Open Access
ARTICLE
Image Super-Resolution Reconstruction Based on the DSSTU-Net Model
1 School of Electronic Engineering and Automation, Guilin University of Electronic Technology, Guilin, 541004, China
2 School of Architecture and Transportation Engineering, Guilin University of Electronic Technology, Guilin, 541004, China
3 Key Laboratory of Intelligence Integrated Automation in Guangxi Universities, Guilin, 541004, China
* Corresponding Author: Peng Sun. Email:
(This article belongs to the Special Issue: Computer Vision and Image Processing: Feature Selection, Image Enhancement and Recognition)
Computers, Materials & Continua 2025, 83(1), 1057-1078. https://doi.org/10.32604/cmc.2025.059946
Received 21 October 2024; Accepted 21 January 2025; Issue published 26 March 2025
Abstract
Super-resolution (SR) reconstruction addresses the challenge of enhancing image resolution, which is critical in domains such as medical imaging, remote sensing, and computational photography. High-quality image reconstruction is essential for enhancing visual details and improving the accuracy of subsequent tasks. Traditional methods, including interpolation techniques and basic CNNs, often fail to recover fine textures and detailed structures, particularly in complex or high-frequency regions. In this paper, we present Deep Supervised Swin Transformer U-Net (DSSTU-Net), a novel architecture designed to improve image SR by integrating Residual Swin Transformer Blocks (RSTB) and Deep Supervision (DS) mechanisms into the U-Net framework. DSSTU-Net leverages the Swin Transformer’s multi-scale attention capabilities for robust feature extraction, while DS at various stages of the network ensures better gradient propagation and refined feature learning. The ST block introduces a hierarchical self-attention mechanism, allowing the model to capture both local and global context, which is crucial for high-quality SR tasks. Moreover, DS applied at multiple layers in the decoder enables direct supervision on intermediate feature maps, accelerating convergence and improving performance. The DSSTU-Net architecture was rigorously evaluated on the DIV2K, LSDIR, SET5, and SET14 datasets, demonstrating its superior ability to generate high-quality images. Furthermore, the potential applications of this model extend beyond image enhancement, with promising use cases in medical imaging, satellite imagery, and industrial inspection, where high-quality image reconstruction plays a crucial role in accurate diagnostics and operational efficiency. This work provides a reference method for future research on advanced image restoration techniques.Keywords
SR image reconstruction is a vital area within computer vision that addresses the critical challenge of recovering high-resolution (HR) images from low-resolution (LR) inputs [1,2]. This issue holds significant implications across various domains, including medical imaging, satellite photography, and security systems, where clarity and detail are paramount [3]. The escalating demand for high-quality visual data has spurred extensive research into SR methods. In particular, deep learning techniques have demonstrated unprecedented advancements over traditional approaches, marking a pivotal shift in the field.
Traditional SR techniques, such as bicubic interpolation and patch-based methods, frequently struggle to recover fine details, particularly in images characterized by complex textures or high-frequency components [4]. These methods often rely on handcrafted features or statistical assumptions that do not generalize well across diverse image domains. Conversely, deep learning approaches, especially CNNs, have revolutionized SR tasks by effectively learning complex hierarchical features from data, thereby outperforming classical methods [5]. However, many CNN-based approaches still encounter limitations in reconstructing intricate details and maintaining global context, as they primarily operate on fixed-sized receptive fields.
Recent advancements in transformer architecture, notably the Swin Transformer, have significantly enhanced the capabilities of SR models [6]. Transformers excel at capturing long-range dependencies and global context-attributes that CNNs struggle to encompass. The Swin Transformer introduces a hierarchical structure with local-global attention mechanisms, enabling it to adeptly manage both local details and broader contextual information. This makes it exceptionally suitable for SR tasks, where the recovery of fine textures and overall image coherence is critical.
However, existing transformer-based SR models, such as SwinIR and MUN, still have notable limitations. SwinIR—while effective in capturing global features—struggles with fine detail recovery in high-frequency regions, as its attention mechanism is not always able to maintain the delicate balance between local texture recovery and global consistency. Additionally, SwinIR often faces computational overhead due to the large model size and high memory usage required for processing multi-scale attention. On the other hand, MUN improves local feature extraction by using multiple sub-networks but tends to lose global context and fine-grained details in complex images, limiting its effectiveness in high-resolution recovery tasks.
To address these challenges, we propose the DSSTU-Net model, specifically designed to tackle key issues in SR reconstruction. The DSSTU-Net model integrates the RSTB, which leverage multiscale attention mechanisms within a U-Net architecture renowned for its powerful feature fusion capabilities through skip connections. This combination ensures both fine detail recovery and global feature aggregation, thereby enhancing overall reconstruction quality. Furthermore, the inclusion of DS in the decoder phase bolsters gradient flow during training, accelerating convergence and enabling the model to capture richer, multilevel feature representations.
This research contributes to the broader field of SR by:
1. Novel Architectural Integration: Proposing a unique combination of U-Net and Swin Transformer architectures that synergistically enhance both detail recovery and contextual understanding, allowing the model to effectively manage varying scales of information.
2. Introducing the RSTB, which employs multiscale attention mechanisms to capture intricate details and long-range dependencies, significantly improving the model’s ability to reconstruct high-frequency content.
3. Implementing a DS strategy in the decoder phase that improves gradient flow and accelerates convergence during training. The DSSTU-Net model provides a reference method for future research on advanced image restoration techniques.
The significance of this research lies in its potential to advance HR image reconstruction, particularly in applications where the recovery of fine details is crucial. Consequently, the DSSTU-Net model provides a reference method for future research on advanced image restoration techniques.
SR is a class of techniques that enhance the resolution of an imaging system. The primary aim of super-resolution technologies is to reconstruct HR images from their LR counterparts. This is crucial in various applications such as satellite imaging, medical imaging, and consumer electronics where enhancing image quality can significantly impact the output and usability of the images.
The seminal work by Yu et al. [7] serves as a foundational reference for our study. Their approach to seamlessly integrating learning-based and reconstruction-based methods to enhance single-image super-resolution has informed the development of our framework. By employing a unified dictionary learned directly from the low-resolution input and integrating advanced filtering techniques, their methodology provides crucial insights into achieving high-quality super-resolution without the introduction of artifacts, which has significantly influenced our own methodological enhancements.
Han et al. [8] presented a multi-level U-Net residual structure, composed of two different levels of U-Net structures, to extract multi-level features from LR images. Meanwhile, they presented a multi-scale residual block that extracts multi-level features through dual-branch convolutional layers with different kernels and uses both long and short skip connections to bypass a large amount of low-frequency information. Extensive experimental results demonstrate that their MUN outperforms other state-of-the-art super-resolution methods.
