Open Access
ARTICLE
GACL-Net: Hybrid Deep Learning Framework for Accurate Motor Imagery Classification in Stroke Rehabilitation
1 State Key Laboratory of Multimodal Artificial Intelligence Systems, Institute of Automation, Chinese Academy of Sciences, Beijing, 100190, China
2 School of Computing, Gachon University, Seongnam, 13120, Republic of Korea
3 Computer Science Department, Faculty of Information Technology, The World Islamic Sciences and Education University, Amman, 11947, Jordan
4 Intelligent Systems Department, Faculty of Artificial Intelligence, Al-Balqa Applied University, Al-Salt, 19117, Jordan
5 IT Infrastructure Department, Qatrana Cement Company, Amman, 11821, Jordan
6 Division of Industrial and Logistics Engineering Technology, Faculty of Engineering and Technology, King Mongkut’s University of Technology North Bangkok, Rayong Campus, Rayong, 21120, Thailand
* Corresponding Author: Sangwoo Kang. Email:
Computers, Materials & Continua 2025, 83(1), 517-536. https://doi.org/10.32604/cmc.2025.060368
Received 30 October 2024; Accepted 01 February 2025; Issue published 26 March 2025
Abstract
Stroke is a leading cause of death and disability worldwide, significantly impairing motor and cognitive functions. Effective rehabilitation is often hindered by the heterogeneity of stroke lesions, variability in recovery patterns, and the complexity of electroencephalography (EEG) signals, which are often contaminated by artifacts. Accurate classification of motor imagery (MI) tasks, involving the mental simulation of movements, is crucial for assessing rehabilitation strategies but is challenged by overlapping neural signatures and patient-specific variability. To address these challenges, this study introduces a graph-attentive convolutional long short-term memory (LSTM) network (GACL-Net), a novel hybrid deep learning model designed to improve MI classification accuracy and robustness. GACL-Net incorporates multi-scale convolutional blocks for spatial feature extraction, attention fusion layers for adaptive feature prioritization, graph convolutional layers to model inter-channel dependencies, and bidirectional LSTM layers with attention to capture temporal dynamics. Evaluated on an open-source EEG dataset of 50 acute stroke patients performing left and right MI tasks, GACL-Net achieved 99.52% classification accuracy and 97.43% generalization accuracy under leave-one-subject-out cross-validation, outperforming existing state-of-the-art methods. Additionally, its real-time processing capability, with prediction times of 33–56 ms on a T4 GPU, underscores its clinical potential for real-time neurofeedback and adaptive rehabilitation. These findings highlight the model’s potential for clinical applications in assessing rehabilitation effectiveness and optimizing therapy plans through precise MI classification.Keywords
Cite This Article
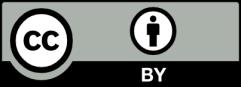
This work is licensed under a Creative Commons Attribution 4.0 International License , which permits unrestricted use, distribution, and reproduction in any medium, provided the original work is properly cited.