Open Access
ARTICLE
A Low-Collision and Efficient Grasping Method for Manipulator Based on Safe Reinforcement Learning
China Institute of FTZ Supply Chain, Shanghai Maritime University, Shanghai, 201306, China
* Corresponding Author: Bai Hu. Email:
Computers, Materials & Continua 2025, 83(1), 1257-1273. https://doi.org/10.32604/cmc.2025.059955
Received 21 October 2024; Accepted 06 February 2025; Issue published 26 March 2025
Abstract
Grasping is one of the most fundamental operations in modern robotics applications. While deep reinforcement learning (DRL) has demonstrated strong potential in robotics, there is too much emphasis on maximizing the cumulative reward in executing tasks, and the potential safety risks are often ignored. In this paper, an optimization method based on safe reinforcement learning (Safe RL) is proposed to address the robotic grasping problem under safety constraints. Specifically, considering the obstacle avoidance constraints of the system, the grasping problem of the manipulator is modeled as a Constrained Markov Decision Process (CMDP). The Lagrange multiplier and a dynamic weighted mechanism are introduced into the Proximal Policy Optimization (PPO) framework, leading to the development of the dynamic weighted Lagrange PPO (DWL-PPO) algorithm. The behavior of violating safety constraints is punished while the policy is optimized in this proposed method. In addition, the orientation control of the end-effector is included in the reward function, and a compound reward function adapted to changes in pose is designed. Ultimately, the efficacy and advantages of the suggested method are proved by extensive training and testing in the Pybullet simulator. The results of grasping experiments reveal that the recommended approach provides superior safety and efficiency compared with other advanced RL methods and achieves a good trade-off between model learning and risk aversion.Keywords
Cite This Article
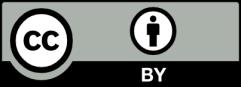
This work is licensed under a Creative Commons Attribution 4.0 International License , which permits unrestricted use, distribution, and reproduction in any medium, provided the original work is properly cited.