were omitted due to their extensive computational requirements and the limited availability of stroke patient data. Domain adaptation and TL approaches, while promising for improving generalizability, face limitations from inherent heterogeneity in stroke EEG data. Advanced data augmentation methods like GAN and CycleGAN were also excluded due to their sensitivity to distribution shifts and challenges in capturing nuanced variability in stroke rehabilitation EEG data.
Future research should focus on integrating GACL-Net with complementary neuroimaging modalities such as functional near-infrared spectroscopy or functional magnetic resonance imaging to enhance understanding of brain activity during MI tasks [33]. Additional priorities include exploring recovery outcome prediction, optimizing rehabilitation strategies, and developing user-friendly clinical interfaces to facilitate adoption in rehabilitation settings.
GACL-Net represents a significant advancement in EEG-based MI classification for stroke rehabilitation, with its high accuracy and robust handling of inter-subject variability addressing key challenges in personalized rehabilitation strategies.
The proposed GACL-Net presents significant advancements in MI classification for stroke rehabilitation, achieving an impressive 99.52% accuracy. By integrating multi-scale convolutional layers, attention mechanisms, and graph convolutional networks, the model effectively addresses key challenges in stroke EEG analysis, including patient variability, overlapping neural signatures, and compensatory mechanisms. This architecture enhances generalization across diverse stroke-induced EEG signals, capturing subtle neural activity patterns crucial for individualized rehabilitation strategies. The GACL-Net’s potential clinical applications are extensive, ranging from evaluating therapeutic interventions to providing real-time neurofeedback. Its ability to offer detailed insights into motor and cognitive recovery processes, coupled with its adaptability to various stages of recovery, positions it as a versatile tool for personalized rehabilitation. By enabling precise monitoring of rehabilitation progress and facilitating treatment plan adjustments, the GACL-Net paves the way for more effective, tailored stroke rehabilitation strategies, ultimately improving patient outcomes and engagement in the recovery process.
Acknowledgement: The authors would like to thank Muhammad Abdullah Iqbal for his assistance with code debugging.
Funding Statement: This work was supported by the Basic Science Research Program through the National Research Foundation of Korea (NRF), funded by the Ministry of Science and ICT under Grant NRF-2022R1A2C1005316.
Author Contributions: The authors confirm contribution to the paper as follows: study conception and design: Chayut Bunterngchit; data collection: Chayut Bunterngchit, Ashraf ALDabbas and Thanaphon Chearanai; analysis and interpretation of results: Chayut Bunterngchit, Laith H. Baniata, Mohammad H. Baniata, Mohannad A. Khair and Sangwoo Kang; draft manuscript preparation: Chayut Bunterngchit and Laith H. Baniata. All authors reviewed the results and approved the final version of the manuscript.
Availability of Data and Materials: The code is available at https://github.com/yiamcb/GACL-Net (accessed on 18 January 2025) and the datasets used in this study are publicly available at https://figshare.com/articles/dataset/EEG_datasets_of_stroke_patients/21679035/5 and https://figshare.com/articles/dataset/EEG_data_of_motor_imagery_for_stroke_patients/7636301 (accessed on 18 January 2025).
Ethics Approval: Not applicable.
Conflicts of Interest: The authors declare no conflicts of interest to report regarding the present study.
