Open Access
ARTICLE
A Federated Learning Incentive Mechanism for Dynamic Client Participation: Unbiased Deep Learning Models
1 School of Computer Science and Technology, Wuhan University of Science and Technology, Wuhan, 430065, China
2 College of Computer Science, South-Central Minzu University, Wuhan, 430074, China
3 School of Computer Science and Technology, Zhejiang Normal University, Jinhua, 321004, China
* Corresponding Author: Yuanai Xie. Email:
(This article belongs to the Special Issue: The Next-generation Deep Learning Approaches to Emerging Real-world Applications)
Computers, Materials & Continua 2025, 83(1), 619-634. https://doi.org/10.32604/cmc.2025.060094
Received 23 October 2024; Accepted 03 January 2025; Issue published 26 March 2025
Abstract
The proliferation of deep learning (DL) has amplified the demand for processing large and complex datasets for tasks such as modeling, classification, and identification. However, traditional DL methods compromise client privacy by collecting sensitive data, underscoring the necessity for privacy-preserving solutions like Federated Learning (FL). FL effectively addresses escalating privacy concerns by facilitating collaborative model training without necessitating the sharing of raw data. Given that FL clients autonomously manage training data, encouraging client engagement is pivotal for successful model training. To overcome challenges like unreliable communication and budget constraints, we present ENTIRE, a contract-based dynamic participation incentive mechanism for FL. ENTIRE ensures impartial model training by tailoring participation levels and payments to accommodate diverse client preferences. Our approach involves several key steps. Initially, we examine how random client participation impacts FL convergence in non-convex scenarios, establishing the correlation between client participation levels and model performance. Subsequently, we reframe model performance optimization as an optimal contract design challenge to guide the distribution of rewards among clients with varying participation costs. By balancing budget considerations with model effectiveness, we craft optimal contracts for different budgetary constraints, prompting clients to disclose their participation preferences and select suitable contracts for contributing to model training. Finally, we conduct a comprehensive experimental evaluation of ENTIRE using three real datasets. The results demonstrate a significant 12.9% enhancement in model performance, validating its adherence to anticipated economic properties.Keywords
Cite This Article
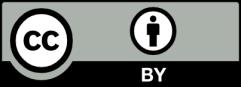
This work is licensed under a Creative Commons Attribution 4.0 International License , which permits unrestricted use, distribution, and reproduction in any medium, provided the original work is properly cited.