Open Access
ARTICLE
Skeleton-Based Action Recognition Using Graph Convolutional Network with Pose Correction and Channel Topology Refinement
1 School of Engineering, Guangzhou College of Technology and Business, Foshan, 528138, China
2 School of Computer Science and Engineering, Dalian Minzu University, Dalian, 116000, China
3 SEAC key Laboratory of Big Data Applied Technology, Dalian Minzu University, Dalian, 116000, China
* Corresponding Author: Qiguo Dai. Email:
(This article belongs to the Special Issue: Advances in Action Recognition: Algorithms, Applications, and Emerging Trends)
Computers, Materials & Continua 2025, 83(1), 701-718. https://doi.org/10.32604/cmc.2025.060137
Received 25 October 2024; Accepted 25 December 2024; Issue published 26 March 2025
Abstract
Graph convolutional network (GCN) as an essential tool in human action recognition tasks have achieved excellent performance in previous studies. However, most current skeleton-based action recognition using GCN methods use a shared topology, which cannot flexibly adapt to the diverse correlations between joints under different motion features. The video-shooting angle or the occlusion of the body parts may bring about errors when extracting the human pose coordinates with estimation algorithms. In this work, we propose a novel graph convolutional learning framework, called PCCTR-GCN, which integrates pose correction and channel topology refinement for skeleton-based human action recognition. Firstly, a pose correction module (PCM) is introduced, which corrects the pose coordinates of the input network to reduce the error in pose feature extraction. Secondly, channel topology refinement graph convolution (CTR-GC) is employed, which can dynamically learn the topology features and aggregate joint features in different channel dimensions so as to enhance the performance of graph convolution networks in feature extraction. Finally, considering that the joint stream and bone stream of skeleton data and their dynamic information are also important for distinguishing different actions, we employ a multi-stream data fusion approach to improve the network’ s recognition performance. We evaluate the model using top-1 and top-5 classification accuracy. On the benchmark datasets iMiGUE and Kinetics, the top-1 classification accuracy reaches 55.08% and 36.5%, respectively, while the top-5 classification accuracy reaches 89.98% and 59.2%, respectively. On the NTU RGB+D dataset, for the two benchmark settings (X-Sub and X-View), the classification accuracy achieves 89.7% and 95.4%, respectively.Keywords
Recently, human action recognition has been widely investigated and it has been adopted in the domain of computer vision, such as in the recognition of abnormal actions using graph convolutional networks, and dynamic hand gesture recognition using 3D convolutional neural network (3D-CNN) and Long Short-Term Memory (LSTM) networks. In particular, Due to its robustness in dynamic environments and complicated backgrounds, skeleton-based action recognition has become more widely studied. In contrast to RGB-based action recognition approaches, action recognition using skeletal data can better remove the interference of background noise and focus on the changes in key points and skeleton structure of the human body, thus improving recognition accuracy. In addition, skeleton-based feature extraction is more adaptable to changes in lighting and viewing angle. Owing to the temporal characteristics of human actions, the skeleton-based approach performs well in temporal modelling in action recognition and can effectively capture the dynamic changes of actions. Overall, using skeletons for action recognition provides significant efficiency, stability, and flexibility advantages.
In earlier works, joint coordinate points were used to represent human feature vectors [1–3], but the connections between body joints were ignored. As deep learning techniques continue to evolve, skeleton data has been treated as a set of independent features, which are then processed into pseudo-images [4–6], or coordinate vector sequences [7–9], and employed to forecast human behaviors through recurrent neural networks (RNNs) or convolutional neural networks (CNNs). However, the inherent connections between joints, which can reflect the topology of the human body, are not represented in these method. Therefore, the connections between body joints should be considered in action recognition using skeletons. In this field, spatial-temporal graph convolutional network (STGCN) [10] is the first graph-based neural network approach, where graphs are used to represent the connections between joints in the human body so as to automatically learn spatial and temporal relationships from the data. Due to the remarkable performance of STGCN in action recognition using skeletons, several GCN-based approaches have also been put forward [11,12] to enhance the model’s performance. However, these methods use a manually defined graph topology in the network structure, making it challenging to capture the relationships between irregularly connected human joints. To overcome this challenge, recent approaches [13–15] learn the human skeleton’s topology by using adaptive graph convolution or other mechanisms. However, these methods use one topology in all channels, which hampers the ability of GCN to extract features effectively. Since each channel corresponds to a distinct type of motion characteristic, and the connections between joints under different motion characteristics may be different, using one or the same topology may be not the best choice.
Furthermore, it is essential to extract key coordinate features of the human body from pictures or videos in action recognition using skeletal data. As pose estimation algorithms continue to evolve, despite that these algorithms have achieved an improved performance, wrong extraction of pose features may occur due to the obscured view or body parts captured by the camera. For example, Human action recognition using encoder-decoder network (HAREDNet) [16] was proposed to overcome the problem of random changes in human variations, illumination, and backgrounds to improve model performance in uncontrolled environments. In this study, we propose pose correction and channel topology graph convolutional network (PCCTR-GCN) to address the above challenges. Compared with STGCN, the PCCTR-GCN which consists of a pose correction module and channel topology graph convolution has achieved a better performance in action recognition. First, we correct the human pose with spatial and temporal information as a way to reduce the likelihood of incorrect estimation of pose features in 2D or 3D human poses. Second, we use channel topology graph convolution to create graph convolutional network units that can dynamically learn the topology and combine joint features from different channel dimensions, in order to enhance the flexibility of GCN in feature extraction. To further improve the accuracy of action recognition, we use a multi-stream fusion network based on raw joint coordinate information (joint streams), spatial coordinate difference information of joint data (bone streams), and temporal dimensional differences in joint streams and bone streams (joint motion streams and bone motion streams), respectively.
