Open Access
ARTICLE
Skeleton-Based Action Recognition Using Graph Convolutional Network with Pose Correction and Channel Topology Refinement
1 School of Engineering, Guangzhou College of Technology and Business, Foshan, 528138, China
2 School of Computer Science and Engineering, Dalian Minzu University, Dalian, 116000, China
3 SEAC key Laboratory of Big Data Applied Technology, Dalian Minzu University, Dalian, 116000, China
* Corresponding Author: Qiguo Dai. Email:
(This article belongs to the Special Issue: Advances in Action Recognition: Algorithms, Applications, and Emerging Trends)
Computers, Materials & Continua 2025, 83(1), 701-718. https://doi.org/10.32604/cmc.2025.060137
Received 25 October 2024; Accepted 25 December 2024; Issue published 26 March 2025
Abstract
Graph convolutional network (GCN) as an essential tool in human action recognition tasks have achieved excellent performance in previous studies. However, most current skeleton-based action recognition using GCN methods use a shared topology, which cannot flexibly adapt to the diverse correlations between joints under different motion features. The video-shooting angle or the occlusion of the body parts may bring about errors when extracting the human pose coordinates with estimation algorithms. In this work, we propose a novel graph convolutional learning framework, called PCCTR-GCN, which integrates pose correction and channel topology refinement for skeleton-based human action recognition. Firstly, a pose correction module (PCM) is introduced, which corrects the pose coordinates of the input network to reduce the error in pose feature extraction. Secondly, channel topology refinement graph convolution (CTR-GC) is employed, which can dynamically learn the topology features and aggregate joint features in different channel dimensions so as to enhance the performance of graph convolution networks in feature extraction. Finally, considering that the joint stream and bone stream of skeleton data and their dynamic information are also important for distinguishing different actions, we employ a multi-stream data fusion approach to improve the network’ s recognition performance. We evaluate the model using top-1 and top-5 classification accuracy. On the benchmark datasets iMiGUE and Kinetics, the top-1 classification accuracy reaches 55.08% and 36.5%, respectively, while the top-5 classification accuracy reaches 89.98% and 59.2%, respectively. On the NTU RGB+D dataset, for the two benchmark settings (X-Sub and X-View), the classification accuracy achieves 89.7% and 95.4%, respectively.Keywords
Cite This Article
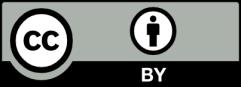
This work is licensed under a Creative Commons Attribution 4.0 International License , which permits unrestricted use, distribution, and reproduction in any medium, provided the original work is properly cited.