Liang et al. [9] proposed a strong baseline model, SwinIR, for image restoration based on the Swin Transformer. SwinIR consists of three parts: shallow feature extraction, deep feature extraction, and high-quality image reconstruction. Particularly, the deep feature extraction module is composed of several RSTB, each containing multiple Swin Transformer layers along with a residual connection. They conducted experiments on image super-resolution, and the results demonstrate that SwinIR outperforms state-of-the-art methods on various tasks by up to 0.45 dB, while the total number of parameters can be reduced by up to 67%.
Chen et al. [10] shifted their focus to the frequency domain since the natural global properties of the frequency domain can address this issue. To explore attention maps from a frequency domain perspective, they investigated and corrected some misconceptions in existing frequency domain feature processing methods and proposed a new frequency domain attention mechanism called frequency-enhanced pixel attention (FPA). Additionally, they used large kernel convolutions and partial convolutions to improve the ability to extract deep features while maintaining a lightweight design. Based on these improvements, they proposed a large kernel frequency-enhanced network (LKFN) with a smaller model size and higher computational efficiency. It effectively captures long-range dependencies between pixels in a whole image and achieves state-of-the-art performance in existing efficient super-resolution methods.
Li et al. [11] proposed a Multi-scale Dual-Attention based Residual Dense Generative Adversarial Network (MARDGAN), which uses multi-branch paths to extract image features and obtain multi-scale feature information. They also designed the channel and spatial attention block (CSAB), which is combined with the enhanced residual dense block (ERDB) to extract multi-level depth feature information and enhance feature reuse. In addition, the multi-scale feature information extracted under the three-branch path is fused with global features and sub-pixel convolution is used to restore the high-resolution image. The experimental results show that the objective evaluation index of MARDGAN on multiple benchmark datasets is higher than that of other methods, and the subjective visual effect is also better.
The method of image super-resolution reconstruction through the dictionary typically uses only a single-layer dictionary, which not only fails to extract deep features of the image but also requires a large trained dictionary to achieve better reconstruction effects. Huang et al. [12] proposed a new deep dictionary learning model. Firstly, after preprocessing the images in the training set, the dictionary is trained using the deep dictionary learning method, and the adjusted anchored neighborhood regression method is used for image super-resolution reconstruction. The proposed algorithm was compared with several classical algorithms on the Set5 and Set14 datasets. The visualization and quantification results show that the proposed method improves PSNR and SSIM, effectively reduces the dictionary size, and saves reconstruction time compared with traditional super-resolution algorithms.
Despite the impressive strides made in SR technology, certain limitations persist that must be addressed to further refine the performance and applicability of SR systems. Moreover, while current SR methods excel at upscaling images with moderate upscaling factors, their efficacy diminishes as the factor increases, particularly for complex textures and fine details. This limitation underscores the need for models that can maintain robust performance even at high magnification levels without compromising on detail or introducing artifacts. Additionally, the reliance on vast amounts of high-quality training data for optimal performance poses a barrier, especially in domains where such data are scarce or expensive to procure. The exploration of novel attention mechanisms and network architectures that focus more on feature selection and optimization may provide new pathways to enhance the quality of super-resolved images.
This study proposes the DSSTU-Net model for efficient image super-resolution reconstruction tasks. The model combines DS and the Swin Transformer architecture to improve the conversion effect of LQ to HQ images through multi-scale feature extraction and detail reconstruction. The model structure is shown in Fig. 1.
Figure 1: The DSSTU-Net model
The DSSTU-Net model uses a symmetric network based on the U-Net structure, including modules such as down-sampling, up-sampling, and skip connections to ensure that global context information and local details are preserved during the reconstruction process [13]. The most significant improvement is the use of RSTB as the main feature extraction unit. Each RSTB strengthens feature learning through a residual structure and uses the local attention mechanism of the Swin Transformer to enhance the modeling ability of image details and edges. The model improves the feedback of the training process by introducing DS modules at multiple feature scales. DS not only adds supervision signals to the output layer but also introduces a loss function in the intermediate feature layer so that the network can optimize the feature representation of different scales layer by layer during the learning process, promoting higher-quality image reconstruction.
The DSSTU-Net model consists of multiple modules, each of which plays a specific role in the image super-resolution reconstruction task. The following steps describe the functions and roles of each module in detail:
1. Input: The input to the model is an LQ image, typically derived from a low-resolution image. It undergoes feature extraction through a convolutional layer, mapping the image from pixel to feature space for subsequent processing and analysis.
2. Convolution Layer (Conv): The convolutional layer is used to extract local low-level features of the image, such as edges, textures, and more. It employs a standard 3 × 3 convolutional kernel to extract features from the input image and passes the output to the subsequent RSTB module. The convolution operation converts the image into multiple feature maps, which serve as the input for the following modules.
3. RSTB module: The RSTB module is responsible for extracting and processing deep features. It combines the residual connection with the attention mechanism of the Swin Transformer to capture both local and global features of the image. The residual connection in the RSTB module helps alleviate the gradient vanishing problem and ensures that the model can learn deeper information through skip connections. The Swin Transformer extracts features in local areas and captures long-range dependencies through the multi-head self-attention mechanism of the sliding window, enhancing both the retention of image details and the capture of global information.
4. STL module (Swin Transformer Layer): Each RSTB module consists of multiple STLs, which can extract local features in-depth and detail, enhancing the model’s perception of subtle image information. The STL module employs a multi-head self-attention mechanism (MSA) and a feedforward neural network (MLP) to improve the image’s detail and global feature extraction capabilities through layer normalization (Layer Norm) and residual connections, ultimately enhancing the quality of super-resolution.
5. Skip connection: Skip connections transfer features between the encoder and decoder of the network, helping to retain the low-level features of the input image and preventing the loss of important information during high-level feature extraction. By directly connecting the features from the encoding stage to the decoding stage, the corresponding features are fused, effectively improving detail restoration and ensuring that the generated high-resolution image contains both global information and ample details.
6. Up-sampling and Down-sampling: Down-sampling is used to reduce the resolution of the image to capture global information while up-sampling is used to gradually restore the resolution of the image and restore details. Up-sampling and down-sampling operations are performed through convolution or other interpolation methods so that the model can not only process the global features of low-resolution images but also restore their details during up-sampling.
7. Supervision module: Supervision modules are applied at multiple levels of the network, and multi-level optimization is achieved by comparing the output with labeled images, enhancing both the training effect and the model’s convergence speed. The DS module is applied not only at the network’s output layer but also introduces loss functions at intermediate levels to gradually optimize features.
8. Output: The output is a reconstructed HQ image, and its goal is to have a high-resolution image that is as close to the target as possible. The extracted high-dimensional features are mapped back to the pixel space through the final convolution layer to generate high-quality images.
Through the seamless integration of these modules, the DSSTU-Net model effectively enhances the SR reconstruction of images, retains more detailed information, and ensures the efficiency and robustness of the training process.