To assess the performance of PCCTR-GCN, we perform a series of experiments on three public datasets: iMiGUE [17], Kinetics-Skeleton [18], and NTU-RGB 60 [7]. The experimental results show that our proposed method outperforms other methods on all three datasets. The contribution of this study can be summarized as follows: (1) We propose a new graph convolution learning framework, namely the PCCTR-GCN, which combines pose correction with channel topology refinement graph convolution. In addition, it can correct the pose through learning the temporal and spatial information and reduce potential errors during pose feature extraction. Besides, it can dynamically learn the topology in different channel dimensions and effectively aggregate joint features to enhance the network’s performance. (2) A multi-stream fusion network based on skeleton data is introduced, which includes joint streams, bones streams, joint motion streams and bone motion streams, respectively. The performance of the model has been improved by fusing all data streams. (3) Our approach outperforms other methods on three datasets, as demonstrated by the comparative results.
The organization of the paper is outlined as follows: Section 2 reviews related work on GCN and action recognition based on skeleton data using GCN, while Section 3 gives a detailed explanation of the PCCTR-GCN. Section 4 discusses the experimental design, including ablation studies and experimental results. Section 5 concludes with the research findings and briefly describes the future directions.
2.1 Graph Convolutional Networks
It was graph neural networks (GNN) that constituted the first method for processing graph-structured data. Recently, numerous variations of the technique have been proposed, with a significant amount of emphasis on GCN method owing to the benefits it offers in dealing with graph-structured information. Specifically, spatial and spectral approaches are the two main categories into which GCNs are typically divided. The spatial methods [19–21] to convolutional filters applied directly to graph nodes and their neighbours. Comparatively, in spatial methods, spectral methods [22–25], the spectral domain of the graph signal is transformed to the spectral domain by means of a fourier transform and then perform convolution operations. Furthermore, several strategies have been proposed for enhancing the performance of GCNs. One method adds an attention mechanism that applies distinct weights to different nodes of the neighbors [26]. A subgraph training method is proposed in the work [27] to train the model on large scale graphs with less the memory and computational resource requirements of the model on the graph convolution. Therefore, in this work we empolyed GCN to learn the representation of body gesture from skeleton information estimated by estimation algorithms.
2.2 GCN-Based Skeleton Action Recognition
Human skeleton feature data can be readily obtained using depth sensors and pose estimation techniques, and have strong robustness to complex backgrounds [28] from videos for action recognition using skeletal data. GCN has been widely used [15,29,30], which can be divided into manually marked methods and deep learning methods. ST-GCN [10] was the first to propose the use of spatial-temporal graphs to represent joint sequence data and built a model using graph convolutional networks. Since previous graph topologies are set manually in GCN-based methods, Two-stream adaptive graph convolutional network(2s-AGCN) [13] developed an adaptive graph convolutional network framework that learns graph topologies dynamically for various convolutional layers and skeleton data, offering advantages for action recognition studies. To address the drawback of the large computational complexity of GCN-based methods, Shift-GCN [31] developed a lightweight and efficient graph convolutional network that replaces traditional graph convolutions with shift-graph operations and pointwise convolutions to obtain good results. Similarly, Pose refinement graph convolutional network(PR-GCN) [32] achieved a good balance between accuracy and network parameters through the operation of gradually fusing motion and spatial information. In GCN, the vertex connectivity relations of the skeleton graph contain important information, many researchers are interested in topology-based modeling methods, and such research work can be divided into two categories: 1) whether the topological information can be dynamically adjusted during inference, which can be divided into static methods [10] and dynamic methods [15]; 2) whether the topology is shared in different channels [32–34], it can be further divided into topology shared methods and topology unshared methods. Recently, transformer-based networks have show great potential on skeleton-based action recognition tasks. GCN-Transformer Network [35] employed GCN in combination with a transformer as a means of obtaining action representations that maintain the human skeleton’s natural topology. To extract adjacency matrices with semantically meaningful edges, Hierarchically decomposed graph convolutional network (HD-GCN) [36] employed a hierarchically decomposed GCN for action recognition using skeletal data. Interactional channel excited GCN (ICE-GCN) [37] and large-kernel attention graph convolutional network (LKA-GCN) [38] enhance the accuracy and robustness of action recognition by introducing the interaction channel excitation (ICE) module and skeleton large kernel attention (SLKA) arithmetic. These advancements enable the models to effectively capture interactions among various spatial-temporal patterns and improve the modeling of long-term dependencies. Table 1 shows the topological structure characteristics of each method. From the table, it can be seen that most of the methods use a shared topological structure, and none of the methods simultaneously satisfy both the “Non-shared” and “Dynamic” properties.
In skeleton-based action recognition methods, many approaches begin by utilizing pose estimation tools to propose key point feature coordinates of a video character, followed by training them using corresponding neural network methods. However, the extraction of joint coordinates may be prone to errors due to factors such as the shooting angle of the video or occlusion of the character’s limb parts. Moreover, traditional skeleton-based graph convolution methods for action recognition often rely on a single topology for the channels, which limits the ability of graph convolution to extract meaningful features. Consequently, this limitation can affect the recognition of the model’s performance. In order to address the above issues, this paper built a new graph convolution learning framework, which combines pose correction with channel topology refinement graph convolution.
In this part, we introduce the design of the Pose Correction and Channel Topology Refinement Graph Convolution Network (PCCTR-GCN), including the details of the pose correction module, channel topology graph convolution, and skeleton data multi-stream fusion. The proposed PCCTR-GCN consists of three components as shown in Fig. 1. Firstly, the input pose was estimated and corrected by the pose correction module. Secondly, the channel topology refinement module was used to learn its topology and aggregate joint features in different channel dimensions. Finally, we performed multi-stream data modality fusion, using joint streams, bone streams, joint motion streams, and bone motion streams of body skeleton data, respectively. Independent training was carried out for each stream, and the scores of the four streams were combined to get the final scores. All aspects of the network framework are explained in detail in next section.
