Open Access
ARTICLE
Semi-Supervised New Intention Discovery for Syntactic Elimination and Fusion in Elastic Neighborhoods
School of Information and Electronic Engineering, Hebei University of Engineering, Handan, 056038, China
* Corresponding Author: Di Wu. Email:
Computers, Materials & Continua 2025, 83(1), 977-999. https://doi.org/10.32604/cmc.2025.060319
Received 29 October 2024; Accepted 17 January 2025; Issue published 26 March 2025
Abstract
Semi-supervised new intent discovery is a significant research focus in natural language understanding. To address the limitations of current semi-supervised training data and the underutilization of implicit information, a Semi-supervised New Intent Discovery for Elastic Neighborhood Syntactic Elimination and Fusion model (SNID-ENSEF) is proposed. Syntactic elimination contrast learning leverages verb-dominant syntactic features, systematically replacing specific words to enhance data diversity. The radius of the positive sample neighborhood is elastically adjusted to eliminate invalid samples and improve training efficiency. A neighborhood sample fusion strategy, based on sample distribution patterns, dynamically adjusts neighborhood size and fuses sample vectors to reduce noise and improve implicit information utilization and discovery accuracy. Experimental results show that SNID-ENSEF achieves average improvements of 0.88%, 1.27%, and 1.30% in Normalized Mutual Information (NMI), Accuracy (ACC), and Adjusted Rand Index (ARI), respectively, outperforming PTJN, DPN, MTP-CLNN, and DWG models on the Banking77, StackOverflow, and Clinc150 datasets. The code is available at , accessed on 16 January 2025.Keywords
Cite This Article
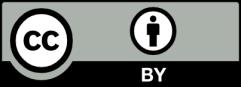
This work is licensed under a Creative Commons Attribution 4.0 International License , which permits unrestricted use, distribution, and reproduction in any medium, provided the original work is properly cited.