The RSTB module is derived from the Swin Transformer architecture [14]. Its core concept is to extract image features through a local windowed MSA mechanism. Unlike traditional convolution operations, the Swin Transformer divides the image into blocks by partitioning local windows and applying the self-attention mechanism within each window. This approach captures both local details and long-range dependencies simultaneously, thereby enhancing the model’s ability to retain high-frequency information. The RSTB module is in the lower half of Fig. 1.
Specifically, the RSTB module combines residual connections and multiple STL structures to ensure information flow in the deep network while avoiding the gradient vanishing problem. The residual structure allows features to be accumulated and fed back within the module, thereby enhancing the flow of information and the ability to reconstruct image details. The STL structure is in the lower half of Fig. 1.
The STL module processes image features through the self-attention mechanism within Windows and ensures global information transmission by shifting windows during interactions between them. STL combines MSA, Layer Norm, and a feedforward neural network to capture both local and global features of the image.
Before performing each self-attention operation, the Layer Norm normalizes the input features to ensure stability in the input distribution. Next, the model divides the input image features into multiple windows, and STL applies self-attention within each window to capture local information.
(1)
Q, K, V represents query, key, and value matrices, respectively, and is a scaling factor used to avoid excessive dot product results. Through this attention mechanism, the relationship between pixels in each window is learned to capture local image features.
In the STL module, after the self-attention mechanism in the window, there will be a residual connection to add the input features to the features after the self-attention operation.
(2)
Then, the features after self-attention are further processed by a MLP to enhance the nonlinear mapping ability of the model.
(3)
and are weight matrices; are bias terms; is the activation function.
Finally, the output of the feedforward network is added to the initial input through a residual connection to keep the information flowing and avoid the gradient vanishing problem. The role of the residual connection is to add the input directly to the output.
(4)
The STL module includes two residual connections, one after the self-attention module and one after the feedforward network, to ensure smooth feature transmission across different processing stages, maximize the retention of key information, and enhance the model’s generalization ability.
The RSTB module combines residual connections with the self-attention mechanism of the Swin Transformer to effectively capture both local and global features of the image, improving the performance of super-resolution reconstruction.
DS plays a crucial role in enhancing the performance of deep neural network models by strategically placing supervision signals within the hidden layers [15]. This method addresses common challenges in deep learning, such as gradient vanishing and slow convergence, particularly in very deep architectures. By guiding intermediate layers directly with the ground truth, DS ensures more effective and faster learning. Fig. 2 illustrates the DS strategy.
Figure 2: The DS strategy illustrates the structure of a deep learning model’s feature extraction and prediction pipeline. (a) shows the feature extraction process; (b) depicts the Multi-level supervision unit; (c) represents the Final prediction phase
The DS strategy can be articulated through three distinct modules, each designed to refine feature processing and enhance the overall learning dynamics:
(1) Feature Extraction: Feature Extraction includes Average Pooling, Conv, ReLU Activation.
The Feature Extraction module in the DS strategy can be formalized as Eq. (5). Average Pooling reduces the spatial dimensions of the input feature map by summarizing features within a local neighborhood , leading to a more generalized feature representation.
(5)
The Feature Extraction module helps in decreasing sensitivity to the exact locations of features within the input, thereby providing a more generalized feature representation.
Conv applies various filters to the pooled features to extract significant attributes, represented by:
(6)
where denotes the convolution operation, and is the bias. This step is crucial for capturing essential patterns that are indicative of underlying structures in the data.
ReLU Activation introduces non-linearity to the processed features, enabling the model to capture more complex patterns and dependencies in the data.
(7)
ReLU Activation function is fundamental for enhancing the model’s capability to learn and adapt from nonlinear data.
(2) Multi-level Supervision Unit: The Multi-level Supervision Unit includes Dropout, ReLU Activation, and Inner Product.
Dropout acts as a regularization technique that randomly zeros out a portion of the feature detectors during training.
(8)
where is a mask generated from a Bernoulli distribution with probability . This prevents the model from relying too heavily on any single feature or a small group of features, promoting more robust learning and reducing overfitting. It also simulates ensemble learning by generating different thinned networks during each training pass.
ReLU Activation is reapplied to reintroduce non-linearity to the features after dropout, ensuring that the model’s capacity to capture complex functions remains intact despite the regularization.
The Inner Product performs a weighted summation of the inputs, serving as a linear transformation.
(9)
where represents the weight matrix, and is the bias. This step typically reduces dimensionality and helps formulate decisions, preparing the network for the final decision-making layer.
(3) Final Prediction: This final step aggregates the contributions from all the features processed through the supervision units to generate the final output prediction, which can be mathematically represented as Eq. (10):
(10)
where are the inputs from various layers, and and are learnable parameters specific to this aggregation phase. This summation is crucial as it integrates all the learned representations into a final decision, reflecting the cumulative understanding of the network about the input data.
Each component within the DS framework plays a pivotal role in enhancing the model’s performance. It not only improves the flow and adjustment of gradients throughout the network but also ensures that each layer contributes effectively to the final output. This structured approach enriches the model’s ability to reconstruct high-quality outputs from low-quality inputs.
Given that the model employs a DS module, each layer’s output applies the same cross-entropy loss function, adhering to Eqs. (11) and (12). The formula for the total loss is as follows:
(11)
(12)
represents the loss from the network’s final output, which is weighted at 0.8 to prioritize refining key feature information during training and to underscore the significance of the final output. denotes the loss from the output of the i-th intermediate layer, with the combined weight for these intermediate losses set at 0.2. This weighting scheme prevents these auxiliary losses from unduly influencing the primary task’s learning trajectory, while still contributing to effective feature extraction and gradient flow. is the weight of the i-th layer loss. The before each intermediate layer loss should ensure that so that the proportion of the total in the total loss remains 0.2.
The RSTB module in DSSTU-Net significantly enhances the model’s performance in super-resolution tasks by integrating advanced features from the Swin Transformer architecture. The RSTB module utilizes a local windowed MSA, enabling the model to effectively capture both local details and long-range dependencies. This method surpasses traditional convolution operations because it preserves high-frequency information that is crucial for high-quality image reconstruction. Residual connections within the RSTB module play a pivotal role in preserving the flow of information through deep network layers, preventing gradient vanishing, and ensuring consistent feature retention across the network. The integration of the STL within the RSTB structure incorporates two residual connections: one following the self-attention module and another after the feedforward network. This arrangement ensures seamless feature transmission across different processing stages, maximizes the retention of essential information, and enhances the model’s ability to integrate both local and broader image contexts effectively. By normalizing input features through Layer Norm before applying self-attention, the RSTB module ensures stability and consistent performance. This architecture, which combines the innovative Swin Transformer’s capacity for capturing both local and global features with robust residual connections, underpins DSSTU-Net’s superior ability to perform detailed and accurate super-resolution, outperforming models that rely on more traditional deep learning techniques.