Figure 1: Overview of the proposed PCCTR-GCN framework
Graph convolutional networks model body skeleton data as a graph G=(V,E) with joints as vertices and bones as the edges, where V={v1,…,vN} is the set of N vertices and E is the set of edges. The adjacency matrix A∈{0,1}N×N is employed to depict the skeleton graph, where its element aij represents the strength of the connection from vi to vj. The neighboring nodes of vi are denoted as N(vi) and aij≠0. X is the set of features for vertex N, denoted by the matrix X∈RN×C, and the features of vi are denoted as xi∈RC. The shared-topology graph convolution is aggregated by aij to the neighboring vertices of vi and represented by a feature transformation using weights W, which can be denoted as:
zi=∑vj∈N(vi)aijxjW(1)
where aij can be represented in two ways: the parameters are defined manually or can be trained through static methods, and generated by the model of the input samples through dynamic methods.
Although action recognition using skeletal data is highly robust in complex backgrounds, there may be errors in extracting human joint features using pose estimation algorithms, due to the video shooting angle or occlusion of the characters’ body joints by objects, the accurate recognition of body joint coordinates becomes challenging when using OpenPose for extracting key body points.
In this work, the offset (Δx,Δy) of each joint was estimated by the pose correction module and added to the corresponding coordinate (x,y) to reduce the estimation errors. For two-dimensional skeleton sequence, we obtained the coordinates (x,y) of each joint by using the human pose estimation algorithms. As illustrated in Fig. 2, the offset was determined by combining convolution, graph convolution, and temporal convolution layers. Pose correction was achieved by using the graph convolution layer for spatial relationships and the temporal convolution layer for time continuity. Finally, the offset (Δx,Δy) was added to the two-dimensional coordinates of each joint. Similarly, for three-dimensional skeleton sequences, we extracted the location coordinates of the body’s joints, then calculate the offset (Δx,Δy,Δz) of each joint coordinate (x,y,z) using this module and added it to its corresponding coordinate of three-dimensional skeleton sequence. Therefore, the corrected skeleton coordinates can be denoted as:
Xi=xi+Δxi(2)
where Xi is the corrected coordinate, xi is the original coordinate, and Δxi is the offset from the original coordinate. The pose correction module corrects each skeleton coordinate data by estimating the offsets of each joint and adding these offsets to the original skeleton coordinates. This process improves the accuracy of the pose.
Figure 2: Illustration of the pose correction module. “Conv”, “GConvL”, “TConvL” denotes 1×1 convolution layers, graph and temporal convolution layers, respectively
3.3 Channel Topology Refinement Module
To aggregate joint features efficiently, We introduced the channel topology refinement module [33]. As shown in the Fig. 3, the dynamic topology refinement graph convolution is made up of three components: (1) Feature transformation comprising the function T(⋅); (2) Channel topology modeling, including a function M(⋅) for relational modeling and a function R(⋅) for refinement; (3) The features in each channel are aggregated with the corresponding topology by the aggregation function A(⋅), and the final features are taken as output. The process can be represented as:
Z=A(T(X),R(M(X),A))(3)
where Z∈RN×C′ represents the output, X∈RN×C represents the input feature, and A∈RN×N represents a shared topology.
Figure 3: The schematic process of the CTR-GC(Section 3.3)
In feature transformation process, a linear transformation operation was employed as a shared topological graph convolution. The transformation of low-level features into high-level representations via T(⋅) can be denoted as:
˜X=T(X)=XW(4)
where W ∈RC×C′ represents the weight matrix and ˜X∈RN×C′ represents the changed feature.
In channel topology modeling, firstly, the channel correlation Q∈RN×N×C′ between vertices was modeled using the function M(⋅). Assuming that a pair of vertices (vi,vj) is provided, then its corresponding feature is (xi,xj), thus the correlation modeling function M(⋅) can be denoted as:
M(ψ(xi),ϕ(xj))=MLP(ψ(xi)∥ϕ(xj))(5)
where MLP is multilayer perceptron, ∥ stands for concatenate operation. ψ and ϕ are the linear transformation functions used for feature dimensionality reduction. Finally, the channel specificity correlation Q∈RN×N×C′ was obtained through the linear transformation ξ to increase the channel dimension. It can be denoted as:
Qij=ξ(M(ψ(xi)),ϕ(xj)))(6)
where Qij∈RC′ represents the channel-specific relationship between vi and vj. Ultimately, the channel topology R∈RN×N×C′ was determined using the shared topology and the channel-specific correlation Q, it can be denoted as:
R=R(Q,A)=A+α⋅Q(7)
where α represents a scalar quantity that can be used to adjust refinement intensity.
In channel feature aggregation, the modified different channel topologies Rc∈RN×N of different channels were matched with the corresponding high-level features ˜X(c∈{1,...,C′}). The aggregated overall feature Z is the output of the aggregation function A(⋅), it can be denoted as:
Z=A(˜X,R)=[R1˜x:,1∥R2˜x:,2∥⋅⋅⋅∥RC′˜x:,C′](8)
In skeleton-based GCNs studies, 2D or 3D joint coordinates are usually used as input, However, in addition to the raw joint information stream of the skeleton data, the bone information stream (spatial coordinate difference information of joint data) and its corresponding joint motion information stream and bone motion information stream (temporal dimensional differences in joint streams and bone streams) are also important for action recognition. They can be represented by the following mathematic formulas: source joint coordinates defined in frame t can be represented as vi,t,S=(xi,t,S,yi,t,S,zi,t,S), and the target joint can be represented as:
vj,t,T=(xj,t,T,yj,t,T,zj,t,T)(9)
The bones can be depicted as follows:
bi,j,t=(xj,t,T−xi,t,S,yj,t,T−yi,t,S,zj,t,T−zi,t,S)(10)
And the bone stream data is generated by processing the raw joint stream. Motion information can be calculated based on the same joint coordinates or differences in sequential bone frames. The set vi,t=(xi,t,yi,t,zi,t) is the joint at frame t, vi,t+1=(xi,t+1,yi,t+1,zi,t+1) is the same joint at frame t+1, and the motion information is represented as:
mi,t,t+1=(xi,t+1−xi,t,yi,t+1−yi,t,zi,t+1−zi,t)(11)
The network is trained individually by inputting these four data streams to the network. Finally, the fusion of “2s” (joint and bone streams), and “4s” (all data streams) are independently compared to compute the final score. The workings of the PCCTR-GCN framework are outlined in Algorithm 1.
