Open Access
ARTICLE
Bilateral Dual-Residual Real-Time Semantic Segmentation Network
Institute of Electronic Science and Technology, University of Electronic Science and Technology of China, Chengdu, 611731, China
* Corresponding Author: Dan Tian. Email:
(This article belongs to the Special Issue: Novel Methods for Image Classification, Object Detection, and Segmentation)
Computers, Materials & Continua 2025, 83(1), 497-515. https://doi.org/10.32604/cmc.2025.060244
Received 28 October 2024; Accepted 30 December 2024; Issue published 26 March 2025
Abstract
Real-time semantic segmentation tasks place stringent demands on network inference speed, often requiring a reduction in network depth to decrease computational load. However, shallow networks tend to exhibit degradation in feature extraction completeness and inference accuracy. Therefore, balancing high performance with real-time requirements has become a critical issue in the study of real-time semantic segmentation. To address these challenges, this paper proposes a lightweight bilateral dual-residual network. By introducing a novel residual structure combined with feature extraction and fusion modules, the proposed network significantly enhances representational capacity while reducing computational costs. Specifically, an improved compound residual structure is designed to optimize the efficiency of information propagation and feature extraction. Furthermore, the proposed feature extraction and fusion module enables the network to better capture multi-scale information in images, improving the ability to detect both detailed and global semantic features. Experimental results on the publicly available Cityscapes dataset demonstrate that the proposed lightweight dual-branch network achieves outstanding performance while maintaining low computational complexity. In particular, the network achieved a mean Intersection over Union (mIoU) of on the Cityscapes validation set, surpassing many existing semantic segmentation models. Additionally, in terms of inference speed, the network reached frames per second when tested on an NVIDIA GeForce RTX 3090 GPU, significantly improving real-time performance.Keywords
Cite This Article
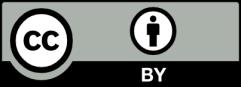
This work is licensed under a Creative Commons Attribution 4.0 International License , which permits unrestricted use, distribution, and reproduction in any medium, provided the original work is properly cited.