The DS module significantly enhances DSSTU-Net by embedding supervision signals directly within its architecture, addressing common deep learning challenges like gradient vanishing and slow convergence. This method strategically applies ground truth guidance at multiple levels, ensuring each layer not only learns effectively but also contributes directly to the final output. This granular level of supervision results in faster and more effective learning processes, especially in deep network structures where traditional methods might falter. By integrating this with advanced feature extraction techniques such as average pooling, convolution, and ReLU activation, the module aids in crafting a nuanced, detail-rich output. This structured supervision not only refines the feature extraction across layers but also stabilizes the learning process, leading to superior performance in reconstructing high-quality images from low-quality inputs, thus significantly outperforming models lacking such intricate supervision frameworks.
These combined strategies not only advance the model’s ability to generate HR outputs from low-quality inputs but also reinforce the network’s overall learning process, demonstrating their critical roles in the success of SR architecture.
4 Experimental Datasets and Results
In this paper, we utilized four prominent datasets—DIV2K [16], LSDIR [17], Set5, and Set14—which are instrumental for training and benchmarking our DSSTU-Net model for image SR tasks. SET5 and SET14 are widely used benchmark datasets in super-resolution tasks, specifically designed for evaluating SR model performance. SET5 consists of 5 images, including diverse scenes like textures, human faces, and simple objects, while SET14 contains 14 more complex images, offering a broader scope for testing SR algorithms. Table 1 provides a detailed description of each dataset.
DIV2K is a well-established dataset widely used in image SR challenges. Its name comes from its resolution (approximately 2K), and it is recognized for its diverse image content, making it a robust dataset for training and validating SR models. The training set in DIV2K consists of 800 HR images rich in textures and details, ideal for training advanced deep-learning models. The validation set includes 100 HR images, used for periodic validation during training to monitor and evaluate the model’s performance. The testing set contains 100 HR images to evaluate the final model performance.
LSDIR is designed to offer a comprehensive resource for training models across various image restoration tasks, including SR, denoising, and more. It addresses the limitations of smaller datasets by providing an extensive collection of high-quality images. The training data in LSDIR consists of 84,991 high-definition, HR images, with corresponding LR versions for downscaling factors of 2, 3, and 4, offering substantial variety for robust training. The validation data includes 1000 high-definition HR images, with the LR counterparts intended for release to support more precise model validation. The test data comprises 1000 high-definition HR images, primarily used for benchmarking, with LR images available for downscaling factors of 2, 3, and 4 to enable comprehensive testing.
By leveraging the DIV2K and LSDIR datasets, the DSSTU-Net model can be meticulously trained and rigorously tested, ensuring strong performance across various settings and meeting the high standards required for practical applications in image SR.
To evaluate the performance of our DSSTU-Net model on the image SR task, we use two widely recognized metrics: Peak signal-to-noise ratio (PSNR) and Structural Similarity Index Measure (SSIM) [18]. These metrics are crucial for measuring the effectiveness of SR methods by comparing the similarity between the generated HR images and the original HR ground truths.
PSNR is a standard metric that quantifies the quality of reconstruction in imaging and video compression. It expresses the result on a logarithmic decibel scale, based on the MSE between the ground truth and the reconstructed image. Eq. (13) shows how to calculate PSNR.
(13)
where the MSE is calculated as Eq. (14):
(14)
is the maximum possible pixel value of the image; is the original HR image; K is the super-resolved image generated by the model; m and n are the dimensions of the images.
SSIM is another critical metric used to measure the perceived quality of digital images and videos. Unlike PSNR, SSIM considers changes in structural information, luminance, and contrast, making it more aligned with human visual perception. Eq. (15) shows how to calculate SSIM.
(15)
are the averages of x and y, respectively; is the covariance of x and y; , are the variances of x and y; , are constants to stabilize the division with a weak denominator; L is the dynamic range of the pixel-values; = 0.01 and = 0.03 are default values.
SSIM values range between −1 and 1, where 1 indicates perfect similarity. The SSIM index is designed to improve the perceptual relevance of the quality assessment by comparing local patterns of pixel intensities that have been normalized for luminance and contrast. It assesses the visual impact of three characteristics of an image: luminance, contrast, and structure, thus aligning more closely with the quality perception of the human visual system.
PSNR provides insights into the overall error rate and noise level, while SSIM offers a deeper understanding of the qualitative aspects of super-resolution images. It is crucial to fully evaluate the effectiveness of DSSTU-Net in practical applications by using both PSNR and SSIM.
We tested the performance of U-Net, TransU-Net, DSU-Net (DS + U-Net), STU-Net (Swin Transformer + U-Net), and DSSTU-Net using the same training, validation, and test sets. The data-splitting strategy followed the same approach as in the DIV2K and LSDIR competitions. The test datasets consist of the validation sets from DIV2K and LSDIR, along with Set5 and Set14. All models were trained on a computer equipped with an NVIDIA GeForce RTX 4090 D (24 GB) GPU, which provided the computational power needed for high-intensity training sessions. We set the initial learning rate to 0.0001, with the Adam optimizer used for adjusting the weights during training, where the beta parameters were set to (0.9, 0.999). This choice of optimizer and learning rate facilitates effective convergence to optimal weights while preventing stagnation in local minima. The training procedure utilized a batch size of 32 and employed 8 workers to optimize data loading and preprocessing, balancing computational load and speed. Training continued until no significant improvement in validation loss was observed, employing early stopping to avoid overfitting. Table 2 provides a comprehensive comparison of key computational metrics across four different super-resolution models (evaluated on a GeForce RTX 4090 D setup): U-Net, DSU-Net, STU-Net, and DSSTU-Net, evaluated on the DIV2K and LSDIR datasets. This table highlights the number of parameters and model size on the device, which are critical for understanding the models’ efficiency and deployment potential. Additionally, it includes training and testing times, offering insights into the practical application and operational efficiency of each model.
Table 3 provides a comprehensive comparison of SR performance across different scales (×2, ×3, ×4) on the DIV2K and LSDIR datasets using U-Net, DSU-Net, STU-Net, and DSSTU-Net. The metrics utilized for evaluation are PSNR and SSIM, which are standard for assessing image quality in SR tasks.