To verify the effectiveness of the pose correction module, the channel topology refinement module, and the multi-stream data fusion frameworks, we analyzed separately their performance in different configurations through extensive experiments on iMiGUE. Then on, we compared the experimental results of PCCTR-GCN on iMiGUE, Kinetics, and NTU RGB+D with that of other methods.
iMiGUE. iMiGUE [17] was jointly released by the University of Qulu and Tianjin University in 2021. It comprises 359 videos collected from online video sharing platforms, and each video lasts 0.5 to 25.8 min, thus amounting to 2,092 min in total. The dataset includes 18,499 action samples with 32 categories of actions (32 illustrative actions), and the duration of these action video clips range from 0.18 to 80.82 s in duration. They are categorized into five types of actions, including “Body-Hand”, “Head-Hand”, “Hand”, “Head”, and “Body”, depending on which part of the body the movement takes place. Fig. 4 shows the action categories and distribution. The links to the original videos are accessible, and then according to the dataset tag file, we obtained the training and test data sets using the video clipping tool FFmpeg. There are two types of labels in the dataset, including action and emotion, and we only use the action label.
Figure 4: The distribution of iMiGUE sample numbers (C32 is illustrative gestures, not shown in the figure)
Kinetics. Kinetics [18] is a classical human action dataset, which has a total of 400 action behavior labels. The skeleton sequences are divided into a training set (240, 000 clips) and a test set (20, 000 clips). The original dataset is in video form, but Yan et al. (2018) [10] extracted human joint data from the video dataset via OpenPose [39], where each joint point has 2D coordinates (X,Y) and a prediction confidence score Z. Each skeleton feature contains 18 body joint points. For clips where multiple people appear in the video, only the two people with the highest confidence scores were selected.
NTU RGB+D. NTU RGB+D 60 [7] is a commonly used dataset for 3D action recognition. The data is collected by the depth camera Microsoft Kinect v2, and there are a total of 56,880 video clips falling into 60 categories. This dataset is available in both video and skeleton sequence formats. Each skeleton data point contains 25 three-dimensional joint coordinates. Two evaluation standards are adopted: 1). X-sub: Training set consists of 40,320 video clips and the test set contains 16,560 video clips, both divided by different actors. 2). X-view: Training set includes 37,920 clips from Camera 2 and Camera 3, while the test set has 18,960 clips from Camera 1, with the division based on the cameras used for recording.
Top-1 and top-5 are commonly used metrics for evaluating skeleton-based action recognition. We report top-1 and top-5 on the test sets of the first two datasets, and the top-1 for the third dataset.
4.2 Details of the Implementation
We first carried out experiments on iMiGUE and re-extracted the skeleton feature data according to the Evaluation Protocols [17] using the OpenPose [39] tool. Fig. 5 shows the body joints in the three datasets. Fifty epochs, weight decay 0.0001, 64 batch size, and an optimizer of SGD were set up in network training. In the first 10 epochs, a learning rate of 0.01 was used, and then it was scaled down by 10 epochs in {20,30},{30,40}, and {40,50}, respectively. In iMiGUE, we kept 150 frames as input to the network. All experiments in this study were conducted under RTX 3090 GPU and PyTorch deep learning framework.
Figure 5: The body joint points of iMiGUE, Kinetics, and NTU RGB+D correspond to left, middle, and right, respectively
To confirm the performance of the PCCTR-GCN component, we performed experiments using the iMiGUE dataset. Firstly, we split the data into training and test sets with a 7:3 ratio, and obtained the training set (13,936 video clips) and test set (4563 video clips). Secondly, the keypoint coordinates of the human body in the video were extracted using OpenPose, and the feature data’s x and y coordinates were normalized to values between 0 and 1. Finally, the processed feature file was used to generate separate training and test set files, along with their corresponding label files.
To evaluate the role of the pose correction module (PCM) in model performance enhancement, we analyzed the impact of this module on action recognition performance. Table 2 illustrates the experimental results on iMiGUE. As we re-extracted skeleton features from the original video dataset using OpenPose, we first performed experiments in the backbone network (STGCN) using joint streams. Then the Pose Correction Module (PCM) was manually added to the network and the differences between the two were compared. As seen from the experimental results, The top-1 and top-5 accuracies of backbone are 49.43% and 86.50%, respectively. After adding PCM to backbone, its top-1 and top-5 accuracies are 51.23% and 87.26%, which are 1.8% and 0.76% higher than that of backbone, respectively. Moreover, to further verify the robustness of PCM, we carried out experiments on the bone-motion, joint-motion, and bone-motion streams, respectively. The experimental results are shown in Table 3 (“w” is the shortened form of “with”, “wo” is the shortened form of “without”), and we compared the performance of PCM on each stream in detail. Experimental results show that adding PCM to the network improves the top-1 and top-5 accuracies for the bone streams, joint motion(JM) streams, and bone motion(BM) streams are 50.22%, 86.72%; 49.01%, 85.07%; and 46.47%, 84.02%, respectively. Among them, for the bone streams and joint motion streams, compared to the case without PCM, the top-1 accuracy and top-5 accuracy increased by 3.03% and 0.57%, and by 1.77% and 0.81%, respectively. For the bone motion stream, compared to the case without PCM, the top-1 accuracy decreased by 0.88%, while the top-5 accuracy improved by 1.12%. Overall, the experimental results indicate that, except for the slightly lower top-1 accuracy in the bone motion streams, the results for other data stream are all better after adding PCM compared to the backbone network.
