Open Access
ARTICLE
CPEWS: Contextual Prototype-Based End-to-End Weakly Supervised Semantic Segmentation
1 School of Computer Science, Zhengzhou University of Aeronautics, Zhengzhou, 450046, China
2 National Key Laboratory of Air-Based Information Perception and Fusion, Luoyang, 471000, China
3 Chongqing Research Institute of Harbin Institute of Technology, Chongqing, 401151, China
4 Aerospace Electronic Information Technology Henan Collaborative Innovation Center, Zhengzhou, 401151, China
* Corresponding Authors: Jiaqi Han. Email: ; Lingling Li. Email:
(This article belongs to the Special Issue: Novel Methods for Image Classification, Object Detection, and Segmentation)
Computers, Materials & Continua 2025, 83(1), 595-617. https://doi.org/10.32604/cmc.2025.060295
Received 29 October 2024; Accepted 02 January 2025; Issue published 26 March 2025
Abstract
The primary challenge in weakly supervised semantic segmentation is effectively leveraging weak annotations while minimizing the performance gap compared to fully supervised methods. End-to-end model designs have gained significant attention for improving training efficiency. Most current algorithms rely on Convolutional Neural Networks (CNNs) for feature extraction. Although CNNs are proficient at capturing local features, they often struggle with global context, leading to incomplete and false Class Activation Mapping (CAM). To address these limitations, this work proposes a Contextual Prototype-Based End-to-End Weakly Supervised Semantic Segmentation (CPEWS) model, which improves feature extraction by utilizing the Vision Transformer (ViT). By incorporating its intermediate feature layers to preserve semantic information, this work introduces the Intermediate Supervised Module (ISM) to supervise the final layer’s output, reducing boundary ambiguity and mitigating issues related to incomplete activation. Additionally, the Contextual Prototype Module (CPM) generates class-specific prototypes, while the proposed Prototype Discrimination Loss and Superclass Suppression Loss guide the network’s training, effectively addressing false activation without the need for extra supervision. The CPEWS model proposed in this paper achieves state-of-the-art performance in end-to-end weakly supervised semantic segmentation without additional supervision. The validation set and test set Mean Intersection over Union (MIoU) of PASCAL VOC 2012 dataset achieved 69.8% and 72.6%, respectively. Compared with ToCo (pre trained weight ImageNet-1k), MIoU on the test set is 2.1% higher. In addition, MIoU reached 41.4% on the validation set of the MS COCO 2014 dataset.Keywords
Cite This Article
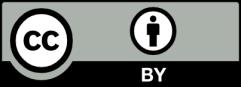
This work is licensed under a Creative Commons Attribution 4.0 International License , which permits unrestricted use, distribution, and reproduction in any medium, provided the original work is properly cited.