At scaling ×2, the DSSTU-Net model significantly outperforms other methods within the DIV2K dataset, achieving the highest PSNR and SSIM across all test sets (Set5, Set14, and Validation). When employing DIV2K as the training set and Set5 as the test, DSSTU-Net achieves a PSNR of 37.84, marking substantial improvements of 25.6% over U-Net, 15.2% over DSU-Net, and 9.8% over STU-Net. Similarly, employing LSDIR dataset as the training set and Set5 as the test, DSSTU-Net records a PSNR of 38.78, surpassing the scores of U-Net by 23.6%, DSU-Net by 10.3%, and STU-Net by 8.4%. Transitioning to the Set14 test dataset and DIV2K as the training set, DSSTU-Net continues to impress with a PSNR of 34.47, outperforming U-Net by 22.7%, DSU-Net by 12.8%, and STU-Net by 4.5%. On the LSDIR dataset as the training set, DSSTU-Net achieves a PSNR of 34.61, showing gains of 18.4% over U-Net, 9.7% over DSU-Net, and 4.4% over STU-Net. Furthermore, in the Validation dataset of DIV2K, DSSTU-Net posts a PSNR of 36.81, which is significantly higher than that of U-Net by 19.7%, DSU-Net by 9.5%, and STU-Net by 6.0%. Lastly, in the LSDIR dataset within the Validation set, DSSTU-Net’s PSNR of 36.22 reflects improvements of 15.4% over U-Net, 8.5% over DSU-Net, and 7.7% over STU-Net.
At scale ×3 across various datasets, DSSTU-Net continues to demonstrate its superior performance compared to U-Net, DSU-Net, and STU-Net, though the performance margins narrow as the complexity increases. When employing DIV2K as the training set and Set5 as the test, DSSTU-Net achieves a PSNR of 35.74, which is 20.1% higher than U-Net at 29.77 PSNR, and marginally better than STU-Net by 2.2%. This trend of DSSTU-Net’s superior capability extends across other datasets like Set14 and the validation sets. For instance, in Set14, DSSTU-Net slightly leads with a PSNR of 30.88 compared to STU-Net’s 30.73, showing a small but significant refinement in handling intricate details. Similarly, in the validation sets of DIV2K and LSDIR, DSSTU-Net outperforms STU-Net by 5% and 4.5%, respectively, which underscores its robust architectural design that efficiently handles upscaling tasks even at higher complexities. These improvements highlight DSSTU-Net’s sophisticated integration of Swin Transformers and DS mechanisms, enabling better detail preservation and texture handling across diverse conditions and datasets. However, the narrow margins at this scale suggest that the challenges of higher upscaling factors begin to test the limits of current technologies, including DSSTU-Net, which, while still leading, shows reduced dominance compared to lower scales.
At scale ×4, DSSTU-Net showcases a pronounced efficiency in handling the highest upscaling challenges across various datasets. When employing DIV2K as the training set and Set5 as the test, DSSTU-Net achieves a PSNR of 32.62, outperforming U-Net, DSU-Net, and STU-Net with improvements of 14.1%, 11.5%, and 4.3%, respectively. This trend is echoed in the LSDIR dataset where DSSTU-Net records a PSNR of 33.54, surpassing U-Net, DSU-Net, and STU-Net by 12.9%, 5.9%, and 4.7%. In the Set14, DSSTU-Net’s PSNR of 29.96 leads U-Net by 13.7% and STU-Net by 5.1%. In the LSDIR dataset, it further extends its lead, achieving a PSNR of 32.47, which is significantly higher than STU-Net’s 30.76 by 5.5%. The validation sets echo these results, DSSTU-Net scores 31.59 PSNR on DIV2K, outperforming U-Net by 10.6% and STU-Net by 4.5%. In the LSDIR validation dataset, its PSNR of 31.95 is superior to STU-Net’s 30.40 by 5.1%. These results underscore DSSTU-Net’s sophisticated architecture, which incorporates Swin Transformers and DS mechanisms, providing superior detail preservation and textural fidelity compared to its counterparts. However, the reduced margins of improvement as the scaling factor increases to ×4 also highlights the escalating challenges of super-resolution tasks at higher scales.
U-Net demonstrates the least effective performance across all scales and datasets, highlighting the need for more advanced architectural features and robust training mechanisms to address the complexities of modern SR challenges. U-Net’s underperformance on the DIV2K and LSDIR dataset underscores the importance of continuous improvements in both architectural and algorithmic strategies to advance SR technology.
Building upon U-Net, DSU-Net introduces moderate improvements but does not fully close the gap with more complex models. While it outperforms U-Net by leveraging enhanced feature extraction capabilities, DSU-Net offers only incremental improvements and falls short of reaching the high standards set by more sophisticated models.
STU-Net introduces robust enhancements that improve performance, though it still lags behind the top-performing DSSTU-Net. The comparison highlights the critical impact of additional layers and training enhancements integrated into DSSTU-Net, which are essential for achieving superior performance. This is particularly evident under the demanding conditions of the LSDIR dataset at higher scales. While STU-Net narrows the performance gap with DSSTU-Net at scale ×3, it becomes clear that both models benefit from advanced architectural elements. However, the deeper integration of sophisticated features in DSSTU-Net provides a distinct advantage.
DSSTU-Net demonstrating the substantial benefits of integrating Swin Transformer blocks and DS mechanisms. The model excels at higher scales, where precision in reconstructing high-frequency details is crucial. At scale ×2 on the DIV2K dataset, DSSTU-Net significantly outperforms even the enhanced capabilities of U-Net, capturing finer details and textures with remarkable clarity. This trend continues as the scale increases, solidifying DSSTU-Net’s dominance and highlighting the effectiveness of its advanced design in pushing the boundaries of image SR performance.
Fig. 3 illustrates the performance of four different models—U-Net, DSU-Net, STU-Net, and DSSTU-Net—across three test sets (Set5, Set14, and Val) at different scaling factors (×2, ×3, ×4) on the DIV2K and LSDIR datasets. The graph uses lines to represent each of the test sets, with blue for Set5, orange for Set14, and grey for Val. The PSNR values are plotted on the Y-axis, which helps in comparing the image quality enhancements provided by each model. The graph highlights the superior performance of DSSTU-Net, which consistently achieves higher PSNR values across all test sets and scaling factors, indicating its robustness and effectiveness in handling super-resolution tasks. Notably, DSSTU-Net shows significant improvements over the other models, especially in more challenging settings at higher scales. This visualization effectively captures the advancements DSSTU-Net brings in terms of detailed retention and image clarity, validating its architectural benefits over traditional methods like U-Net and its variants.
Figure 3: Comparative analysis of PSNR performance across models and scales on DIV2K and LSDIR datasets
Fig. 4 presents the SSIM performance of various super-resolution models—U-Net, DSU-Net, STU-Net, and DSSTU-Net—across three test sets, Set5, Set14, and Val, detailed at scaling factors ×2, ×3, and ×4 on the DIV2K and LSDIR datasets. The SSIM metric quantifies the visual similarity between the reconstructed images and their high-resolution counterparts, with higher values indicating closer resemblance. The chart uses three colors to differentiate the test sets, displaying a trend where DSSTU-Net consistently achieves the highest SSIM scores. This visualization underlines DSSTU-Net’s superior performance in maintaining structural integrity and detail across varying levels of image resolution, setting it apart from other models like U-Net, DSU-Net, and STU-Net which exhibit varying degrees of effectiveness across the scales and datasets.