4.3.2 Channel Topology Refinement Module
To demonstrate that channel topology graph convolution combined with a pose correction module can improve the performance for skeleton-based human action recognition, firstly, according to [33], we constructed a block of graph convolution units using the CTR-GC. The entire network consists of 10 blocks of graph convolution units, which have 64, 64, 64, 64, 64, 128, 128, 128, 256, 256 and 256 output channels, respectively. Then a data batching layer was added to the network layer to normalize the input data. Next, the global average pooling and softmax classifier was used to obtain the prediction results. Secondly, we tested two configurations: in the first configuration, the network was reconstructed using CTR-GC and the impact on joint streams, bone streams, joint-motion streams, and bone-motion streams was analyzed separately. In the second setting, based on the first configuration combined with the PCM, the overall effectiveness on different data streams was verified. Table 4 illustrates the experimental results, the top-1 and top-5 of the four data streams are 52.24%, 87.92%; 50.42%, 87.35%; 46.45%, 83.47%; and 46.84%, 83.10%, respectively, after combining PCM in the CTR-GC-based network. Compared to the use of CTR only, the individual data streams were higher by 1.21%, 0.37%; 2.06%, 0.17%; 0.51%, 0.39%; and 1.73%, 0.04%, respectively. The above results show that the combination of PCM in the network using CTR-GC significantly improves the prediction performance of the model.
4.3.3 Fusion of Multiple Data Streams
To improve accuracy, we examined the impact of fusing multiple data streams on action recognition performance, and Table 5 shows the comparative results between them. Here we have two settings: firstly, the fusion of joint and bone streams, named “2S-PCCTR-GCN”, and secondly, the fusion of four streams, named “4S-PCCTR-GCN”. Moreover, we employed joint streams and bone streams as input, named “1s-PCCTR-GCN” and “Bs-PCCTR-GCN”, respectively, and compared them with the fusion approach. As the experimental results suggest, PCCTR-GCN attains the highest level of performance when all data streams are fused, with top-1 accuracy and top-5 accuracy higher than the other three methods by 2.84% and 1.43%, 4.66% and 2.00%, and 1.31% and 0.46%, respectively. These results indicate that fusing multiple data streams can significantly improve the performance of our proposed method. In particular, the best performance is obtained when using all data streams.
4.3.4 Model Complexity Analysis of PCCTR-GCN
In order to analyze the impact of the individual modules on the complexity of PCCTR-GCN, we calculated the number of parameters (params), required by the model for each round of the test using backbone, PCM, and CTR-GC, respectively. The results of the experiment are presented in Table 6. Compared with the model using only the backbone network, PCCTR-GCN improves the top-1 accuracy and top-5 accuracy by 2.31% and 1.42%, respectively, after adding the modules, although the number of params increases slightly.
4.4 Comparison With the Other Methods
To evaluate the effectiveness of PCCTR-GCN, we compared the performance of the mode with other advanced methods. In this section, three datasets (iMiGUE, kinetic, and NTU RGB+D) were used. In iMiGUE, we selected 12 methods for comparison, among which nine are methods based on skeletal data, namely ST-GCN [10], Shift-GCN [31], S-VAE [40], GCN-NAS [41], 2S-GCN [13], MS-G3D [42], DG-STGCN [43], StrongAug [44] and CTR-GCN [33]. The other three methods are based on RGB data form, including R3D-101 [45], I3D [46], and C3D [47]. The advantages of PCCTR-GCN can be fully demonstrated by comparing a series of various deep learning-based methods with PCCTR-GCN. The performance of these methods was reported in [17]. Table 7 illustrates the experimental results. We can see that the 4s PCCTR-GCN better than other algorithms in top-1 values. Although the recognition accuracy is slightly lower than MS-G3D [42] in top-5 values, a competitive performance was still obtained.
For Kinetics, we compared five GCN-based methods and one self-attentive method [48]. We rounded the results to one decimal place, and Table 8 shows the comparison results with other methods. Specifically, PCCTR-GCN achieves the best model prediction when fusing joint stream and bone streams, with top-1 and top-5 of 36.5% and 59.2%, respectively. The comparative results of our method with other methods in NTU RGB+D are shown in Table 9, among which we conducted experiments on X-Sub and X-View and reported their accuracy on top-1 values, respectively. Specifically, our “4s PCCTR-GCN” obtains 89.7% and 95.4%, respectively, which is the best among the selected algorithms, in addition to the same accuracy as the Two-stream TL-GCN(2s TL-GCN) method in the X-View. Based on the data in Tables 8 and 9, the conclusion can be drawn that the values of top-1 and top-5 for PCCTR-GCN are higher than those of previously reported methods in both datasets. In general, the comparison results above demonstrate that the proposed PCCTR-GCN performs well in human action recognition.
Current many human skeleton action recognition using GCN methods are limited by the use of a single topology, restricting the flexibility of feature extraction. Additionally, pose estimation algorithms may introduce errors when extracting human joint features. To address these issues, we proposed a PCCTR-GCN approach that combines pose correction and channel topology refinement for action recognition using skeleton data. Firstly, a pose correction module was built, which corrects the body joint coordinates and reduces the error of the pose estimation algorithm in feature extracting. Secondly, the graph convolution network was constructed by channel topology graph convolution, which can dynamically learn the topology of different types of motions in different channel dimensions and efficiently aggregate the corresponding joint features. Finally, the bone, joint-motion, and bone-motion streams are extracted on the basis of joint stream, respectively, and a multi-stream model fusion framework was employed. We trained each data feature stream individually and obtained the scores of their fusion. We performed experiments using three datasets to evaluate the effectiveness of the PCCTR-GCN. The results show that it outperforms other methods on these datasets. Although PCCTR-GCN performed well in terms of recognition accuracy, the number of parameters in the network model increased with the addition of PCM and CTR-GC modules. Therefore, we will investigate lightweight components to reduce the model’s parameter complexity in future work. Meanwhile, we will also consider introducing more visual information (e.g., heat maps, and dense maps), expecting to improve the model’s predictive performance.
Acknowledgment: The authors would like to acknowledge Associate Professor Xin Liu from the School of Electrical and Information Engineering, Tianjin University, for the data support.
Funding Statement: The Fundamental Research Funds for the Central Universities provided financial support for this research.
Author Contributions: Yuxin Gao: data collection, writing draft, analysis and interpretation of results; Xiaodong Duan: study conception and design, review; Qiguo Dai: manuscript final layout, fund acquisition. All authors reviewed the results and approved the final version of the manuscript.
Availability of Data and Materials: For model validation, the following public dataset was used: https://github.com/linuxsino/iMiGUE, accessed on 08 March 2022.
