Open Access
ARTICLE
An Efficient Instance Segmentation Based on Layer Aggregation and Lightweight Convolution
1 College of Mechanical Engineering, Chongqing University of Technology, Chongqing, 400054, China
2 Robot and Intelligent Manufacturing Technology, Key Laboratory of Chongqing Education Commission of China, Chongqing, 400054, China
* Corresponding Author: Hui Jin. Email:
(This article belongs to the Special Issue: Novel Methods for Image Classification, Object Detection, and Segmentation)
Computers, Materials & Continua 2025, 83(1), 1041-1055. https://doi.org/10.32604/cmc.2025.060304
Received 29 October 2024; Accepted 16 January 2025; Issue published 26 March 2025
Abstract
Instance segmentation is crucial in various domains, such as autonomous driving and robotics. However, there is scope for improvement in the detection speed of instance-segmentation algorithms for edge devices. Therefore, it is essential to enhance detection speed while maintaining high accuracy. In this study, we propose you only look once-layer fusion (YOLO-LF), a lightweight instance segmentation method specifically designed to optimize the speed of instance segmentation for autonomous driving applications. Based on the You Only Look Once version 8 nano (YOLOv8n) framework, we introduce a lightweight convolutional module and design a lightweight layer aggregation module called Reparameterization convolution and Partial convolution Efficient Layer Aggregation Networks (RPELAN). This module effectively reduces the impact of redundant information generated by traditional convolutional stacking on the network size and detection speed while enhancing the capability to process feature information. We experimentally verified that our generalized one-stage detection network lightweight method based on Grouped Spatial Convolution (GSconv) enhances the detection speed while maintaining accuracy across various state-of-the-art (SOTA) networks. Our experiments conducted on the publicly available Cityscapes dataset demonstrated that YOLO-LF maintained the same accuracy as yolov8n (mAP@0.5 = 37.9%), the model volume decreased by 14.3% from 3.259 to 2.804 M, and the Frames Per Second (FPS) increased by 14.48% from 57.47 to 65.79 compared with YOLOv8n, thereby demonstrating its potential for real-time instance segmentation on edge devices.Keywords
Cite This Article
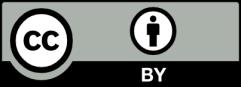
This work is licensed under a Creative Commons Attribution 4.0 International License , which permits unrestricted use, distribution, and reproduction in any medium, provided the original work is properly cited.