Figure 4: Comparative analysis of SSIM performance across models and scales on DIV2K and LSDIR datasets
Fig. 5 presents a visual comparison of SR results (×2) produced by different models on an image from the DIV2K validation set (DIV2K_val_0896.PNG). The sequence of images from (b) to (e) illustrates the incremental improvements in image quality achieved by each model, along with the corresponding PSNR and SSIM values that quantify their performance.
Figure 5: The visual comparison of SR results (×2) produced by different models on an image from the DIV2K validation set (DIV2K_val_0896.PNG). (a) displays the original high-resolution image from the DIV2K dataset, which is used as a benchmark for the super-resolution models. (b) presents results from the U-Net model. (c) shows output from the DSU-Net. (d) from the STU-Net achieves a PSNR of 40.94 and an SSIM of 0.9623. (e) features the DSSTU-Net, achieving the best performance with a PSNR of 43.25 and an SSIM of 0.9672, indicating superior detail and texture accuracy close to the original image
Fig. 5a displays the original HR image from the DIV2K validation set, serving as a benchmark for comparing the SR outputs of the various models. This image provides detailed textures in the feathers and background, allowing for thorough assessment.
In Fig. 5b, the result from U-Net achieves a PSNR of 38.01 and an SSIM of 0.9474. While the image is reasonably clear, there is noticeable softness in the details, particularly around the feather edges in the zoomed-in segment. Finer textures appear somewhat blurred, indicating the need for more advanced processing to capture intricate details.
Fig. 5c shows the output from DSU-Net, which marks an improvement with a PSNR of 39.86 and an SSIM of 0.9571. The image is sharper than U-Net’s output, with better-defined feather and background textures. The edges are crisper, and there is less blurring, demonstrating the model’s enhanced ability to restore fine details.
The result from STU-Net in Fig. 5d further refines the image quality, achieving a PSNR of 40.94 and an SSIM of 0.9623. This model offers even greater clarity and detail recovery, particularly in the intricate textures of the feathers and background. The improvements in sharpness and texture definition are evident, making this model more effective at handling complex image content.
Finally, Fig. 5e presents the output from DSSTU-Net, the top performer, with a PSNR of 43.25 and an SSIM of 0.9672. This model produces the clearest and most detailed image. The background is smoother and less noisy, highlighting the model’s superior capability in preserving high-frequency details while minimizing artifacts.
Fig. 6 presents a visual comparison of SR results (×4) for an image from the LSDIR validation set (LSDIR_val_0000229.PNG), showcasing the performance of different models on a highly detailed natural scene.
Figure 6: The visual comparison of SR results (×4) for an image from the LSDIR validation set (LSDIR_val_0000229.PNG). (a) displays the original high-resolution image, used as a benchmark for the super-resolution models. (b) presents results from the U-Net model. (c) from the DSU-Net shows a slight improvement with PSNR and SSIM values of 33.58 and 0.8876, respectively. (d) features the STU-Net, which further enhances the image with a PSNR of 33.84 and an SSIM of 0.8912. (e) demonstrates the DSSTU-Net’s superior performance with a PSNR of 34.81 and an SSIM of 0.9053, closely approximating the original image’s detail and texture, providing the highest fidelity among the models
Fig. 6a features the original HR image, serving as the reference for assessing the SR quality of the subsequent models.
In Fig. 6b, the U-Net model produces an image with a PSNR of 31.06 and an SSIM of 0.8763. The result exhibits a noticeable loss of texture detail and sharpness, especially in the finer scales, which appear slightly blurred and lack the original image’s crispness.
Fig. 6c shows the output from DSU-Net, which improves upon U-Net’s rendering with a PSNR of 33.58 and an SSIM of 0.8876. This model better preserves texture, though some fine details remain less sharp than in the original image.
Fig. 6d presents the STU-Net model, which further enhances image quality, achieving a PSNR of 33.84 and an SSIM of 0.8912. This model’s output more closely approximates the original, with improved sharpness in texture details, showcasing a better balance between detail preservation and noise reduction.
Finally, Fig. 6e shows the DSSTU-Net model, which delivers the best results with a PSNR of 34.81 and an SSIM of 0.9053. This output closely matches the original image’s quality, displaying exceptional detail in the textures and intricate patterns. The image is sharper, clearer, and more vivid, highlighting DSSTU-Net’s effectiveness in reconstructing high-frequency details with minimal artifacts.
Overall, the progressive improvements from U-Net through DSSTU-Net illustrate significant advancements in the ability to enhance and restore finer details and textures in SR tasks, particularly in complex natural images like those in DIV2K and LSDIR datasets.
Table 4 provides a comprehensive comparison of state-of-the-art methods for image super-resolution across various scales, highlighting DSSTU-Net’s performance in comparison with other advanced models. Each model is evaluated on the Set5, Set14, and validation sets using PSNR and SSIM metrics.
While MUN [8] leverages a multi-level U-Net structure for enhancing feature extraction from low-resolution images, DSSTU-Net extends this concept by incorporating Swin Transformer blocks that offer a more nuanced approach to feature extraction and integration. MUN’s reliance on multi-scale residual blocks for multi-level feature extraction is innovative; however, DSSTU-Net’s approach allows for a more efficient and deeper feature extraction capability, which is evident from its superior performance metrics, especially in terms of PSNR and SSIM across various datasets.
SwinIR [9] establishes a robust baseline with its use of Swin Transformer layers for deep feature extraction, which DSSTU-Net also employs. However, DSSTU-Net advances this concept by combining these transformers with DS mechanisms, enhancing the model’s ability to reconstruct high-quality images from severely degraded inputs. While SwinIR has demonstrated substantial improvements in image quality, DSSTU-Net’s enhancements enable it to achieve even higher benchmarks, particularly in handling more complex upscaling tasks.
Focused on exploiting the frequency domain for image restoration, LKFN [10] introduces frequency-enhanced pixel attention mechanisms to capture long-range dependencies effectively. DSSTU-Net, while not specifically targeting frequency domain enhancements, integrates a comparable depth of feature processing through its transformer-based architecture, yielding results that often surpass those of LKFN in direct comparisons, particularly at higher scales of image upscaling.
MARDGAN [11] uses a generative adversarial network framework enhanced with multi-scale dual-attention mechanisms, aiming to blend detailed feature extraction with generative capabilities. DSSTU-Net, by contrast, focuses on a deterministic approach with its DS and transformer layers, ensuring consistent high-quality image output without relying on adversarial training, which can be unstable and unpredictable.