Ethics Approval: Not applicable.
Conflicts of Interest: The authors declare no conflicts of interest to report regarding the present study.
References
1. Du Y, Wang W, Wang L. Hierarchical recurrent neural network for skeleton based action recognition. In: Proceedings of the IEEE Conference on Computer Vision and Pattern Recognition; 2015; Boston, MA, USA. p. 1110–8. doi:10.1109/CVPR.2015.7298714. [Google Scholar] [CrossRef]
2. Fernando B, Gavves E, Oramas JM, Ghodrati A, Tuytelaars T. Modeling video evolution for action recognition. In: Proceedings of the IEEE Conference on Computer Vision and Pattern Recognition; 2015; Boston, MA, USA. p. 5378–87. doi:10.1109/CVPR.2015.7299176. [Google Scholar] [CrossRef]
3. Vemulapalli R, Arrate F, Chellappa R. Human action recognition by representing 3D skeletons as points in a lie group. In: Proceedings of the IEEE Conference on Computer Vision and Pattern Recognition; 2014; Columbus, OH, USA. p. 588–95. doi:10.1109/CVPR.2014.82. [Google Scholar] [CrossRef]
4. Soo Kim T, Reiter A. Interpretable 3D human action analysis with temporal convolutional networks. In: Proceedings of the IEEE Conference on Computer Vision and Pattern Recognition Workshops; 2017; Honolulu, HI, USA. p. 20–8. doi:10.1109/CVPRW.2017.207. [Google Scholar] [CrossRef]
5. Li C, Zhong Q, Xie D, Pu S. Skeleton-based action recognition with convolutional neural networks. In: 2017; IEEE International Conference on Multimedia & Expo Workshops (ICMEW); 2017; Hong Kong, China: IEEE. p. 597–600. doi:10.1109/ICMEW.2017.8026285. [Google Scholar] [CrossRef]
6. Liu H, Tu J, Liu M. Two-stream 3D convolutional neural network for skeleton-based action recognition. 2017. doi:10.48550/arXiv.1705.08106. [Google Scholar] [CrossRef]
7. Shahroudy A, Liu J, Ng T-T, Wang G. NTU RGB+D: a large scale dataset for 3D human activity analysis. In: Proceedings of the IEEE Conference on Computer Vision and Pattern Recognition; 2016; Las Vegas, NV, USA. p. 1010–9. doi:10.1109/CVPR.2016.115. [Google Scholar] [CrossRef]
8. Zhang P, Lan C, Xing J, Zeng W, Xue J, Zheng N. View adaptive recurrent neural networks for high performance human action recognition from skeleton data. In: Proceedings of the IEEE International Conference On Computer Vision; 2017; Venice, Italy. p. 2117–26. doi:10.1109/ICCV.2017.233. [Google Scholar] [CrossRef]
9. Zheng W, Li L, Zhang Z, Huang Y, Wang L. Relational network for skeleton-based action recognition. In: 2019 IEEE International Conference on Multimedia and Expo (ICME); 2019; Shanghai, China: IEEE. p. 826–31. doi:10.1109/ICME.2019.00147. [Google Scholar] [CrossRef]
10. Yan S, Xiong Y, Lin D. Spatial temporal graph convolutional networks for skeleton-based action recognition. Proc AAAI Conf Artif Intell. 2018;32(1). doi:10.1609/aaai.v32i1.12328. [Google Scholar] [CrossRef]
11. Si C, Chen W, Wang W, Wang L, Tan T. An attention enhanced graph convolutional LSTM network for skeleton-based action recognition. In: Proceedings of the IEEE/CVF Conference on Computer Vision and Pattern Recognition; 2019; Long Beach, CA, USA. p. 1227–36. doi:10.1109/CVPR.2019.00132. [Google Scholar] [CrossRef]
12. Wen Y-H, Gao L, Fu H, Zhang F-L, Xia S. Graph CNNs with motif and variable temporal block for skeleton-based action recognition. Proc AAAI Conf Artif Intell. 2019;33(1):8989–96. doi:10.1609/aaai.v33i01.33018989. [Google Scholar] [CrossRef]
13. Shi L, Zhang Y, Cheng J, Lu H. Two-stream adaptive graph convolutional networks for skeleton-based action recognition. In: Proceedings of the IEEE/CVF Conference on Computer Vision and Pattern Recognition; 2019; Long Beach, CA, USA. p. 12026–35. doi:10.1109/CVPR.2019.01230. [Google Scholar] [CrossRef]
14. Zhang P, Lan C, Zeng W, Xing J, Xue J, Zheng N. Semantics-guided neural networks for efficient skeleton-based human action recognition. In: Proceedings of the IEEE/CVF Conference on Computer Vision and Pattern Recognition; 2020; Seattle, WA, USA. p. 1112–21. doi:10.1109/CVPR42600.2020.00119. [Google Scholar] [CrossRef]
15. Ye F, Pu S, Zhong Q, Li C, Xie D, Tang H. Dynamic GCN: context-enriched topology learning for skeleton-based action recognition. In: Proceedings of the 28th ACM International Conference on Multimedia; 2020; Seattle, WA, USA. p. 55–63. doi:10.1145/3394171.341394. [Google Scholar] [CrossRef]
16. Nasir IM, Raza M, Shah JH, Wang S-H, Tariq U, Khan MA. HaredNet: a deep learning based architecture for autonomous video surveillance by recognizing human actions. Comput Electr Eng. 2022;99:107805. doi:10.1016/j.compeleceng.2022.107805. [Google Scholar] [CrossRef]
17. Liu X, Shi H, Chen H, Yu Z, Li X, Zhao G. IMiGUE: an identity-free video dataset for micro-gesture understanding and emotion analysis. In: Proceedings of the IEEE/CVF Conference on Computer Vision and Pattern Recognition; 2021; Nashville, TN, USA. p. 10 631–42. doi:10.1109/CVPR46437.2021.01049. [Google Scholar] [CrossRef]