References
1. Poomalai G, Prabhakar S, Sirala Jagadesh N. Functional ability and health problems of stroke survivors: an explorative study. Cureus. 2023 Jan;15(1):e33375. [Google Scholar]
2. Vavoulis A, Figueiredo P, Vourvopoulos A. A review of online classification performance in motor imagery-based brain-computer interfaces for stroke neurorehabilitation. Signals. 2023;4(1):73–86. doi:10.3390/signals4010004. [Google Scholar] [CrossRef]
3. Bunterngchit C, Wang J, Chearanai T, Hou ZG. Enhanced EEG-fNIRS classification through concatenated convolutional neural network with band analysis. In: 2023 IEEE International Conference on Robotics and Biomimetics (ROBIO); 2023;Piscataway, NJ, USA:IEEE. p. 1–6. [Google Scholar]
4. Yang H, Wan J, Jin Y, Yu X, Fang Y. EEG- and EMG-driven poststroke rehabilitation: a review. IEEE Sens J. 2022;22(24):23649–60. doi:10.1109/JSEN.2022.3220930. [Google Scholar] [CrossRef]
5. Poveda-García A, Moret-Tatay C, Gómez-Martínez M. The association between mental motor imagery and real movement in stroke. Healthcare. 2021;9(11):1568. doi:10.3390/healthcare9111568. [Google Scholar] [CrossRef]
6. Rocha RP, Koçillari L, Suweis S, De Filippo De Grazia M, de Schotten MT, Zorzi M, et al. Recovery of neural dynamics criticality in personalized whole-brain models of stroke. Nat Commun. 2022;13(1):166. doi:10.1038/s41467-022-30892-6. [Google Scholar] [CrossRef]
7. Keser Z, Buchl SC, Seven NA, Markota M, Clark HM, Jones DT, et al. Electroencephalogram (EEG) with or without Transcranial Magnetic Stimulation (TMS) as biomarkers for post-stroke recovery: a narrative review. Front Neurol. 2022;13:827866. doi:10.3389/fneur.2022.827866. [Google Scholar] [CrossRef]
8. Zhong Y, Yao L, Pan G, Wang Y. Cross-subject motor imagery decoding by transfer learning of tactile ERD. IEEE Trans Neural Syst Rehabil Eng. 2024;32:662–71. doi:10.1109/TNSRE.2024.3358491. [Google Scholar] [CrossRef]
9. Saibene A, Caglioni M, Corchs S, Gasparini F. EEG-based BCIs on motor imagery paradigm using wearable technologies: a systematic review. Sensors. 2023;23(5):2798. doi:10.3390/s23052798. [Google Scholar] [CrossRef]
10. Bunterngchit C, Wang J, Su J, Wang Y, Liu S, Hou ZG. AMFN: autoencoder-led multimodal fusion network for EEG-fNIRS classification. Procedia Comput Sci. 2024;250:8–14. doi:10.1016/j.procs.2024.11.002. [Google Scholar] [CrossRef]
11. Liao W, Li J, Zhang X, Li C. Motor imagery brain-computer interface rehabilitation system enhances upper limb performance and improves brain activity in stroke patients: a clinical study. Front Hum Neurosci. 2023;17:1117670. doi:10.3389/fnhum.2023.1117670. [Google Scholar] [CrossRef]
12. Wang A, Tian X, Jiang D, Yang C, Xu Q, Zhang Y, et al. Rehabilitation with brain-computer interface and upper limb motor function in ischemic stroke: a randomized controlled trial. Med. 2024;5(6):559–69.e4. doi:10.1016/j.medj.2024.02.014. [Google Scholar] [CrossRef]
13. Choy CS, Cloherty SL, Pirogova E, Fang Q. Virtual reality assisted motor imagery for early post-stroke recovery: a review. IEEE Rev Biomed Eng. 2023;16:487–98. doi:10.1109/RBME.2022.3165062. [Google Scholar] [CrossRef]
14. Bunterngchit C. Improving motor imagery classification for stroke rehabilitation using a stroke-aware capsule attention network. In: 2024 International Conference on Decision Aid Sciences and Applications (DASA); 2024;Piscataway, NJ, USA:IEEE. p. 1–5. [Google Scholar]
15. Meghdadi AH, Karic MS, Berka C. EEG analytics: benefits and challenges of data driven EEG biomarkers for neurodegenerative diseases. In: 2019 IEEE International Conference on Systems, Man and Cybernetics (SMC); 2019. Vol. 8, p. 1280–5. doi:10.1109/SMC43495.2019. [Google Scholar] [CrossRef]
16. Bunterngchit C, Wang J, Hou ZG. Simultaneous EEG-fNIRS data classification through selective channel representation and spectrogram imaging. IEEE J Transl Eng Health Med. 2024;12:600–12. doi:10.1109/JTEHM.2024.3448457. [Google Scholar] [CrossRef]
17. Voinas AE, Das R, Khan MA, Brunner I, Puthusserypady S. Motor imagery EEG signal classification for stroke survivors rehabilitation. In: 2022 10th International Winter Conference on Brain-Computer Interface (BCI); 2022;Piscataway, NJ, USA:IEEE. p. 1–5. [Google Scholar]