Huang et al. [12] using dictionary learning for super-resolution is innovated upon by incorporating deep learning methods to enhance performance. DSSTU-Net, however, bypasses the need for extensive dictionary sizes by directly learning an end-to-end mapping from low to high-resolution images using deep learning architectures, simplifying the model and reducing the overhead associated with dictionary maintenance and update.
SCNet [19] represents a notable advancement in super-resolution technology, proposing a lightweight model with fully convolutional layers that utilize fewer parameters, offering a novel shift-convolution (SC) layer that adapts stride and direction hyper-parameters to extend the receptive fields traditionally associated with normal convolution. In contrast, our DSSTU-Net extends the capabilities of traditional SR models by integrating Swin Transformer blocks with DS mechanisms. While SCNet focuses on parameter efficiency and adaptability in its architecture, allowing for scalability across various model sizes and potential integration with attention mechanisms, DSSTU-Net leverages the power of Swin Transformers to achieve deeper feature extraction and enhanced image reconstruction quality. This is particularly beneficial in handling complex upscaling tasks that require high fidelity in restored textures and details. DSSTU-Net provides a more in-depth feature extraction capability, which is crucial for restoring high-complexity image details that SCNet may not fully capture due to its emphasis on reducing parameter count. Both models offer scalability; however, DSSTU-Net’s integration with DS allows it to maintain high performance across various scales and conditions without compromising on the quality of the output.
SuRGe [20] introduces a novel approach to super-resolution by employing a fully-convolutional Generative Adversarial Network (GAN) architecture. The SuRGe model emphasizes the optimal combination of convolutional features at increasing depths through learnable convex weights, and employs advanced loss functions like Jensen-Shannon and Gromov-Wasserstein to refine the generation of high-resolution images from low-resolution inputs. In contrast, DSSTU-Net leverages Swin Transformer blocks integrated with DS mechanisms, focusing on a deterministic approach rather than generative adversarial methods. While SuRGe utilizes GANs to potentially enhance textural details through adversarial training, DSSTU-Net aims for consistency and stability in image quality enhancement without the risk of mode collapse associated with GANs. Unlike SuRGe, which uses complex loss functions to guide its generative process, DSSTU-Net employs a more straightforward loss landscape that ensures predictable performance and easier optimization.
Zhu et al. [21] detail a new method in the field of image super-resolution that integrates the advantages of both spatial and frequency domain processing through a novel architecture named Attention Retractable Frequency Fusion Transformer (ARFFT). This method addresses the limitations of previous Transformer-based models, which were restricted by their receptive fields due to the deployment of self-attention within non-overlapping windows. The ARFFT model stands out for its use of a spatial-frequency fusion block (SFFB) which significantly enhances the Transformer’s ability to extend its receptive field across the whole image, thereby improving the quality of super-resolution results. This model introduces a progressive training strategy that involves training on image patches of varying sizes, which aids in further refining the super-resolution performance across various benchmark datasets. Despite the promising advances, the paper’s methodology still faces the challenge common to Transformer-based approaches, which is the computational intensity associated with the processing of global interactions. Additionally, the model’s reliance on large amounts of training data to achieve high performance might limit its application in resource-constrained scenarios.
Smith [22] introduced a novel approach to single-image super-resolution in his thesis, emphasizing the challenges and limitations of current super-resolution techniques while also showcasing the strengths and potential of deep learning-based solutions. This is particularly evident in his comparative analysis of U-Net and its advanced iterations. Smith points out the limited capabilities of basic models like U-Net in effectively enhancing image quality to meet practical application needs, particularly in high-complexity scenarios such as those offered by the LSDIR dataset. He critically evaluates how these models struggle with the fine details necessary for high-quality image reconstruction, emphasizing the need for more sophisticated architectures. To address these shortcomings, Smith proposes the use of GANs and introduces a lightweight SR network that incorporates SwinIR as the generator, enhanced by a GAN framework with MobileViT as a lightweight discriminator. This combination, he argues, significantly improves the quality of image super-resolution. when set against Smith’s approach that utilizes GANs to address super-resolution, DSSTU-Net provides a more straightforward yet effective solution. While Smith’s method significantly enhances image quality by combining advanced GAN structures with SwinIR and MobileViT, it introduces complexities related to training stability and model tuning. DSSTU-Net, in contrast, achieves comparable or superior results without the need for adversarial training, thus simplifying the training process and reducing the potential for model instability.
In conclusion, while each of the compared methods has its strengths and has significantly pushed forward the boundaries of super-resolution technology, DSSTU-Net’s integration of Swin Transformers with DS mechanisms not only addresses but also surpasses many of the limitations faced by these methods. This positions DSSTU-Net as a leading solution in the field, particularly in scenarios where complex image details and textures need to be restored at high upscaling factors.
In conclusion, while each of the compared methods has its strengths and has significantly pushed forward the boundaries of super-resolution technology, DSSTU-Net’s integration of Swin Transformers with deep supervision mechanisms not only addresses but also surpasses many of the limitations faced by these methods. This positions DSSTU-Net as a leading solution in the field, particularly in scenarios where complex image details and textures need to be restored at high upscaling factors. However, the model’s computational intensity, primarily due to the deep Swin Transformer blocks, requires substantial GPU resources which may not be feasible for all application scenarios, particularly in real-time or on-device processing environments. Optimizations such as model pruning, quantization, or the development of more efficient transformer models could potentially mitigate these issues.
Furthermore, DSSTU-Net’s performance is heavily dependent on the availability of extensive, high-quality training datasets. This reliance poses challenges in environments where such datasets are limited or where data privacy concerns preclude the use of extensive personal data. Exploring techniques like few-shot learning, synthetic data augmentation, or unsupervised learning approaches could help reduce this dependency, making DSSTU-Net more adaptable to varied and constrained settings.
The DSSTU-Net introduces a groundbreaking approach to SR technology by integrating RSTB and deep supervision within a U-Net architecture. This model excels at reconstructing HR images from LR inputs, demonstrating superior performance on the DIV2K and LSDIR datasets. By leveraging advanced transformer technology and deep supervision techniques, DSSTU-Net achieved significant improvements in image detail and texture accuracy. The DSSTU-Net model not only enhances the quality of super-resolution outputs but also sets a reference method for future research in image restoration and related fields.
Acknowledgement: None.
Funding Statement: This work was supported in part by the National Natural Science Foundation of China (62263006), 2021 Director’s Fund of the Guangxi Key Laboratory for Automatic Detection Technology and Instruments (YQ21107), Guilin University of Electronic Technology Scientific Research Fund Project (UF24014Y), Innovation Project of Guangxi Graduate Education (YCSW2024336), Middle-aged and Young Teachers’ Basic Ability Promotion Project of Guangxi (2021KY0802).