18. Kay W, Carreira J, Simonyan K, Zhang B, Hillier C, Vijayanarasimhan S, et al. The kinetics human action video dataset. 2017. doi:10.48550/arXiv.1705.06950. [Google Scholar] [CrossRef]
19. Niepert M, Ahmed M, Kutzkov K. Learning convolutional neural networks for graphs. In: International Conference on Machine Learning; 2016; New York, NY, USA: PMLR. p. 2014–23. doi:10.48550/arXiv.1605.05273. [Google Scholar] [CrossRef]
20. Monti F, Boscaini D, Masci J, Rodola E, Svoboda J, Bronstein MM. Geometric deep learning on graphs and manifolds using mixture model CNNs. In: Proceedings of the IEEE Conference on Computer Vision and Pattern Recognition; 2017; Honolulu, HI, USA. p. 5115–24. doi:10.1109/CVPR.2017.576. [Google Scholar] [CrossRef]
21. Hamilton W, Ying Z, Leskovec J. Inductive representation learning on large graphs. Adv Neural Inform Process Syst. 2017;30. doi:10.48550/arXiv.1706.02216. [Google Scholar] [CrossRef]
22. Bruna J, Zaremba W, Szlam A, LeCun Y. Spectral networks and locally connected networks on graphs. 2013. doi:10.48550/arXiv.1312.6203. [Google Scholar] [CrossRef]
23. Duvenaud DK, Maclaurin D, Iparraguirre J, Bombarell R, Hirzel T, Aspuru-Guzik A, et al. Convolutional networks on graphs for learning molecular fingerprints. Adv Neural Inform Process Syst. 2015;28. doi:10.48550/arXiv.1509.09292. [Google Scholar] [CrossRef]
24. Defferrard M, Bresson X, Vandergheynst P. Convolutional neural networks on graphs with fast localized spectral filtering. Adv Neural Inform Process Syst. 2016;29. doi:10.48550/arXiv.1606.09375. [Google Scholar] [CrossRef]
25. Kipf TN, Welling M. Semi-supervised classification with graph convolutional networks. 2016. doi:10.48550/arXiv.1609.02907. [Google Scholar] [CrossRef]
26. Velickovic P, Cucurull G, Casanova A, Romero A, Lio P, Bengio Y, et al. Graph attention networks. Statistics. 2017;1050(20):10–48 550. doi:10.48550/arXiv.1710.10903. [Google Scholar] [CrossRef]
27. Gao H, Wang Z, Ji S. Large-scale learnable graph convolutional networks. In: Proceedings of the 24th ACM SIGKDD International Conference on Knowledge Discovery & Data Mining; 2018; New York, NY, USA: Association for Computing Machinery. p. 1416–24. doi:10.1145/3219819.3219947. [Google Scholar] [CrossRef]
28. Xia H, Gao X. Multi-scale mixed dense graph convolution network for skeleton-based action recognition. IEEE Access. 2021;9:36 475–84. doi:10.1109/ACCESS.2020.3049029. [Google Scholar] [CrossRef]
29. Zhao R, Wang K, Su H, Ji Q. Bayesian graph convolution LSTM for skeleton based action recognition. In: Proceedings of the IEEE/CVF International Conference on Computer Vision; 2019; Seoul, Republic of Korea. p. 6882–92. doi:10.1109/ICCV.2019.00698. [Google Scholar] [CrossRef]
30. Tang Y, Tian Y, Lu J, Li P, Zhou J. Deep progressive reinforcement learning for skeleton-based action recognition. In: Proceedings of the IEEE Conference on Computer Vision and Pattern Recognition; 2018; Salt Lake City, UT, USA. p. 5323–32. doi:10.1109/CVPR.2018.00558. [Google Scholar] [CrossRef]
31. Cheng K, Zhang Y, He X, Chen W, Cheng J, Lu H. Skeleton-based action recognition with shift graph convolutional network. In: Proceedings of the IEEE/CVF Conference on Computer Vision and Pattern Recognition; 2020; Seattle, WA, USA. p. 183–92. doi:10.1109/CVPR42600.2020.00026. [Google Scholar] [CrossRef]
32. Li S, Yi J, Farha YA, Gall J. Pose refinement graph convolutional network for skeleton-based action recognition. IEEE Robot Autom Lett. 2021;6(2):1028–35. doi:10.1109/LRA.2021.3056361. [Google Scholar] [CrossRef]
33. Chen Y, Zhang Z, Yuan C, Li B, Deng Y, Hu W. Channel-wise topology refinement graph convolution for skeleton-based action recognition. In: Proceedings of the IEEE/CVF International Conference on Computer Vision; 2021; Montreal, QC, Canada. p. 13 359–68. doi:10.1109/ICCV48922.2021.01311. [Google Scholar] [CrossRef]
34. Cheng K, Zhang Y, Cao C, Shi L, Cheng J, Lu H. Decoupling GCN with dropgraph module for skeleton-based action recognition. In: Computer Vision-ECCV 2020: 16th European Conference; 2020 Aug 23–28; Glasgow, UK: Springer. p. 536–53. doi:10.1007/978-3-030-58586-0_32. [Google Scholar] [CrossRef]
35. Pang C, Lu X, Lyu L. Skeleton-based action recognition through contrasting two-stream spatial-temporal networks. IEEE Trans Multimedia. 2023;25:8699–711. doi:10.1109/TMM.2023.3239751. [Google Scholar] [CrossRef]
36. Lee J, Lee M, Lee D, Lee S. Hierarchically decomposed graph convolutional networks for skeleton-based action recognition. In: Proceedings of the IEEE/CVF International Conference on Computer Vision; 2023; Paris, France. p. 10444–53. doi:10.1109/ICCV51070.2023.00958. [Google Scholar] [CrossRef]
37. Wang S, Pan J, Huang B, Liu P, Li Z, Zhou C. ICE-GCN: an interactional channel excitation-enhanced graph convolutional network for skeleton-based action recognition. Mach Vision Appl. 2023;34(3):40. doi:10.1007/s00138-023-01386-2. [Google Scholar] [CrossRef]