18. Djamal EC, Ramadhan RI, Mandasari MI, Djajasasmita D. Identification of post-stroke EEG signal using wavelet and convolutional neural networks. Bull Electr Eng Inform. 2020;9(5):1890–8. doi:10.11591/eei.v9i5.2005. [Google Scholar] [CrossRef]
19. Raza H, Chowdhury A, Bhattacharyya S. Deep learning based prediction of EEG motor imagery of stroke patients’ for neuro-rehabilitation application. In: 2020 International Joint Conference on Neural Networks (IJCNN); 2020;Piscataway, NJ, USA:IEEE. p. 1–8. [Google Scholar]
20. Xu F, Rong F, Leng J, Sun T, Zhang Y, Siddharth S, et al. Classification of left-versus right-hand motor imagery in stroke patients using supplementary data generated by CycleGAN. IEEE Trans Neural Syst Rehabil Eng. 2021;29:2417–24. doi:10.1109/TNSRE.2021.3123969. [Google Scholar] [CrossRef]
21. Miladinović A, Ajčević M, Jarmolowska J, Marusic U, Silveri G, Battaglini PP, et al. Performance of EEG motor-imagery based spatial filtering methods: a BCI study on Stroke patients. Procedia Comput Sci. 2020;176:2840–8. doi:10.1016/j.procs.2020.09.270. [Google Scholar] [CrossRef]
22. Bunterngchit C, Chearanai T, Bunterngchit Y. Towards robust cross-subject EEG-fNIRS classification: a hybrid deep learning model with optimized feature selection. In: 2024 22nd International Conference on Research and Education in Mechatronics (REM); 2024;Piscataway, NJ, USA:IEEE. p. 291–5. [Google Scholar]
23. Benzy VK, Vinod AP, Subasree R, Alladi S, Raghavendra K. Motor imagery hand movement direction decoding using brain computer interface to aid stroke recovery and rehabilitation. IEEE Trans Neural Syst Rehabil Eng. 2020;28(12):3051–62. doi:10.1109/TNSRE.2020.3039331. [Google Scholar] [CrossRef]
24. Xu F, Miao Y, Sun Y, Guo D, Xu J, Wang Y, et al. A transfer learning framework based on motor imagery rehabilitation for stroke. Sci Rep. 2021;11(1):1. doi:10.1038/s41598-021-99114-1. [Google Scholar] [CrossRef]
25. Xie J, Siyu C, Zhang Y, Gao D, Liu T. Combining generative adversarial network and multi-output CNN for motor imagery classification. J Neural Eng. 2021;18(4):046026. [Google Scholar]
26. Wang P, Jiang A, Liu X, Shang J, Zhang L. LSTM-based EEG classification in motor imagery tasks. IEEE Trans Neural Syst Rehabil Eng. 2018;26(11):2086–95. doi:10.1109/TNSRE.2018.2876129. [Google Scholar] [CrossRef]
27. Xu F, Dong G, Li J, Yang Q, Wang L, Zhao Y, et al. Deep convolution generative adversarial network-based electroencephalogram data augmentation for post-stroke rehabilitation with motor imagery. Int J Neural Syst. 2022;32(9):639. doi:10.1142/S0129065722500393. [Google Scholar] [CrossRef]
28. Alnaanah M, Wahdow M, Alrashdan M. CNN models for EEG motor imagery signal classification. Signal Image Video Process. 2022;17(3):825–30. doi:10.1007/s11760-022-02293-1. [Google Scholar] [CrossRef]
29. Liu H, Wei P, Wang H, Lv X, Duan W, Li M, et al. An EEG motor imagery dataset for brain computer interface in acute stroke patients. Sci Data. 2024;11(1):309. doi:10.1038/s41597-023-02787-8. [Google Scholar] [CrossRef]
30. Jia T. EEG data of motor imagery for stroke. figshare; 2019 [cited 2025 Jan 31]. Available from: https://figshare.com/articles/EEG_data_of_motor_imagery_for_stroke_patients/7636301/6. [Google Scholar]
31. Venu K, Natesan P. Optimized deep learning model using modified whale’s optimization algorithm for EEG signal classification. Inf Technol Control. 2023;52(3):744–60. doi:10.5755/j01.itc.52.3.33320. [Google Scholar] [CrossRef]
32. Babatunde OH, Armstrong L, Leng J, Diepeveen D. A geneticalgorithm-based feature selection. Int J Electron Commun Comput Eng. 2014;5(4):899–905. [Google Scholar]
33. Bunterngchit C, Wang J, Su J, Wang Y, Liu S, Hou ZG. Temporal attention fusion network with custom loss function for EEG-fNIRS classification. J Neural Eng. 2024 Nov;21(6):066016. doi:10.1088/1741-2552/ad8e86. [Google Scholar] [CrossRef]
Cite This Article
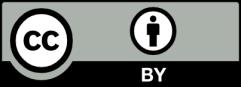
This work is licensed under a Creative Commons Attribution 4.0 International License , which permits unrestricted use, distribution, and reproduction in any medium, provided the original work is properly cited.