Author Contributions: The authors confirm contribution to the paper as follows: data curation: Taiping Mo; Formal analysis: Qi Ma; Investigation: Qiumei Li; Methodology: Bonan Yu; Validation: Peng Sun. All authors reviewed the results and approved the final version of the manuscript.
Availability of Data and Materials: The data that support the findings of this study are available from the corresponding author, Peng Sun, upon reasonable request.
Ethics Approval: Not applicable.
Conflicts of Interest: The authors declare no conflicts of interest to report regarding the present study.
References
1. Yu M, Shi J, Xue C, Hao X, Yan G. A review of single image super-resolution reconstruction based on deep learning. Multimed Tools Appl. 2024;83(18):55921–62. doi:10.1007/s11042-023-17660-4. [Google Scholar] [CrossRef]
2. Yan H, Wang Z, Xu Z, Wang Z, Wu Z, Lyu R. Research on image super-resolution reconstruction mechanism based on convolutional neural network. In: Proceedings of the 2024 4th International Conference on Artificial Intelligence, Automation and High Performance Computing; 2024; New York, NY, USA: Association for Computing Machinery. p. 142–6. [Google Scholar]
3. Qiu D, Cheng Y, Wang X. Medical image super-resolution reconstruction algorithms based on deep learning: a survey. Comput Meth Prog Bio. 2023;8(238):107590. doi:10.1016/j.cmpb.2023.107590. [Google Scholar] [PubMed] [CrossRef]
4. Zhang W, Jia S, Zhu H. Single pixel imaging based on bicubic interpolation walsh transform matrix. IEEE Access. 2024;12:138575–81. doi:10.1109/ACCESS.2024.3465659. [Google Scholar] [CrossRef]
5. Shang S, Shan Z, Liu G, Wang L, Wang X, Zhang Z, et al. ResDiff: combining cnn and diffusion model for image super-resolution. Proc AAAI Conf Artif Intell. 2024;38(8):8975–83. doi:10.1609/aaai.v38i8.28746. [Google Scholar] [CrossRef]
6. Yu C, Hong L, Pan T, Li Y, Li T. ESTUGAN: enhanced swin transformer with U-Net discriminator for remote sensing image super-resolution. Electronics. 2023;12(20):4235. doi:10.3390/electronics12204235. [Google Scholar] [CrossRef]
7. Yu J, Gao X, Tao D, Li X, Zhang K. A unified learning framework for single image super-resolution. IEEE T Neur Net Lear. 2013;25(4):780–92. [Google Scholar]
8. Han N, Zhou L, Xie Z, Zheng J, Zhang L. Multi-level U-net network for image super-resolution reconstruction. Displays. 2022;73:102192. doi:10.1016/j.displa.2022.102192. [Google Scholar] [CrossRef]
9. Liang J, Cao J, Sun G, Zhang K, Van Gool L, Timofte R. SwinIR: image restoration using swin transformer. In: Proceedings of the IEEE/CVF International Conference on Computer Vision; 2021 Oct 11–17; Montreal, BC, Canada: IEEE. p. 1833–44. [Google Scholar]
10. Chen J, Duanmu C, Long H. Large kernel frequency-enhanced network for efficient single image super-resolution. In: Proceedings of the IEEE/CVF Conference on Computer Vision and Pattern Recognition; 2024 June 17–18; Seattle, WA, USA: IEEE. p. 6317–26. [Google Scholar]
11. Li HA, Wang D, Zhang J, Li Z, Ma T. Image super-resolution reconstruction based on multi-scale dual-attention. Connect Sci. 2023;35(1):2182487. doi:10.1080/09540091.2023.2182487. [Google Scholar] [CrossRef]
12. Huang Y, Bian W, Jie B, Zhu Z, Li W. Image super-resolution reconstruction based on deep dictionary learning and A+. Signal Image Video P. 2024;18(3):2629–41. doi:10.1007/s11760-023-02936-x. [Google Scholar] [CrossRef]
13. Ronneberger O, Fischer P, Brox T. U-net: convolutional networks for biomedical image segmentation. In: Medical Image Computing and Computer-Assisted Intervention-MICCAI 2015: 18th International Conference; 2015 Oct 5–9; Munich, Germany: Springer International Publishing. p. 234–41. [Google Scholar]
14. Liu Z, Lin Y, Cao Y, Hu H, Wei Y, Zhang Z, et al. Swin transformer: hierarchical vision transformer using shifted windows. In: Proceedings of the IEEE/CVF International Conference on Computer Vision; 2021; Montreal, QC, Canada. p. 10012–22. [Google Scholar]
15. Zhang L, Chen X, Zhang J, Dong R, Ma K. Contrastive deep supervision. In: European Conference on Computer Vision; 2022; Cham: Springer Nature Switzerland. p. 1–19. [Google Scholar]
16. Agustsson E, Timofte R, Ntire. Ntire 2017 challenge on single image super-resolution: dataset and study. In: Proceedings of the IEEE Conference on Computer Vision and Pattern Recognition Workshops; 2017; Honolulu, HI, USA. p. 126–35. [Google Scholar]
17. Li Y, Zhang K, Liang J, Cao J, Liu C, Gong R, et al. LSDIR: a large scale dataset for image restoration. In: Proceedings of the IEEE/CVF Conference on Computer Vision and Pattern Recognition; 2023; Vancouver, BC, Canada. p. 1775–87. [Google Scholar]
18. Hore A, Ziou D. Image quality metrics: PSNR vs. SSIM. In: 2010 20th International Conference on Pattern Recognition; 2010 Aug 23–26; Istanbul, Turkey: IEEE. p. 23–6. [Google Scholar]
19. Wu G, Jiang J, Jiang K, Liu X. Fully 1 × 1 convolutional network for lightweight image super-resolution. Mach Intell Res. 2024;8(21):1062–76. doi:10.1007/s11633-024-1501-9. [Google Scholar] [CrossRef]
20. Basu A, Bose K, Mullick SS, Chakrabarty A, Das S. Fortifying fully convolutional generative adversarial networks for image super-resolution using divergence measures. arXiv: 2404.06294. 2024. [Google Scholar]
21. Zhu Q, Li P, Li Q. Attention retractable frequency fusion transformer for image super resolution. In: Proceedings of the IEEE/CVF Conference on Computer Vision and Pattern Recognition; 2023 June 17–24; Vancouver, BC, Canada: IEEE. p. 1756–63. [Google Scholar]
22. Smith S. Deep Learning based single image super-resolution [Ph.D. dissertation]. Santa Clara, CA, USA: Santa Clara University; 2024. [Google Scholar]
Cite This Article
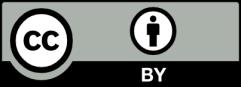
This work is licensed under a Creative Commons Attribution 4.0 International License , which permits unrestricted use, distribution, and reproduction in any medium, provided the original work is properly cited.