38. Liu Y, Zhang H, Li Y, He K, Xu D. Skeleton-based human action recognition via large-kernel attention graph convolutional network. IEEE Trans Vis Comput Graph. 2023;29(5):2575–85. doi:10.1109/TVCG.2023.3247075. [Google Scholar] [PubMed] [CrossRef]
39. Cao Z, Simon T, Wei S-E, Sheikh Y. Realtime multi-person 2D pose estimation using part affinity fields. In: Proceedings of the IEEE Conference on Computer Vision and Pattern Recognition; 2017; Honolulu, HI, USA. p. 7291–9. doi:10.1109/CVPR.2017.143. [Google Scholar] [CrossRef]
40. Shi H, Liu X, Hong X, Zhao G. Bidirectional long short-term memory variational autoencoder. In: Proceedings of the British Machine Vision Conference 2018 (BMVC); 2018 Sep 3rd–6th; Newcastle UK: Bmva Press. [Google Scholar]
41. Peng W, Hong X, Chen H, Zhao G. Learning graph convolutional network for skeleton-based human action recognition by neural searching. Proc AAAI Conf Artif Intell. 2020;34(3):2669–76. doi:10.1609/aaai.v34i03.5652. [Google Scholar] [CrossRef]
42. Liu Z, Zhang H, Chen Z, Wang Z, Ouyang W. Disentangling and unifying graph convolutions for skeleton-based action recognition. In: Proceedings of the IEEE/CVF Conference on Computer Vision and Pattern Recognition; 2020; Seattle, WA, USA. p. 143–52. doi:10.1109/CVPR42600.2020.00022. [Google Scholar] [CrossRef]
43. Duan H, Wang J, Chen K, Lin D. DG-STGCN: dynamic spatial-temporal modeling for skeleton-based action recognition. 2022. doi:10.48550/arXiv.2210.05895. [Google Scholar] [CrossRef]
44. Duan H, Wang J, Chen K, Lin D. PYSKL: towards good practices for skeleton action recognition. In: Proceedings of the 30th ACM International Conference on Multimedia; 2022; New York, NY, USA: Association for Computing Machinery. p. 7351–4. doi:10.1145/3503161.3548546. [Google Scholar] [CrossRef]
45. Hara K, Kataoka H, Satoh Y. Can spatiotemporal 3D CNNs retrace the history of 2D CNNs and imagenet?. In: Proceedings of the IEEE Conference on Computer Vision and Pattern Recognition; 2018; Salt Lake City, UT, USA. p. 6546–55. doi:10.1109/CVPR.2018.00685. [Google Scholar] [CrossRef]
46. Carreira J, Zisserman A. Quo vadis, action recognition? a new model and the kinetics dataset. In: Proceedings of the IEEE Conference on Computer Vision and Pattern Recognition; 2017; Honolulu, HI, USA. p. 6299–308. doi:10.1109/CVPR.2017.502. [Google Scholar] [CrossRef]
47. Tran D, Bourdev L, Fergus R, Torresani L, Paluri M. Learning spatiotemporal features with 3D convolutional networks. In: Proceedings of the IEEE International Conference on Computer Vision; 2015; Santiago, Chile. p. 4489–97. doi:10.1109/ICCV.2015.510. [Google Scholar] [CrossRef]
48. Cho S, Maqbool M, Liu F, Foroosh H. Self-attention network for skeleton-based human action recognition. In: Proceedings of the IEEE/CVF Winter Conference on Applications of Computer Vision; 2020; Snowmass Village, Aspen, CO, USA. p. 635–44. doi:10.1109/WACV45572.2020.9093639. [Google Scholar] [CrossRef]
49. Li M, Chen S, Chen X, Zhang Y, Wang Y, Tian Q. Actional-structural graph convolutional networks for skeleton-based action recognition. In: Proceedings of the IEEE/CVF Conference on Computer Vision and Pattern Recognition; 2019; Long Beach, CA, USA. p. 3595–603. doi:10.1109/CVPR.2019.00371. [Google Scholar] [CrossRef]
50. Zhu G, Zhang L, Li H, Shen P, Shah SAA, Bennamoun M. Topology-learnable graph convolution for skeleton-based action recognition. Pattern Recognit Lett. 2020;135:286–92. doi:10.1016/j.patrec.2020.05.005. [Google Scholar] [CrossRef]
51. Song S, Lan C, Xing J, Zeng W, Liu J. An end-to-end spatio-temporal attention model for human action recognition from skeleton data. Proc AAAI Conf Artif Intell. 2017;31(1). doi:10.1609/aaai.v31i1.11212. [Google Scholar] [CrossRef]
52. Ke Q, Bennamoun M, An S, Sohel F, Boussaid F. A new representation of skeleton sequences for 3D action recognition. In: Proceedings of the IEEE Conference on Computer Vision and Pattern Recognition; 2017; Honolulu, HI, USA. p. 3288–97. doi:10.1109/CVPR.2017.486. [Google Scholar] [CrossRef]
53. Zhang P, Xue J, Lan C, Zeng W, Gao Z, Zheng N. EleAtt-RNN: adding attentiveness to neurons in recurrent neural networks. IEEE Trans Image Process. 2019;29:1061–73. doi:10.1109/TIP.2019.2937724. [Google Scholar] [PubMed] [CrossRef]
54. Liu M, Liu H, Chen C. Enhanced skeleton visualization for view invariant human action recognition. Pattern Recognit. 2017;68(3):346–62. doi:10.1016/j.patcog.2017.02.030. [Google Scholar] [CrossRef]
55. Song Y-F, Zhang Z, Wang L. Richly activated graph convolutional network for action recognition with incomplete skeletons. In: 2019 IEEE International Conference on Image Processing (ICIP); 2019; Taipei, Taiwan: IEEE. p. 1–5. doi:10.1109/ICIP.2019.8802917. [Google Scholar] [CrossRef]
Cite This Article
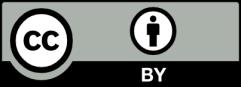
This work is licensed under a Creative Commons Attribution 4.0 International License , which permits unrestricted use, distribution, and reproduction in any medium, provided the original work is properly cited.